The role of data governance frameworks in multi-domain MDM implementation
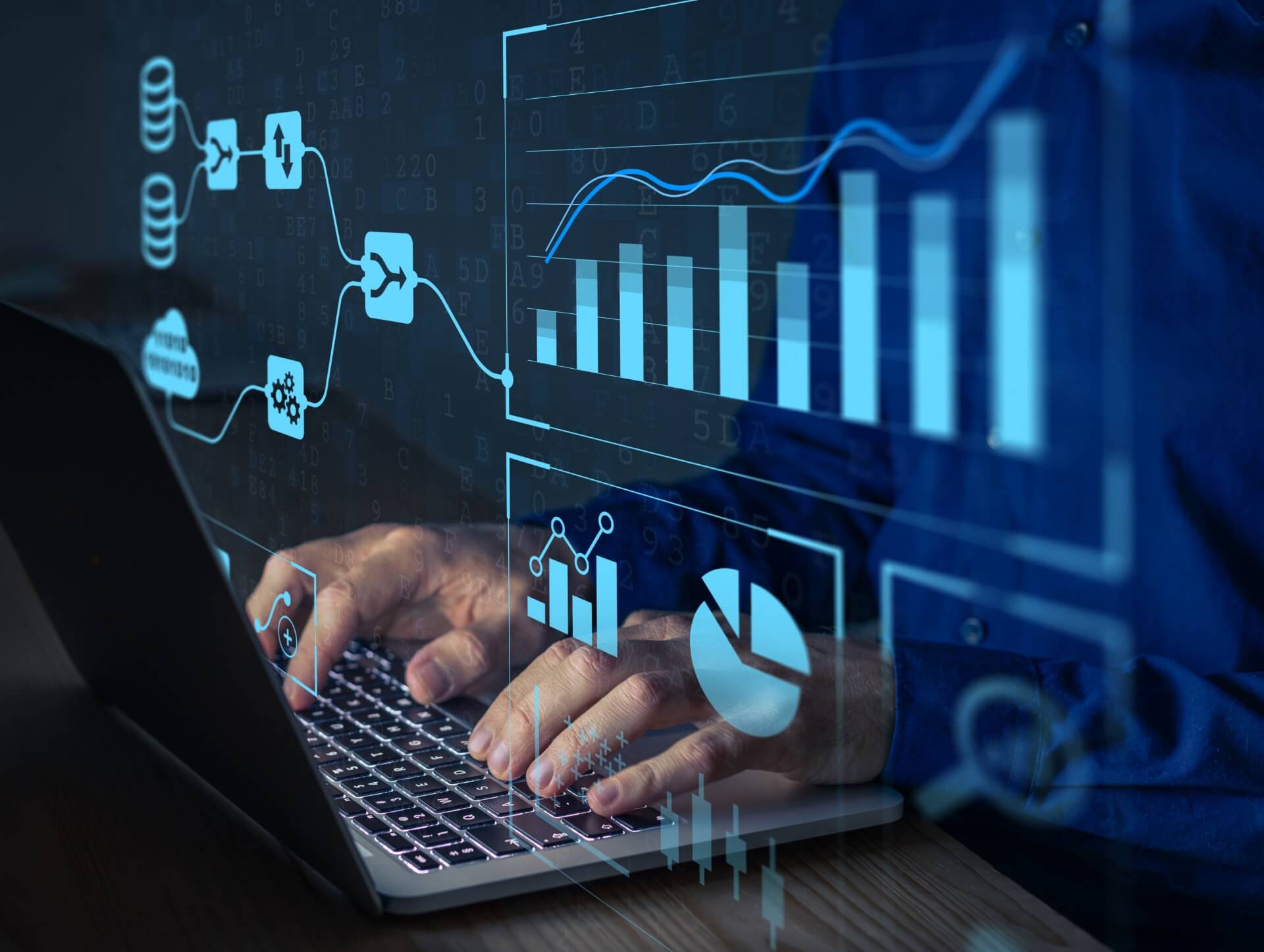
06/09/2023
Master Data Management (MDM) is a critical component of modern data management strategies. It involves the consolidation, cleansing, and governance of data to ensure a single, accurate, and complete view of master data entities such as customers, products, and locations. Implementing MDM solutions requires careful planning and adherence to best practices to achieve optimal results. One crucial aspect of successful MDM implementation is the establishment of robust data governance frameworks.
