The role of data cleansing in a master data management strategy
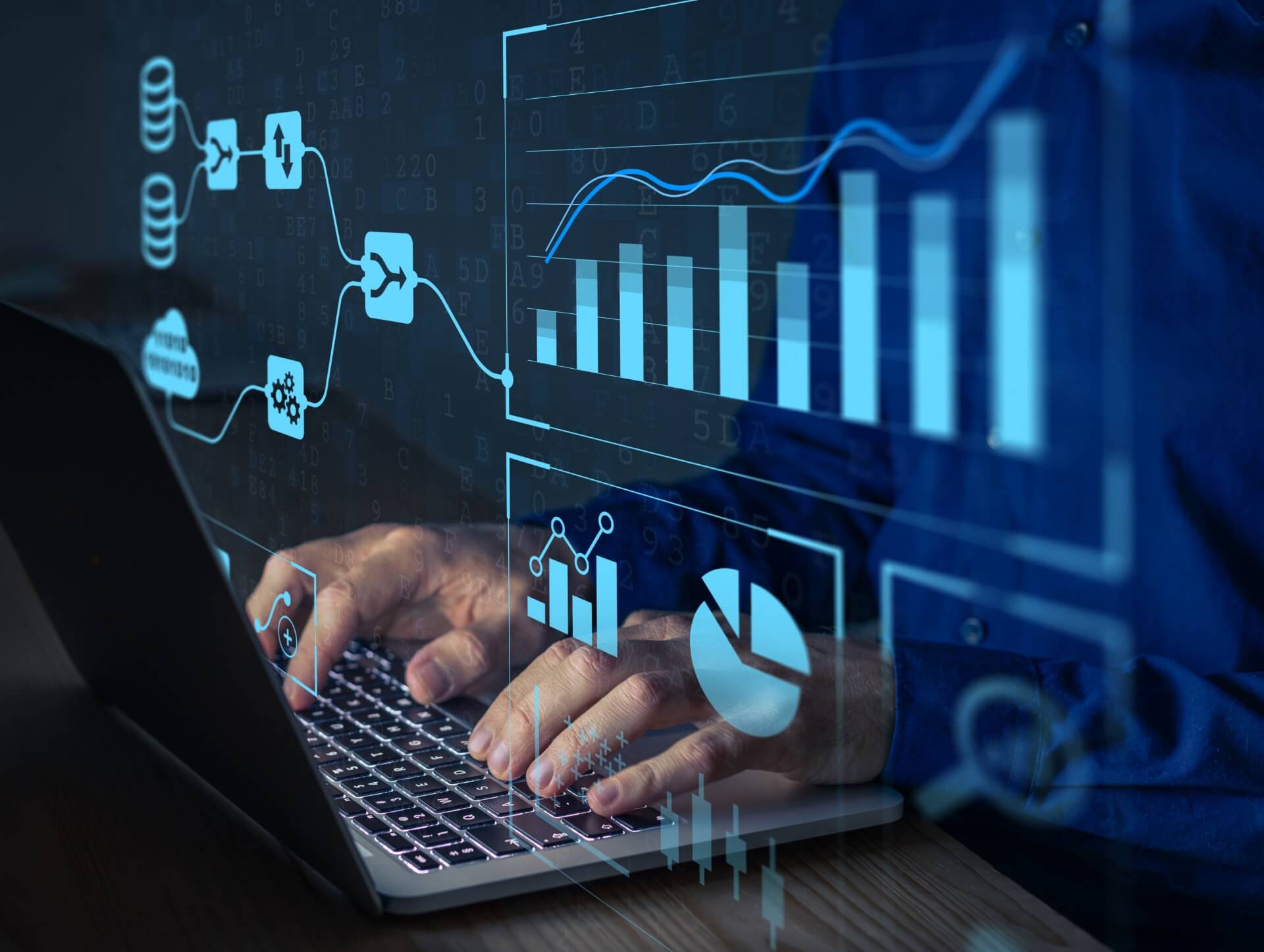
06/09/2023
Master Data Management (MDM) is a comprehensive approach to managing and integrating an organization's critical data assets. It involves the development and implementation of a set of processes, policies, and technologies to ensure data quality, consistency, and accuracy across various systems and applications.
