The role of data governance in ensuring data quality
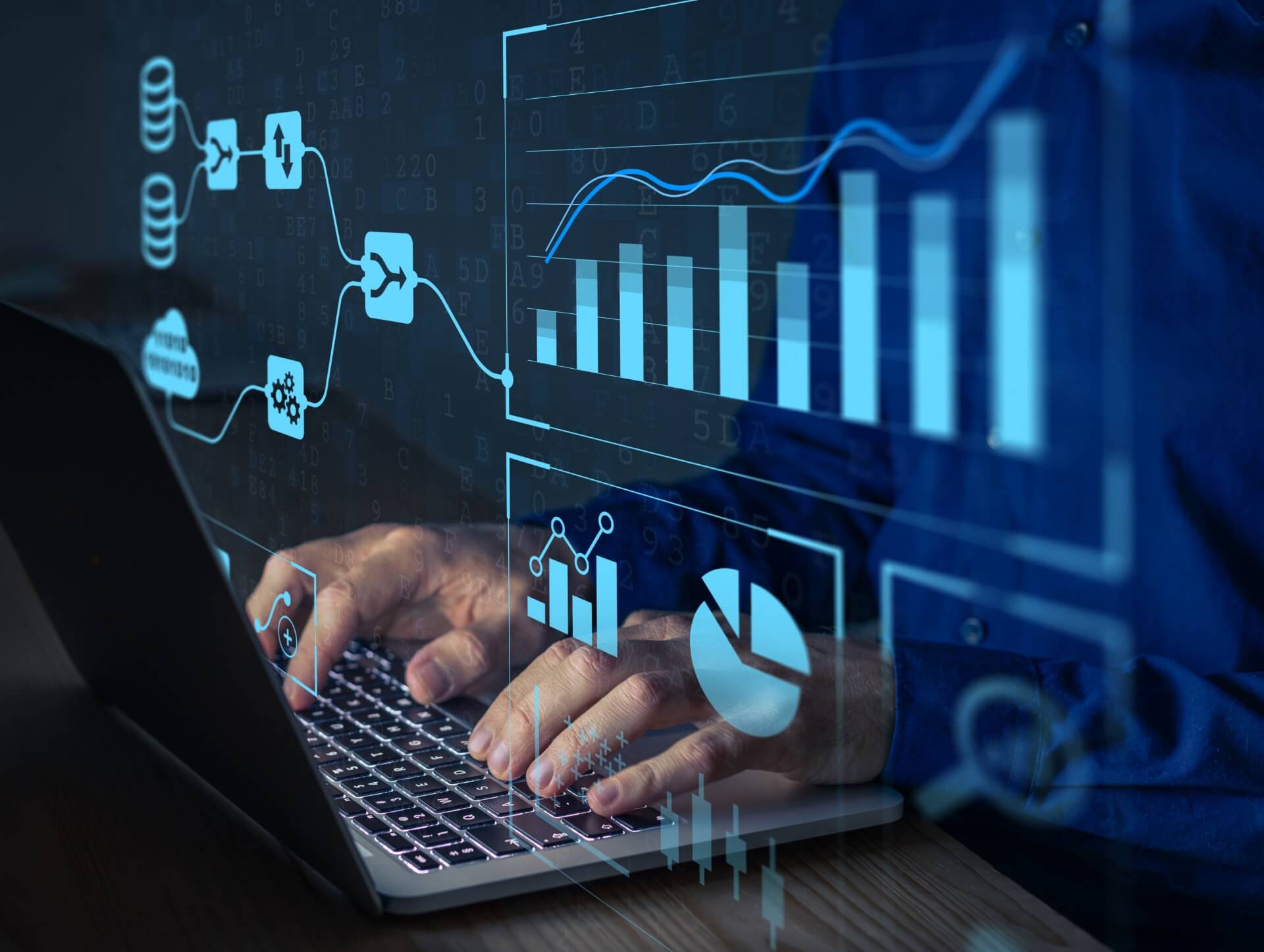
06/09/2023
Data has become a critical asset for businesses in the digital age. It drives decision-making, enables personalized customer experiences, and fuels innovation. However, the value of data is only as good as its quality. Poor data quality can lead to inaccurate insights, ineffective processes, and compromised customer experiences. This is where data governance comes in. Data governance is a strategic approach to managing and ensuring the quality, availability, integrity, and security of data within an organization. In this article, we will explore the role of data governance in ensuring data quality and its importance in today's data-driven world.