Data Governance in MDM
Data governance plays a pivotal role in MDM as it provides the necessary policies, processes, and frameworks to ensure the integrity, quality, and security of master data. In a multi-domain MDM implementation, where multiple business domains are involved, data governance becomes even more critical. It helps define the rules and guidelines for data management across different domains and ensures consistency and standardization of data across the organization.
Benefits of Data Governance in MDM
Implementing data governance frameworks in multi-domain MDM brings several benefits for organizations:
- Improved Data Quality: Data governance ensures that data is accurate, consistent, and up-to-date, leading to improved data quality. This, in turn, enhances decision-making and enables organizations to make informed business decisions.
- Enhanced Data Security: Data governance frameworks establish data security policies, access controls, and data privacy measures, ensuring that master data is protected from unauthorized access or breaches. This is particularly important considering the increasing concerns around data privacy and compliance.
- Increased Operational Efficiency: With data governance in place, organizations can streamline data management processes, eliminate data silos, and improve data integration. This leads to increased operational efficiency and productivity.
- Compliance with Regulations: Data governance frameworks help organizations comply with industry regulations and data protection laws. By defining data stewardship roles and responsibilities, organizations can ensure that master data is managed in accordance with legal and regulatory requirements.
- Better Decision-Making: Reliable and accurate master data, facilitated by data governance, enables better decision-making at all levels of the organization. It provides a single version of truth and eliminates the need for manual data reconciliation, saving time and effort.
Implementing Data Governance in MDM
Implementing data governance frameworks in multi-domain MDM requires careful planning and execution. Here are some best practices to consider:
Data Quality Management
Data quality management is a fundamental aspect of data governance in MDM. It involves the identification, assessment, and improvement of data quality throughout its lifecycle. Organizations should establish data quality metrics and define data quality rules to ensure that master data meets the required standards. Regular data profiling and cleansing activities should be conducted to address data quality issues.
MDM Strategy for Businesses
It is crucial for organizations to develop a clear MDM strategy that aligns with their business objectives. The strategy should define the scope, goals, and roadmap for MDM implementation and outline the roles and responsibilities of key stakeholders. This ensures that data governance frameworks are designed and implemented in line with the organization's overall MDM strategy.
MDM Implementation Process
The implementation process for data governance frameworks in multi-domain MDM involves several key steps:
- Assess Current State: Organizations should assess their current data management practices, identify gaps and areas of improvement, and define the desired future state of data governance.
- Define Data Governance Framework: Based on the desired future state, organizations should define the data governance framework, which includes data governance policies, processes, roles, and responsibilities.
- Establish Data Stewardship: Data stewardship is a critical component of data governance. Organizations should identify data stewards who will be responsible for managing and governing master data across different domains.
- Implement Data Governance Tools and Technologies: Organizations should leverage data governance tools and technologies to support the implementation of data governance frameworks. These tools help automate data governance processes and provide visibility into data quality and compliance.
- Monitor and Measure: Once the data governance frameworks are implemented, organizations should establish monitoring and measurement mechanisms to track the effectiveness of data governance practices. Regular audits and data quality assessments should be conducted to ensure ongoing compliance.
Challenges in Data Governance in MDM
Implementing data governance frameworks in multi-domain MDM is not without its challenges. Some common challenges include:
- Organizational Alignment: Getting buy-in from different business units and stakeholders can be challenging. It requires effective communication and collaboration to ensure that data governance frameworks are implemented consistently across the organization.
- Data Ownership: Identifying data owners and establishing clear lines of responsibility can be complex, especially in organizations with a decentralized data management structure. Data stewards play a crucial role in addressing this challenge.
- Legacy Systems and Data Integration: Integrating data from legacy systems and ensuring data consistency and quality can be a significant challenge. Organizations should invest in data integration technologies and establish robust data integration processes to overcome this challenge.
- Change Management: Implementing data governance frameworks requires changes in processes, roles, and responsibilities. Organizations should invest in change management activities to ensure smooth adoption and acceptance of data governance practices.
MDM Case Studies
Several organizations have successfully implemented data governance frameworks in multi-domain MDM. Here are some case studies:
- Company X: Company X implemented a comprehensive data governance framework to manage its customer master data. The framework included data quality management, data stewardship, and data security measures. As a result, the organization achieved a single, accurate view of customer data and improved customer experience.
