The role of data governance frameworks in MDM processes
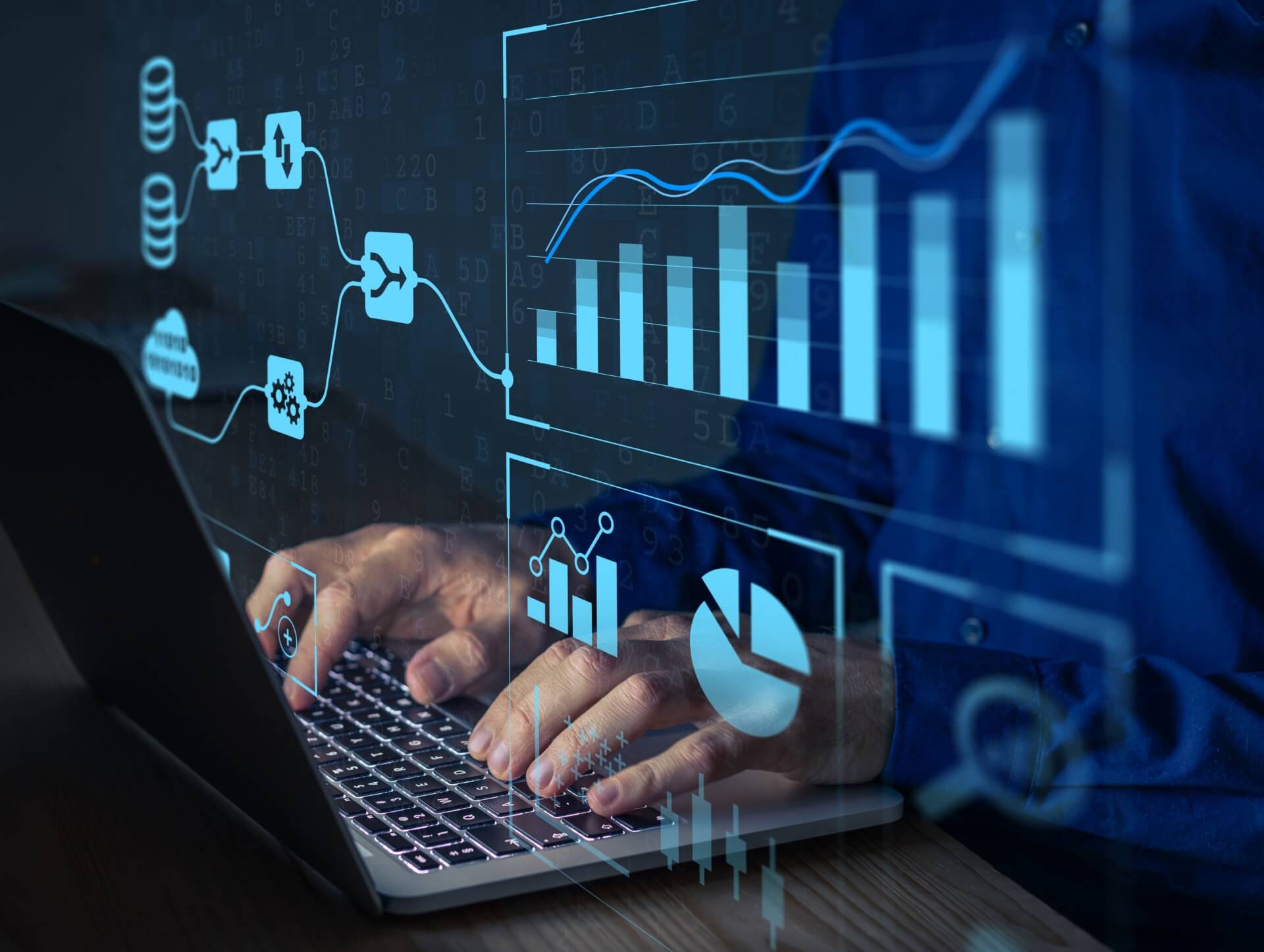
06/09/2023
Master Data Management (MDM) is a crucial process for businesses to ensure data consistency, accuracy, and integrity across various systems and applications. It involves creating and maintaining a centralized repository of master data that serves as the single source of truth for the organization.
However, managing master data effectively requires a robust data governance framework. In this article, we will explore the role of data governance frameworks in MDM processes and how they can benefit businesses.
