The role of data governance frameworks in master data governance
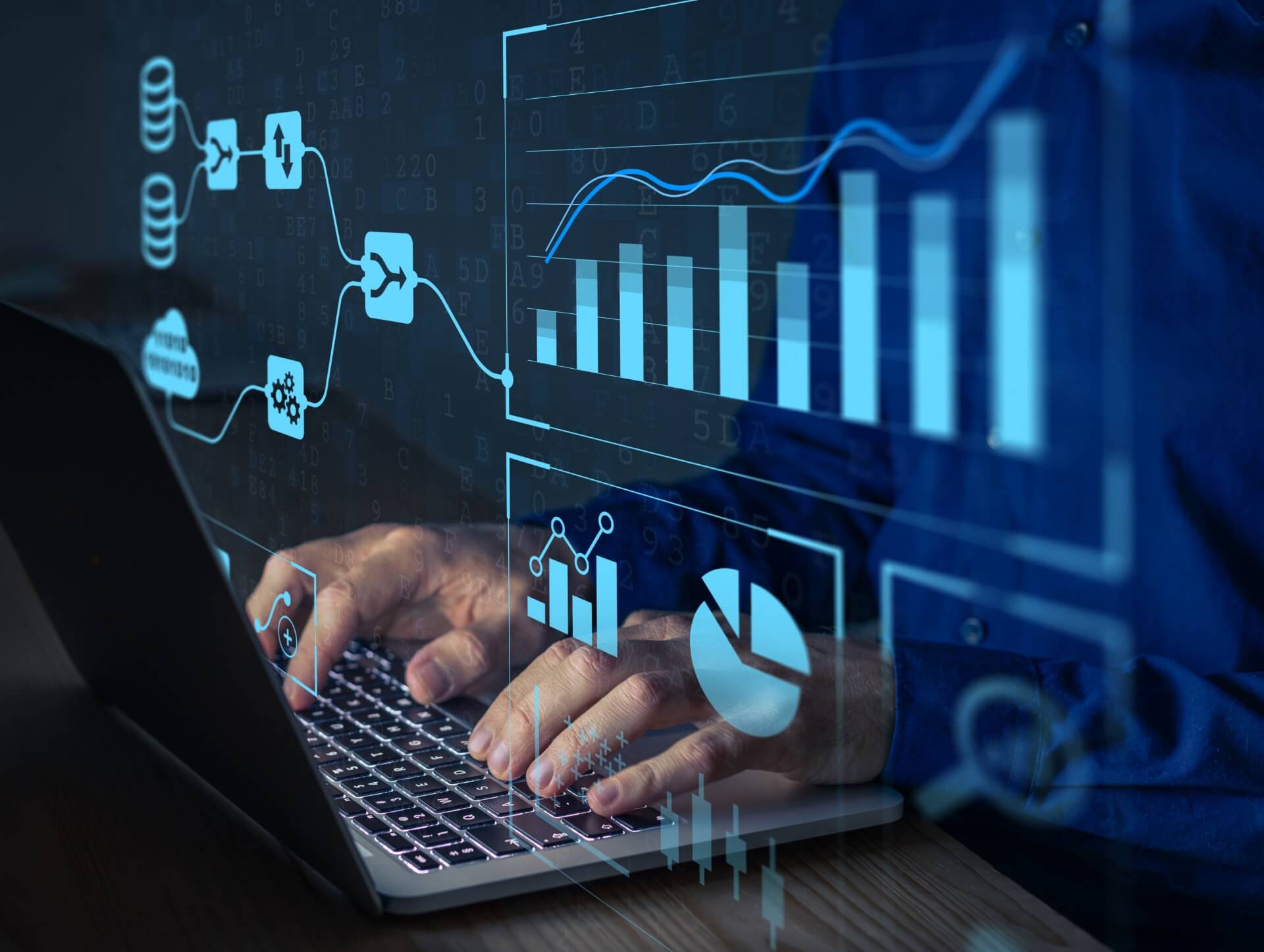
06/09/2023
Master Data Management (MDM) is a crucial discipline for organizations looking to effectively manage their data assets and drive business growth. It involves the processes, tools, and technologies used to create and maintain a single, accurate, and consistent view of an organization's critical data entities, such as customers, products, and suppliers. However, to ensure the success of MDM initiatives, organizations also need to establish robust data governance frameworks that provide guidelines, policies, and procedures for managing master data throughout its lifecycle.
