The role of data integration in achieving data quality in MDM
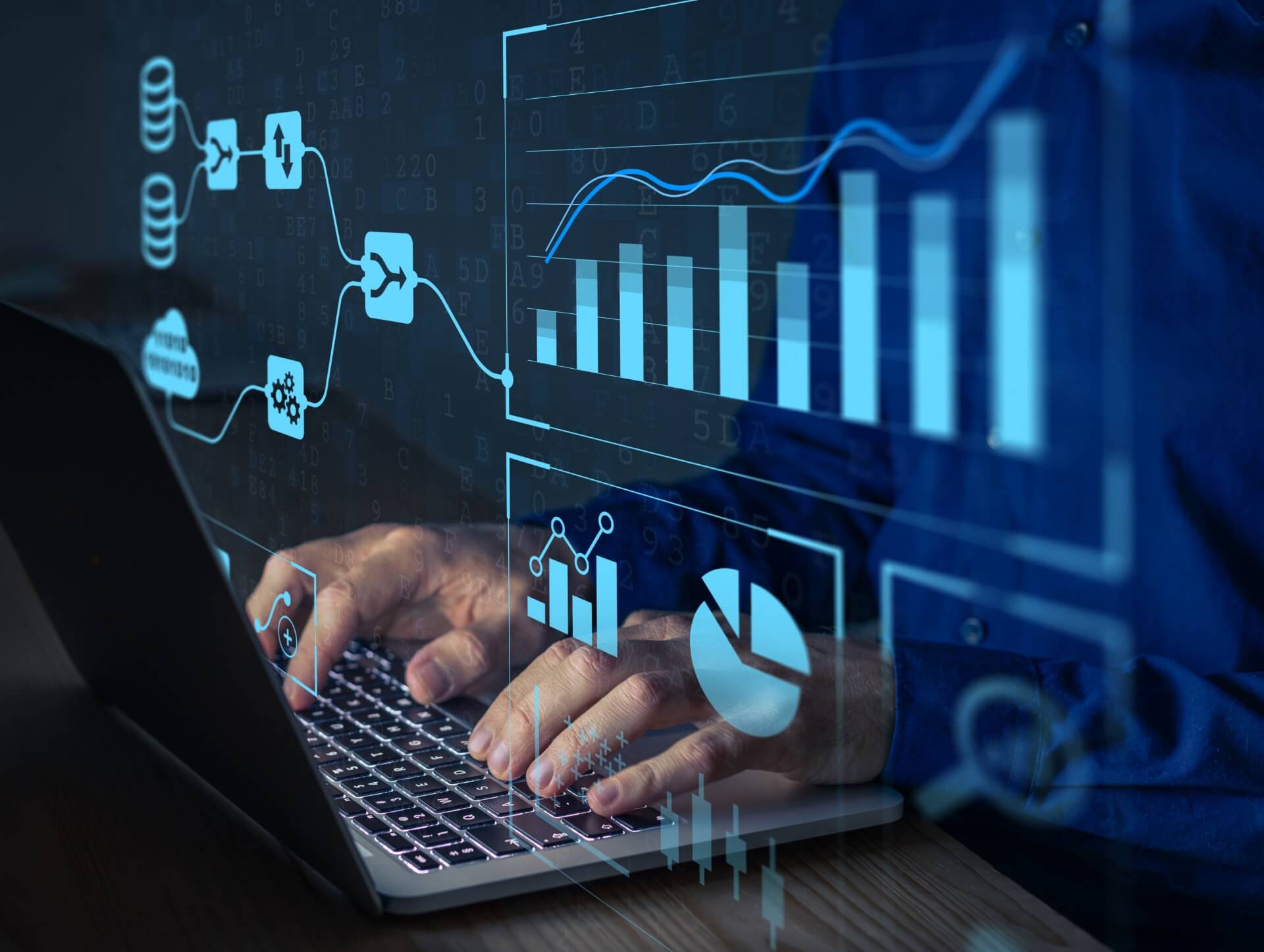
06/09/2023
Master Data Management (MDM) is a comprehensive approach to managing and integrating an organization's critical data across multiple systems and databases. It involves creating a single, reliable version of master data such as customer information, product details, and supplier data. By establishing a centralized and standardized source of data, MDM enables businesses to make informed decisions, improve operational efficiency, and enhance customer experience.