Benefits of Data Governance in MDM
Data governance plays a critical role in the success of MDM initiatives. It provides a set of policies, processes, and procedures to ensure the quality, reliability, and security of master data throughout its lifecycle. Here are some key benefits of implementing a data governance framework in MDM:
- Data Consistency: A data governance framework helps establish standard definitions, formats, and rules for data elements, ensuring consistency across different systems and applications. This consistency enables accurate reporting, analysis, and decision-making.
- Data Quality Management: Data governance ensures that master data is accurate, complete, and up-to-date. It establishes data quality standards and monitors data quality metrics to identify and resolve data quality issues in a timely manner.
- Compliance and Risk Management: Data governance frameworks help organizations comply with regulatory requirements and industry standards. They establish controls and processes to mitigate data-related risks, such as data breaches or unauthorized access.
- Data Security: With the increasing risk of data breaches and cyber threats, data governance frameworks enforce security measures to protect master data from unauthorized access, ensuring data privacy and confidentiality.
- Improved Decision-Making: A well-governed MDM process provides accurate, reliable, and timely master data, enabling better decision-making at all levels of the organization. It ensures that decision-makers have access to trusted data for analysis and insights.
- Efficiency and Cost Reduction: Data governance frameworks streamline MDM processes, reducing data redundancy, duplication, and inconsistencies. This leads to improved operational efficiency, reduced costs, and better resource utilization.
- Business Agility: By establishing clear roles, responsibilities, and processes for managing master data, data governance frameworks enable organizations to adapt quickly to changing business requirements and market dynamics.
Data Governance Best Practices in MDM
Implementing a data governance framework requires careful planning and execution. Here are some best practices to consider:
- Executive Sponsorship: Obtain executive sponsorship and support for the data governance initiative. This ensures that the necessary resources, funding, and authority are allocated to the project.
- Clear Roles and Responsibilities: Define clear roles and responsibilities for data stewards, data owners, and other stakeholders involved in the data governance process. Ensure that everyone understands their responsibilities and is accountable for data quality and integrity.
- Data Governance Policies and Standards: Develop and document data governance policies, standards, and guidelines. These should cover data quality, metadata management, data security, privacy, and compliance with regulatory requirements.
- Data Governance Council: Establish a data governance council or steering committee comprising representatives from different business units and IT. This council should be responsible for setting data governance priorities, making decisions, and resolving conflicts.
- Data Stewardship: Appoint data stewards who are responsible for managing and maintaining master data. They should have the necessary knowledge, skills, and authority to enforce data governance policies and resolve data-related issues.
- Data Governance Tools and Technologies: Invest in appropriate data governance tools and technologies to support the implementation and ongoing management of the data governance framework. These tools should facilitate data profiling, data cleansing, metadata management, and data lineage tracking.
- Data Governance Training and Awareness: Provide training and awareness programs to educate employees about the importance of data governance and their roles in ensuring data quality and integrity. This helps create a data-driven culture within the organization.
- Continuous Monitoring and Improvement: Regularly monitor data quality, compliance, and adherence to data governance policies. Implement mechanisms for feedback, continuous improvement, and remediation of data issues.
Data Governance in MDM Lifecycle
Data governance is not a one-time activity but a continuous process that spans the entire lifecycle of master data. It encompasses the following stages:
- Requirements Gathering: Identify the key data elements and attributes required for MDM. Define the business rules, data quality metrics, and data governance policies for these data elements.
- Data Modeling: Design the master data model and establish relationships between different data entities. Ensure that the data model aligns with the organization's business processes and requirements.
- Data Integration: Integrate master data from various sources into the MDM platform. Implement data integration processes, such as data profiling, data cleansing, and data transformation, to ensure data quality and consistency.
- Data Governance and Stewardship: Enforce data governance policies and standards to ensure data quality, security, and compliance. Appoint data stewards who are responsible for managing and maintaining master data throughout its lifecycle.
- Data Access and Security: Establish access controls and security measures to protect master data from unauthorized access. Implement data privacy and confidentiality measures to comply with regulatory requirements.
- Data Lifecycle Management: Define processes and procedures for data lifecycle management, including data creation, modification, archival, and deletion. Implement metadata management practices to track and document changes to master data.
- Data Quality Management: Monitor and measure data quality metrics to identify and resolve data quality issues. Implement data quality improvement processes, such as data cleansing, deduplication, and enrichment, to maintain data integrity.
- Data Compliance: Ensure compliance with regulatory requirements, industry standards, and internal policies. Implement controls and processes to mitigate data-related risks and ensure data privacy and security.
- Data Analytics: Leverage master data for analytics and reporting purposes. Use data analytics tools and techniques to gain insights and make data-driven decisions.