- Company Y: Company Y, a multinational corporation, implemented data governance in its multi-domain MDM implementation. The organization established data governance policies, processes, and tools to ensure the consistency and integrity of master data across different business domains. This led to improved data quality and operational efficiency.
- Company Z: Company Z, a healthcare provider, implemented data governance in its MDM strategy to address data compliance and security requirements. The organization established data governance frameworks to ensure the privacy and security of patient data, leading to enhanced data security and compliance with industry regulations.
The Role of Data Governance in Lifecycle Management
Data governance is not limited to MDM but extends to data lifecycle management as well. Effective data governance ensures that master data is managed throughout its lifecycle, from creation to retirement. It involves:
Data Security throughout the Lifecycle
Data governance frameworks establish data security measures to protect master data at every stage of its lifecycle. This includes defining access controls, encryption mechanisms, and data privacy policies. By incorporating data security into the data lifecycle management process, organizations can mitigate the risk of data breaches and unauthorized access.
Data Compliance in Management
Data governance frameworks help organizations comply with data protection regulations and industry standards throughout the data lifecycle. By defining data governance policies and processes, organizations can ensure that master data is managed in accordance with legal and regulatory requirements. Regular audits and compliance assessments should be conducted to ensure ongoing compliance.
Master Data in Lifecycle Management
Master data is a critical component of the data lifecycle. Effective data governance ensures that master data is created, managed, and retired in a controlled and consistent manner. By establishing data governance frameworks, organizations can ensure the accuracy, consistency, and integrity of master data throughout its lifecycle.
Metadata Management in Data Lifecycle
Metadata management is an essential aspect of data governance in the data lifecycle. It involves the documentation, storage, and management of metadata, which provides context and meaning to the data. Metadata helps organizations understand the structure, relationships, and quality of master data. By incorporating metadata management into data governance frameworks, organizations can enhance data understanding and facilitate data integration.
Automation in Data Lifecycle
Data governance frameworks enable organizations to automate data management processes throughout the data lifecycle. Automation reduces manual effort, improves efficiency, and minimizes the risk of human error. By leveraging data governance tools and technologies, organizations can automate data quality checks, data integration processes, and data stewardship activities.
Cloud-Based Data Lifecycle
With the increasing adoption of cloud technology, organizations are leveraging cloud-based data lifecycle management solutions. These solutions provide scalability, flexibility, and cost-effectiveness. Data governance frameworks play a crucial role in ensuring the security, privacy, and compliance of master data in cloud environments. Organizations should establish data governance policies and controls specific to cloud-based data lifecycle management.
Analytics in Data Lifecycle
Data analytics is a growing field that provides valuable insights from data. Data governance frameworks enable organizations to leverage analytics throughout the data lifecycle. By incorporating analytics into data governance practices, organizations can gain a deeper understanding of master data, identify trends and patterns, and make data-driven decisions.
Challenges in Data Lifecycle Management
Implementing data governance in the data lifecycle management process comes with its own set of challenges:
- Data Volume and Variety: Managing large volumes of data from various sources can be challenging. Organizations should invest in data integration and data management technologies to handle diverse data types and formats.
- Data Privacy and Security: Ensuring data privacy and security throughout the data lifecycle is crucial. Organizations should establish data governance frameworks that include data security measures, access controls, and encryption mechanisms.
- Data Retention and Storage: Managing data retention and storage can be complex, especially considering data growth and compliance requirements. Organizations should establish data governance policies for data retention and storage and leverage data lifecycle management solutions to automate these processes.
- Data Governance Maturity: Achieving maturity in data governance is an ongoing process. Organizations should continuously assess and improve their data governance frameworks to meet evolving business needs and regulatory requirements.
Best Practices for Data Lifecycle Management
Implementing effective data governance in data lifecycle management requires adherence to best practices:
- Establish Clear Data Governance Policies: Organizations should define data governance policies that align with their business objectives and regulatory requirements. These policies should cover data security, data quality, data privacy, and compliance.
- Define Roles and Responsibilities: Clear roles and responsibilities should be defined for data stewardship, data ownership, and data governance. This ensures accountability and clarity in data management processes.
- Invest in Data Governance Tools and Technologies: Data governance tools and technologies help automate data management processes and provide visibility into data quality and compliance. Organizations should evaluate and invest in the right tools for their data governance needs.
- Establish Data Quality Metrics and Monitoring: Organizations should define data quality metrics and monitoring mechanisms to track and measure data quality throughout the data lifecycle. Regular data quality assessments and audits should be conducted.