The Importance of Data Quality in MDM
Data quality is a crucial aspect of any successful MDM strategy. Poor data quality can lead to inaccurate insights, inefficient processes, and compromised decision-making. To achieve data quality in MDM, organizations need to ensure that the data they collect, store, and manage is accurate, complete, consistent, and up-to-date.
One of the key factors that contribute to data quality in MDM is data integration. Data integration involves combining data from various sources and systems into a unified view. It allows organizations to eliminate data silos, reduce duplication, and ensure data consistency. By integrating data from different systems, organizations can create a holistic and accurate view of their master data.
The Role of Data Integration in MDM
Data integration plays a critical role in achieving data quality in MDM. It enables organizations to:
1. Eliminate Data Silos
Data silos occur when different departments or systems within an organization store their data in separate databases or systems. This can lead to fragmented and inconsistent data. Data integration helps to break down these silos by merging data from various sources into a centralized repository. This allows organizations to have a single, unified view of their master data.
2. Reduce Data Duplication
Data duplication is a common problem in MDM. It occurs when the same data is stored in multiple systems or databases. This can lead to inconsistencies and confusion. Data integration helps to identify and eliminate duplicate data by merging similar records into a single, authoritative source. This not only improves data quality but also reduces storage costs and improves system performance.
3. Ensure Data Consistency
Data consistency is crucial for accurate decision-making and reporting. Inconsistent data can lead to conflicting information and unreliable insights. Data integration helps to ensure data consistency by mapping and transforming data from different sources into a standardized format. This ensures that all data adheres to the same rules and conventions, making it easier to analyze and interpret.
4. Improve Data Accuracy and Completeness
Data integration enables organizations to enhance the accuracy and completeness of their master data. By combining data from multiple sources, organizations can fill in any missing information and validate the accuracy of existing data. This helps to create a more reliable and comprehensive view of the master data, which can be used for various purposes such as analytics, reporting, and decision-making.
Data Integration Techniques in MDM
There are several techniques and approaches that organizations can use to integrate data in MDM:
1. Extract, Transform, Load (ETL)
ETL is a traditional approach to data integration that involves extracting data from various sources, transforming it into a standardized format, and loading it into a target system or database. ETL is commonly used in batch processing scenarios where data is collected and processed in regular intervals. It allows organizations to consolidate and synchronize data from multiple sources into a central repository.
2. Enterprise Service Bus (ESB)
An ESB is a middleware platform that facilitates the integration of various systems and applications. It acts as a central hub for data exchange and communication between different systems. ESBs enable organizations to establish real-time data integration by providing a set of standard interfaces and protocols for data exchange. This allows organizations to integrate data from different systems and databases in a more flexible and scalable manner.
3. Data Virtualization
Data virtualization is an approach to data integration that allows organizations to access and query data from multiple sources as if it were stored in a single database. It creates a virtual layer on top of the underlying data sources, enabling organizations to access and manipulate data without physically moving or replicating it. Data virtualization provides a unified view of data, eliminating the need for complex data integration processes.
4. Application Programming Interfaces (APIs)
APIs are a set of protocols and tools that enable different software applications to communicate and exchange data with each other. Organizations can use APIs to integrate data from various systems and databases by exposing data as services that can be accessed and consumed by other applications. APIs provide a standardized and secure way to integrate data, making it easier to build and maintain integrations between different systems.
Data Integration Best Practices in MDM
To ensure successful data integration in MDM and achieve high-quality data, organizations should follow these best practices:
1. Define Clear Data Integration Goals
Before embarking on a data integration project, organizations should clearly define their goals and objectives. This includes identifying the specific data sets that need to be integrated, the desired outcome, and the expected benefits. Defining clear goals helps organizations stay focused and ensures that the data integration project aligns with their overall MDM strategy.