- Continuous Improvement: Regularly review and refine data governance policies, processes, and procedures. Implement feedback mechanisms and continuous improvement initiatives to enhance the effectiveness of the data governance framework.
Challenges in Data Governance and MDM
Implementing a data governance framework in MDM is not without its challenges. Some common challenges include:
- Organizational Resistance: Resistance from employees who are resistant to change or do not understand the importance of data governance.
- Data Ownership: Lack of clear data ownership and accountability, resulting in data silos and duplication.
- Complex Data Landscape: Managing master data across multiple systems, applications, and data sources can be complex and challenging.
- Data Quality Issues: Poor data quality and data inconsistencies can undermine the effectiveness of the data governance framework.
- Resource Constraints: Limited resources, such as budget, skilled personnel, and technology, can hinder the implementation and maintenance of the data governance framework.
- Regulatory Compliance: Keeping up with evolving regulatory requirements and ensuring compliance can be a challenge.
- Cultural and Organizational Alignment: Aligning the data governance framework with the organization's culture, values, and business priorities can be difficult.
- Change Management: Managing change and ensuring user adoption of the data governance framework require effective change management strategies.
Conclusion
Data governance frameworks play a crucial role in ensuring the success of MDM processes. They provide the necessary policies, processes, and procedures to govern master data effectively throughout its lifecycle. By implementing a data governance framework, businesses can achieve data consistency, quality, security, and compliance, leading to improved decision-making, operational efficiency, and business agility. However, implementing and maintaining a data governance framework comes with its own challenges, which require careful planning, executive sponsorship, and continuous improvement. Overall, data governance is a critical component of a successful MDM strategy and should be prioritized by organizations seeking to leverage their data as a strategic asset.
Contact us
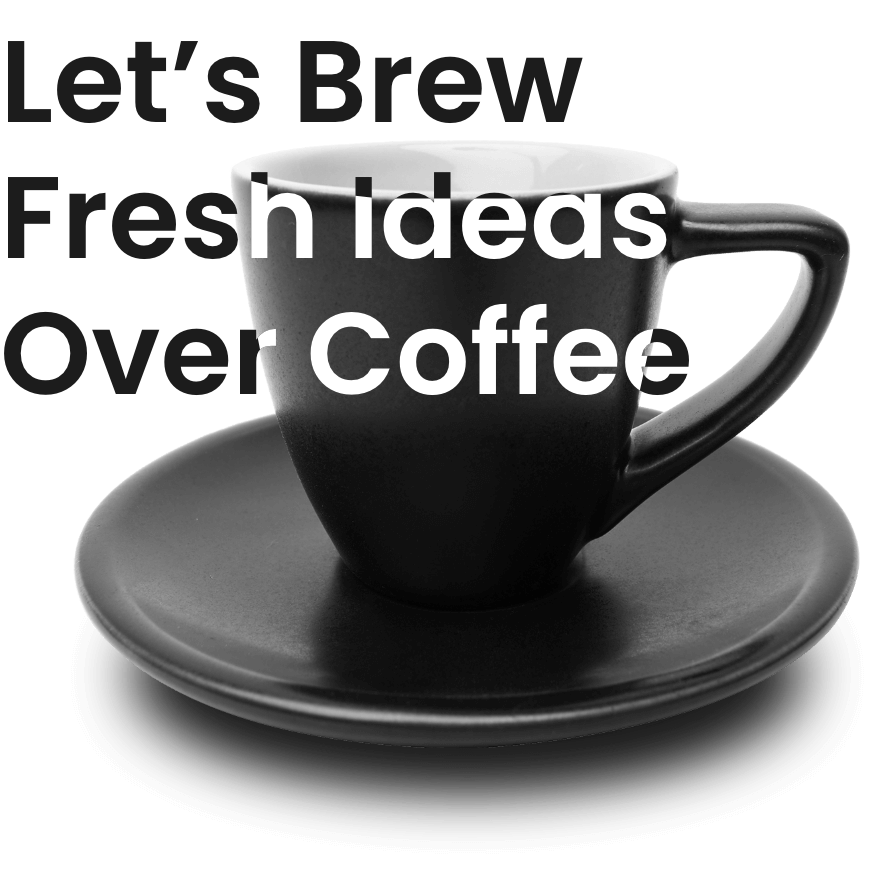
Spanning 8 cities worldwide and with partners in 100 more, we’re your local yet global agency.
Fancy a coffee, virtual or physical? It’s on us – let’s connect!