One of the key aspects of an effective MDM strategy is data cleansing, which plays a vital role in ensuring the integrity and reliability of master data. In this article, we will explore the importance of data cleansing in MDM and how it contributes to the overall success of a master data management initiative.
The Need for Data Cleansing in MDM
Data cleansing, also known as data scrubbing or data cleansing, is the process of identifying and correcting or removing inaccurate, incomplete, or irrelevant data from a database. It involves various activities such as data validation, data standardization, data enrichment, and data de-duplication.
In the context of MDM, data cleansing is essential to ensure the quality and consistency of master data. Master data includes critical business information such as customer data, product data, supplier data, and employee data. This data is used across multiple systems and applications within an organization, and any inconsistencies or errors can have a significant impact on business operations and decision-making.
The Benefits of Data Cleansing in MDM
Implementing a robust data cleansing process as part of an MDM strategy can provide several benefits to an organization:
1. Improved Data Quality
Data cleansing helps improve the quality of master data by identifying and correcting errors, inconsistencies, and duplicates. This ensures that the data is accurate, reliable, and up-to-date, enabling better decision-making and more effective business operations.
2. Enhanced Data Consistency
Data cleansing ensures consistency across different data sources and systems by standardizing data formats, values, and codes. This helps eliminate data conflicts and discrepancies, providing a single, unified view of master data across the organization.
3. Increased Operational Efficiency
By eliminating duplicate and irrelevant data, data cleansing reduces data redundancy and storage requirements. This results in improved system performance, faster data retrieval, and reduced data processing costs.
4. Better Customer Experience
Data cleansing helps organizations maintain accurate and up-to-date customer information, enabling personalized and targeted marketing campaigns, improved customer service, and enhanced customer satisfaction.
Data Cleansing Techniques in MDM
Data cleansing in MDM involves various techniques and methodologies to ensure the accuracy and integrity of master data. Some of the commonly used techniques include:
1. Data Validation
Data validation involves checking the accuracy and integrity of data by comparing it against predefined rules and criteria. This helps identify and eliminate data errors, such as missing or invalid values, inconsistent formats, and incorrect data types.
2. Data Standardization
Data standardization involves transforming data into a consistent and uniform format. This includes standardizing data formats, values, and codes, as well as removing special characters, leading or trailing spaces, and unnecessary punctuation.
3. Data Enrichment
Data enrichment involves enhancing the quality and completeness of data by adding additional information from external sources. This can include enriching customer data with demographic information, product data with specifications, or employee data with job titles and qualifications.
4. Data De-duplication
Data de-duplication involves identifying and eliminating duplicate records from a database. This is done by comparing data fields and attributes to identify potential duplicates and then merging or removing them to ensure a single, unique record for each entity.
Challenges in Data Cleansing
While data cleansing is essential for ensuring data quality in MDM, it also presents several challenges that organizations need to overcome:
1. Data Volume
Managing large volumes of data can be a complex and time-consuming process. Data cleansing becomes challenging when dealing with massive data sets, as it requires significant computational resources and can impact system performance.
2. Data Complexity
Data comes in various formats and structures, making it challenging to standardize and cleanse. Complex data structures, such as hierarchical or nested data, require specialized techniques and tools to ensure accurate and consistent cleansing.
3. Data Integration
Data cleansing becomes more challenging when dealing with data from multiple sources and systems. Integrating data from different databases, applications, and external sources requires careful mapping and transformation to ensure consistency and accuracy.
4. Data Governance
Data cleansing requires a robust data governance framework to ensure that data quality standards and processes are followed consistently across the organization. This includes defining data ownership, establishing data stewardship roles, and implementing data quality monitoring and reporting.