2. Establish Data Governance Policies
Data governance is essential for maintaining data quality and integrity throughout the data integration process. Organizations should establish data governance policies and procedures that define data ownership, data stewardship, and data quality standards. This helps to ensure that data is properly managed, validated, and maintained throughout its lifecycle.
3. Implement Data Quality Management Tools
Data quality management tools can help organizations monitor and improve the quality of their master data. These tools provide functionalities such as data profiling, data cleansing, and data enrichment. By implementing data quality management tools, organizations can identify and resolve data quality issues, ensuring that the integrated data is accurate, complete, and consistent.
4. Perform Regular Data Audits
Regular data audits are essential to ensure the ongoing quality and integrity of integrated data. Organizations should perform periodic data audits to identify any data inconsistencies, errors, or gaps. This helps to identify and address data issues before they impact business operations or decision-making.
Conclusion
Data integration plays a critical role in achieving data quality in MDM. By eliminating data silos, reducing duplication, ensuring data consistency, and improving data accuracy and completeness, organizations can create a single, reliable source of master data. This enables businesses to make better decisions, improve operational efficiency, and enhance customer experience. By following best practices and implementing the right tools and technologies, organizations can successfully integrate data and achieve high-quality data in their MDM initiatives.
Contact us
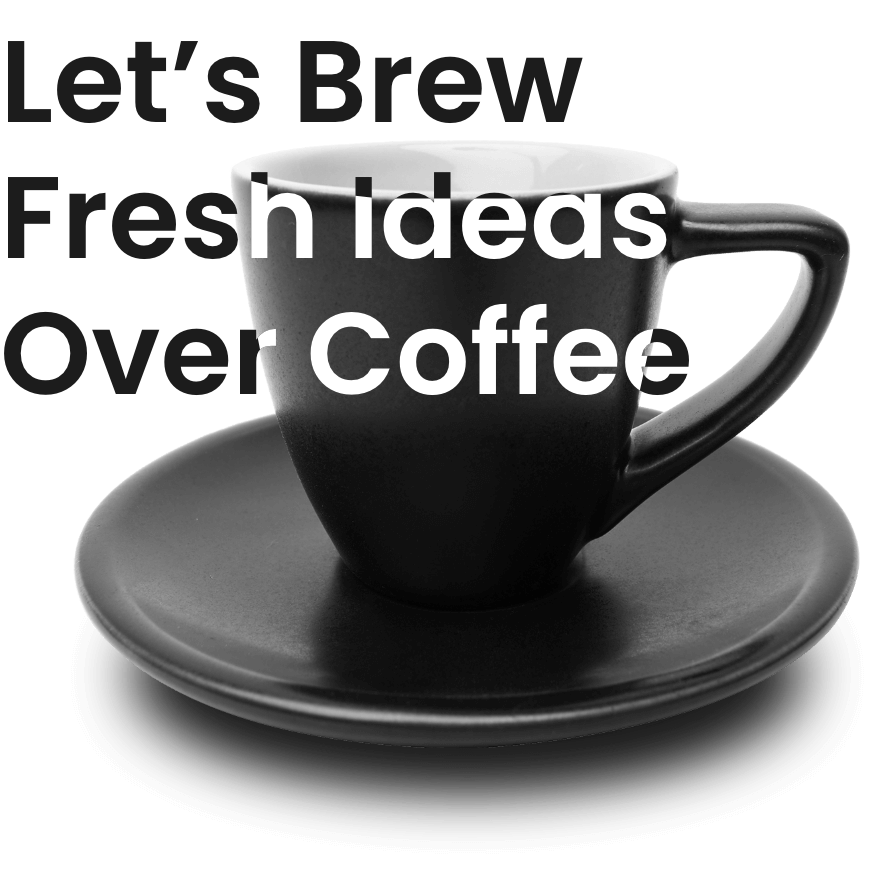
Spanning 8 cities worldwide and with partners in 100 more, we’re your local yet global agency.
Fancy a coffee, virtual or physical? It’s on us – let’s connect!