Data Governance in Master Data Management
Data governance plays a crucial role in the field of Master Data Management (MDM). MDM is a comprehensive approach to managing and harmonizing an organization's critical data, such as customer, product, and supplier data, to create a single trusted view of this data across the enterprise. Effective data governance is essential for the success of an MDM strategy. It provides the framework, processes, and policies to ensure the accuracy, consistency, and integrity of master data.
Benefits of Data Governance in MDM
Data governance in MDM brings a range of benefits to organizations. Let's explore some of the key advantages:
1. Improved Data Quality
Data governance ensures that data is accurate, complete, and consistent. It establishes data quality standards, defines data validation rules, and monitors data quality metrics. By implementing data governance practices, organizations can significantly improve the overall quality of their master data. This, in turn, leads to better decision-making, improved operational efficiency, and enhanced customer experiences.
2. Enhanced Data Security
Data governance helps organizations establish data security policies and controls to protect sensitive and confidential information. It ensures that access to data is granted based on defined roles and responsibilities, and that data is encrypted and stored securely. With data governance in place, organizations can mitigate the risk of data breaches and unauthorized access, safeguarding their valuable master data.
3. Increased Compliance
Data governance ensures that organizations comply with relevant data regulations and industry standards. It helps establish data privacy policies, data retention policies, and data classification frameworks. By adhering to these regulations and standards, organizations can avoid legal and financial penalties, maintain customer trust, and demonstrate their commitment to responsible data management.
4. Improved Decision-Making
Data governance provides a single version of truth for master data, ensuring that all stakeholders have access to accurate and consistent information. This enables better decision-making across the organization, as decision-makers can rely on trusted data for analysis and insights. With data governance, organizations can make more informed decisions, identify new opportunities, and respond quickly to market changes.
MDM Best Practices for Data Governance
Implementing data governance in MDM requires careful planning and adherence to best practices. Here are some key best practices to consider:
1. Establish Clear Roles and Responsibilities
Define clear roles and responsibilities for data stewards, data owners, and other stakeholders involved in data governance. Clearly communicate their responsibilities and empower them to make decisions regarding data quality, security, and compliance.
2. Define Data Governance Policies and Standards
Develop comprehensive data governance policies and standards that align with the organization's overall data strategy. These policies should cover data quality, data security, data privacy, data retention, and other relevant aspects of data management.
3. Implement Data Quality Management Processes
Establish data quality management processes to continuously monitor and improve the quality of master data. This includes data profiling, data cleansing, data validation, and data enrichment activities. Regularly assess data quality metrics and address any issues proactively.
4. Leverage MDM Tools and Technologies
Invest in robust MDM tools and technologies that support data governance capabilities. These tools can automate data governance processes, provide data lineage and traceability, and enable collaboration among data governance stakeholders.
Data Governance in Data Lifecycle Management
Data governance is not limited to MDM; it also plays a crucial role in data lifecycle management. Data lifecycle management encompasses the processes and strategies for managing data throughout its lifecycle, from creation to deletion. Let's explore the role of data governance in different stages of the data lifecycle.
1. Data Security Throughout the Lifecycle
Data governance ensures that appropriate security measures are in place at each stage of the data lifecycle. This includes data encryption, access controls, user authentication, and data masking. By implementing data governance practices, organizations can protect data from unauthorized access, minimize the risk of data breaches, and maintain data confidentiality.
2. Data Compliance in Management
Data governance helps organizations comply with data regulations and industry standards throughout the data lifecycle. It ensures that data privacy policies, data retention policies, and data disposal policies are defined and followed. By adhering to these requirements, organizations can avoid compliance violations and mitigate legal and financial risks.
3. Metadata Management in Data Lifecycle
Data governance focuses on managing metadata throughout the data lifecycle. Metadata provides context and meaning to data, making it easier to understand, interpret, and use. By implementing metadata management practices, organizations can improve data discoverability, enable data lineage and traceability, and enhance data governance processes.
4. Automation in Data Lifecycle
Data governance promotes automation in data lifecycle management processes. By automating data quality checks, data integration, data transformation, and other data management tasks, organizations can improve efficiency, reduce manual errors, and ensure consistency and accuracy of data across different systems and processes.
Challenges in Data Governance and Data Lifecycle Management
Implementing effective data governance and data lifecycle management practices comes with its own set of challenges. Let's explore some common challenges and how to address them:
1. Lack of Executive Support
Data governance and data lifecycle management initiatives require strong executive support and sponsorship. Lack of executive buy-in can hinder the adoption and success of these initiatives. To address this challenge, it is crucial to educate executives about the benefits of data governance and data lifecycle management and demonstrate the value these initiatives bring to the organization.