Data Cleansing Best Practices
To ensure the success of data cleansing in MDM, organizations should follow these best practices:
1. Define Data Quality Metrics
Establish clear data quality metrics and standards to measure the effectiveness of data cleansing efforts. This includes defining criteria for accuracy, completeness, consistency, and timeliness of data.
2. Implement Data Profiling
Conduct data profiling to understand the quality and characteristics of the data. This involves analyzing data patterns, distributions, and anomalies to identify potential data quality issues and prioritize cleansing activities.
3. Involve Data Stewards
Assign dedicated data stewards who are responsible for overseeing the data cleansing process. Data stewards should have a deep understanding of the data and its business context and should collaborate with business users and IT teams to ensure data quality.
4. Automate Data Cleansing
Use automated tools and technologies to streamline and expedite the data cleansing process. This includes implementing data quality management software, data integration tools, and data validation frameworks to automate data cleansing tasks.
Conclusion
Data cleansing plays a critical role in a master data management strategy. It ensures the accuracy, consistency, and reliability of master data, enabling organizations to make informed decisions and improve business operations. By implementing robust data cleansing techniques and following best practices, organizations can unlock the full potential of their master data and drive business growth and success.
Contact us
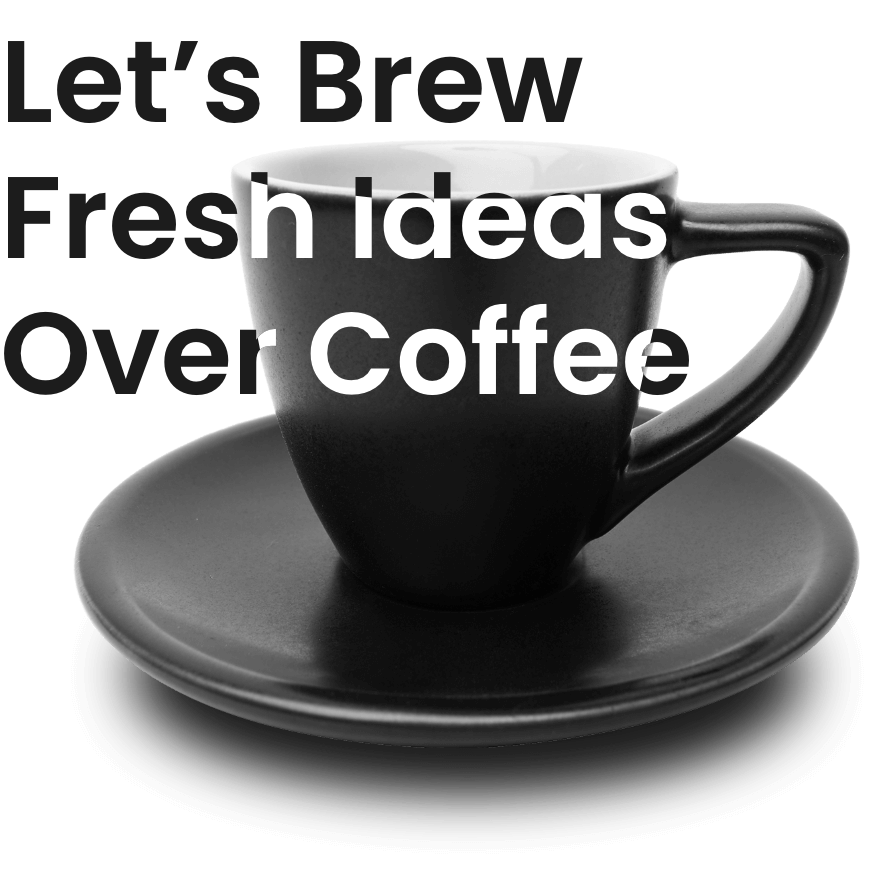
Spanning 8 cities worldwide and with partners in 100 more, we’re your local yet global agency.
Fancy a coffee, virtual or physical? It’s on us – let’s connect!