The Importance of Data Governance in MDM
Data governance in MDM refers to the set of practices and processes that ensure the availability, usability, integrity, and security of master data. It encompasses the establishment of data stewardship roles, data quality management, data integration, metadata management, and compliance with data regulations. A well-defined data governance framework helps organizations achieve the following:
- Consistency: By defining data standards and enforcing them across the organization, data governance ensures consistent and reliable master data.
- Quality: Data governance frameworks facilitate data profiling, cleansing, and enrichment activities, leading to improved data quality.
- Compliance: Organizations operating in regulated industries need to comply with various data privacy and security regulations. Data governance frameworks help ensure compliance with these regulations.
- Efficiency: With clear policies and procedures in place, data governance frameworks streamline data management processes, reducing redundancy and improving efficiency.
- Trust: By establishing accountability and responsibility for data, data governance frameworks build trust in the accuracy and reliability of master data.
Best Practices for Data Governance in MDM
Implementing an effective data governance framework for MDM requires careful planning and adherence to best practices. Here are some key considerations:
1. Define Clear Roles and Responsibilities
Assigning clear roles and responsibilities is essential for effective data governance. Identify data stewards who will be responsible for managing and maintaining master data. Data stewards should have a deep understanding of the business and be empowered to make decisions regarding data quality, data integration, and metadata management.
2. Establish Data Standards and Policies
Data governance frameworks should define data standards and policies to ensure consistency and integrity of master data. This includes defining naming conventions, data formats, data validation rules, and data classification schemes. Regularly review and update these standards to adapt to changing business requirements and data regulations.
3. Implement Data Quality Management
Data quality management is a critical component of data governance in MDM. Implement data profiling, cleansing, and enrichment processes to improve the quality of master data. Use data quality tools and technologies to identify and resolve data issues, such as duplicates, inconsistencies, and inaccuracies.
4. Enable Data Integration
Data integration plays a vital role in MDM by consolidating data from various sources into a single, unified view. Data governance frameworks should include guidelines for data integration, such as data mapping, data transformation, and data synchronization. Implement data integration technologies and platforms to streamline the integration process.
The Role of Data Governance Frameworks in Master Data Governance
Data governance frameworks provide the structure and guidelines for managing master data throughout its lifecycle. They ensure that master data is accurate, consistent, and secure. Let's explore the specific roles of data governance frameworks in master data governance:
1. Lifecycle Management
Data governance frameworks define the processes and policies for managing master data throughout its lifecycle. This includes data creation, data maintenance, data archiving, and data retirement. By establishing clear guidelines for each stage of the lifecycle, organizations can ensure that master data remains relevant and up-to-date.
2. Metadata Management
Metadata, or data about data, is crucial for understanding and managing master data. Data governance frameworks include metadata management practices that capture and document metadata related to master data entities. This includes metadata about data sources, data transformations, data quality rules, and data lineage. Effective metadata management improves data discoverability, traceability, and understanding.
3. Automation and Orchestration
Data governance frameworks leverage automation and orchestration capabilities to streamline data management processes. Automation reduces manual effort and improves efficiency by automating repetitive tasks, such as data validation, data cleansing, and data integration. Orchestration ensures that data management processes are executed in a coordinated and consistent manner across the organization.
4. Security and Compliance
Data governance frameworks address data security and compliance requirements throughout the master data lifecycle. This includes implementing access controls, encryption, and data masking techniques to protect sensitive master data. Data governance frameworks also ensure compliance with data privacy regulations, such as GDPR and CCPA, by defining policies and procedures for data handling and consent management.