2. Siloed Data and Systems
Many organizations struggle with fragmented data and siloed systems, which can hinder effective data governance and data lifecycle management. To address this challenge, organizations should invest in data integration technologies and establish data integration processes to consolidate and harmonize data from different sources. This will create a unified view of data and enable better data governance and data lifecycle management.
3. Data Quality Issues
Data quality issues are common challenges in data governance and data lifecycle management. Poor data quality can lead to inaccurate insights, flawed decision-making, and inefficient processes. To address this challenge, organizations should implement data quality management processes, including data profiling, data cleansing, and data validation. Regularly monitor data quality metrics and take corrective actions to improve data quality.
4. Lack of Data Governance Policies and Processes
Many organizations struggle with the lack of well-defined data governance policies and processes. This can result in inconsistent data management practices and a lack of accountability. To address this challenge, organizations should develop comprehensive data governance frameworks, define data governance policies and standards, and establish data governance processes and workflows. This will ensure consistent and effective data governance throughout the organization.
Conclusion
Data governance plays a critical role in ensuring data quality and the success of Master Data Management (MDM) and data lifecycle management initiatives. It provides the framework, processes, and policies to ensure the accuracy, consistency, integrity, and security of data. By implementing data governance practices, organizations can improve data quality, enhance data security, ensure compliance, and enable better decision-making. However, implementing effective data governance and data lifecycle management practices comes with its own set of challenges. Organizations need to address these challenges by securing executive support, integrating siloed data and systems, addressing data quality issues, and establishing comprehensive data governance policies and processes. By doing so, organizations can unlock the full potential of their data and drive growth, innovation, and success in the digital age.
Contact us
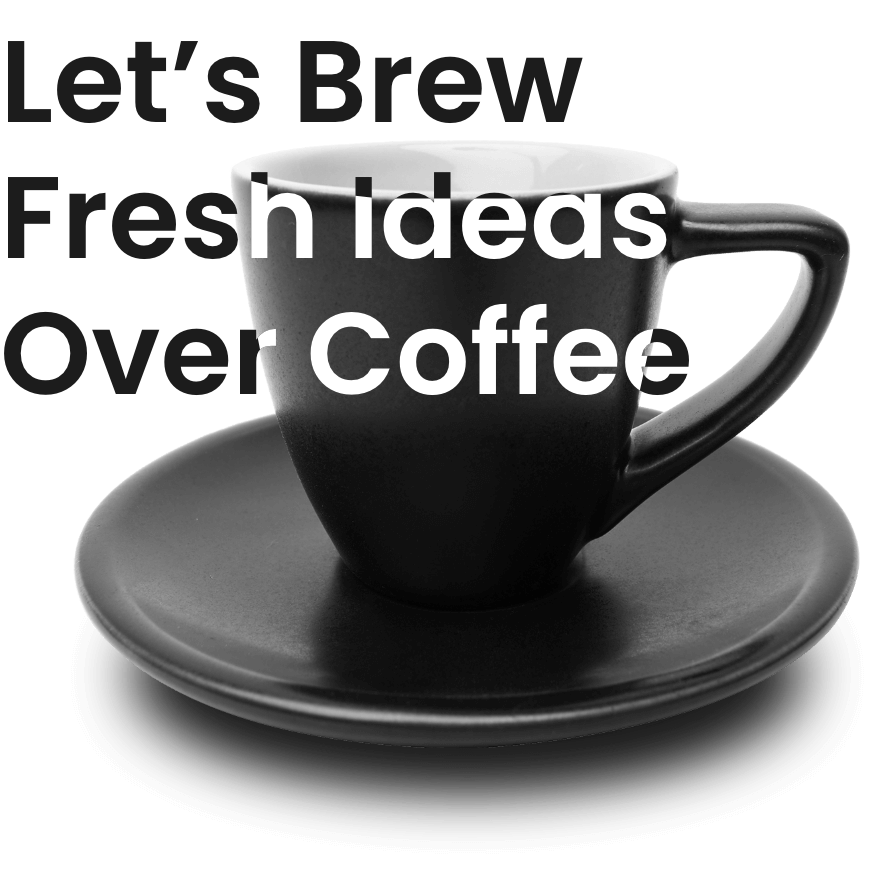
Spanning 8 cities worldwide and with partners in 100 more, we’re your local yet global agency.
Fancy a coffee, virtual or physical? It’s on us – let’s connect!