- Continuously Improve Data Governance Frameworks: Data governance is an ongoing process. Organizations should continuously assess and improve their data governance frameworks to address emerging challenges and meet evolving business needs.
ROI of Effective Data Lifecycle
Implementing effective data governance in the data lifecycle management process brings several benefits and ROI for organizations:
- Cost Savings: Effective data lifecycle management reduces costs associated with data storage, data management, and data compliance. By automating data management processes, organizations can achieve significant cost savings.
- Improved Data Quality: Data governance ensures improved data quality, leading to better decision-making and reduced errors. This, in turn, improves operational efficiency and customer satisfaction.
- Enhanced Data Security and Compliance: Effective data governance frameworks ensure data security and compliance throughout the data lifecycle. This reduces the risk of data breaches and non-compliance penalties.
- Increased Productivity: Automation of data management processes improves productivity by reducing manual effort and minimizing the risk of human error. This allows employees to focus on more value-added tasks.
- Improved Customer Experience: With accurate and consistent master data, organizations can provide a better customer experience. This leads to increased customer satisfaction and loyalty.
Conclusion
Data governance frameworks play a crucial role in multi-domain MDM implementation and data lifecycle management. By establishing robust data governance frameworks, organizations can ensure the integrity, quality, and security of master data. This leads to improved data quality, enhanced data security, increased operational efficiency, and better decision-making. Implementing data governance in MDM and data lifecycle management comes with its own set of challenges, but by following best practices and leveraging the right tools and technologies, organizations can achieve significant ROI and value from their data management efforts.
Contact us
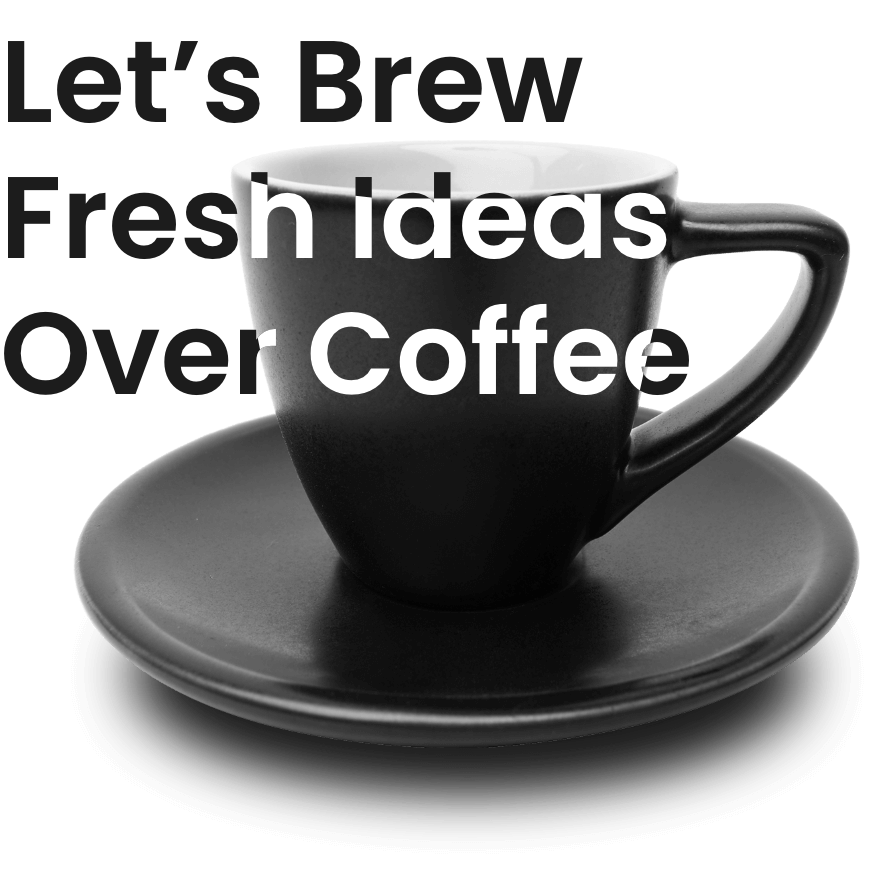
Spanning 8 cities worldwide and with partners in 100 more, we’re your local yet global agency.
Fancy a coffee, virtual or physical? It’s on us – let’s connect!