Challenges in Data Governance Frameworks for MDM
Implementing data governance frameworks for MDM comes with its own set of challenges. Here are some common challenges organizations face:
1. Organizational Alignment
Effective data governance requires strong organizational alignment and buy-in from stakeholders across the organization. Lack of alignment and support from business units can hinder the implementation and adoption of data governance frameworks.
2. Data Ownership
Determining data ownership and accountability can be challenging, especially in large organizations with complex data landscapes. Data governance frameworks should clearly define data ownership and establish processes for resolving data ownership disputes.
3. Data Quality Management
Data quality management is a continuous process that requires ongoing monitoring and improvement. Organizations often struggle with maintaining data quality standards and addressing data quality issues effectively.
4. Data Privacy and Security
With the increasing focus on data privacy and security, organizations need to ensure that data governance frameworks address these concerns. Implementing robust data privacy and security measures can be complex, considering the evolving regulatory landscape and the need to protect sensitive master data.
Conclusion
Data governance frameworks play a crucial role in ensuring the success of master data governance initiatives. By providing guidelines, policies, and procedures for managing master data, data governance frameworks enable organizations to achieve consistency, quality, compliance, efficiency, and trust in their data assets. However, implementing effective data governance frameworks for MDM comes with its own set of challenges. Organizations need to address these challenges and adhere to best practices to maximize the value and ROI of their data lifecycle management efforts.
Contact us
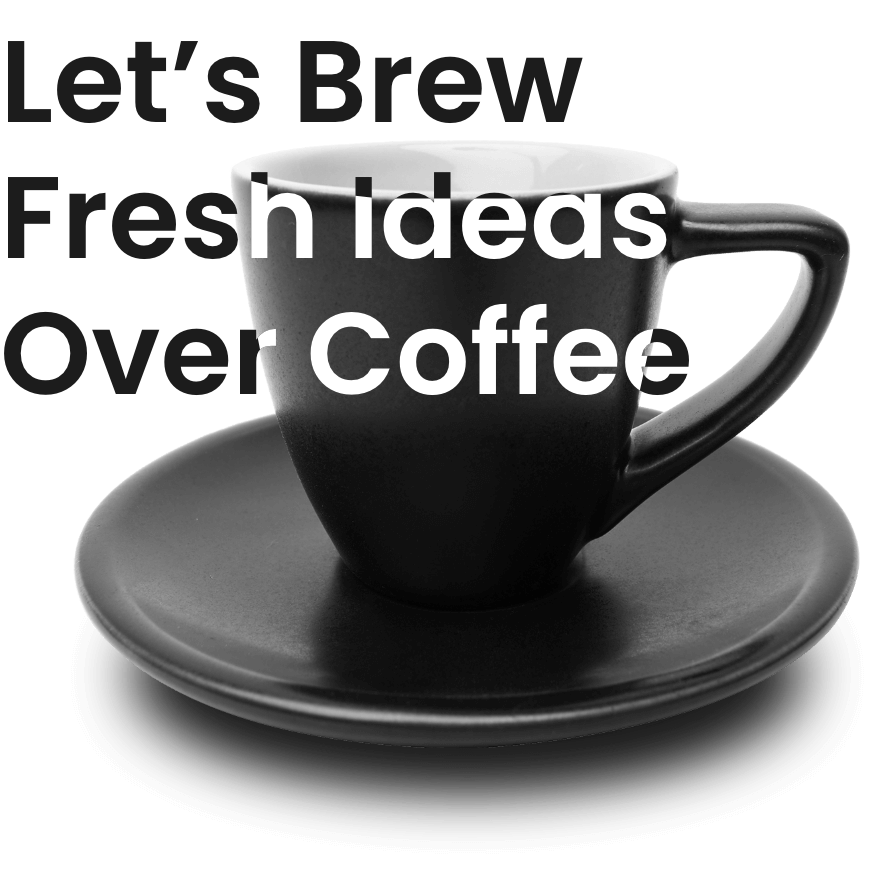
Spanning 8 cities worldwide and with partners in 100 more, we’re your local yet global agency.
Fancy a coffee, virtual or physical? It’s on us – let’s connect!