The role of data modeling in MDM implementation
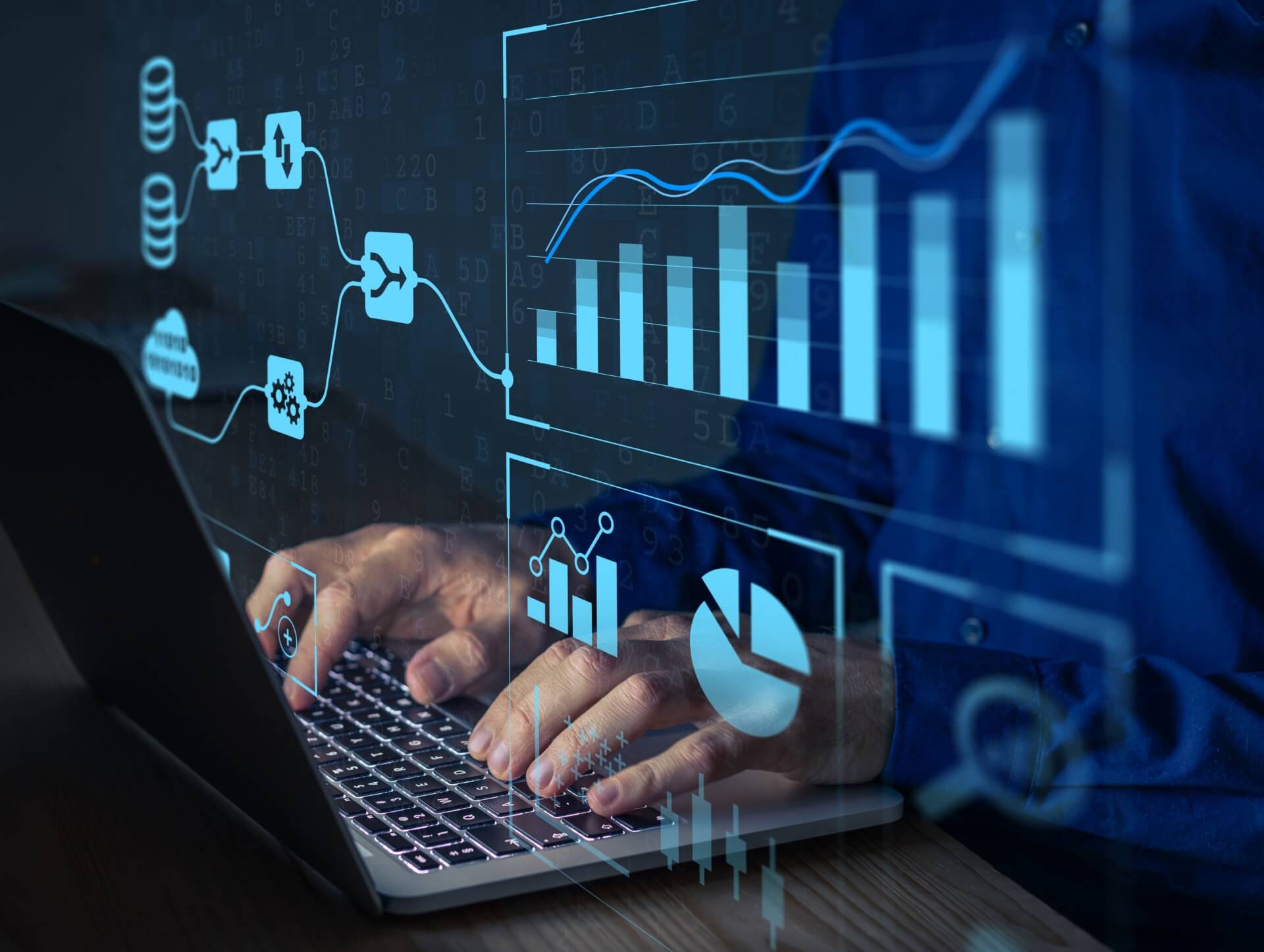
06/09/2023
Master Data Management (MDM) is a crucial aspect of modern businesses that deal with vast amounts of data. It involves the consolidation, cleansing, and management of master data to ensure data accuracy, consistency, and reliability across the organization. One of the key components of MDM is data modeling, which plays a vital role in implementing MDM solutions effectively.
The Importance of Data Modeling in MDM
Data modeling is the process of creating a conceptual representation of data entities, their attributes, and the relationships between them. It provides a structured approach to designing the data architecture and ensures that the data is organized, standardized, and easily accessible. In the context of MDM, data modeling plays a crucial role in several aspects:
1. Defining the Data Model: Data modeling helps in defining the data model for MDM, which serves as the foundation for the entire MDM implementation. The data model captures the structure, attributes, and relationships of the master data entities, such as customers, products, and suppliers. It provides a clear understanding of how the data is organized and enables efficient data management.
2. Data Integration: Data modeling helps in integrating data from various sources into a unified view. It identifies the common data elements and establishes the relationships between them. By mapping the data elements to the data model, organizations can ensure that the data is consistent and accurate across different systems and applications. This is particularly important in MDM, where data integration is a critical aspect of managing master data effectively.
3. Data Governance: Data modeling is closely linked to data governance in MDM. It helps in defining the data governance policies, rules, and standards that govern the creation, maintenance, and usage of master data. By incorporating data governance principles into the data model, organizations can ensure that the master data is managed in a controlled and compliant manner.
4. Data Quality Management: Data modeling is essential for data quality management in MDM. It enables organizations to define data quality rules and metrics that can be used to measure and improve the quality of master data. By incorporating data quality requirements into the data model, organizations can ensure that the master data is accurate, complete, and consistent.
Best Practices for Data Modeling in MDM
Implementing effective data modeling in MDM requires following best practices to ensure the success of the MDM implementation:
1. Engage Business Stakeholders: Involve business stakeholders throughout the data modeling process to ensure that the data model aligns with the business requirements and objectives. This will help in creating a data model that accurately represents the business entities, attributes, and relationships.
2. Follow Industry Standards: Adhere to industry-standard data modeling techniques and conventions to ensure that the data model is easily understandable and maintainable. This will enable effective collaboration among data modelers, developers, and business users.
3. Consider Future Scalability: Design the data model in a way that allows for future scalability and flexibility. Anticipate future data requirements and changes in the business environment to ensure that the data model can accommodate them without significant modifications.
4. Validate and Iterate: Validate the data model by conducting thorough reviews and testing. Iterate on the data model based on feedback and make necessary adjustments to ensure its accuracy and completeness.
Challenges in Data Modeling for MDM
While data modeling is essential for successful MDM implementation, there are several challenges that organizations may face:
1. Data Complexity: The complexity of the data and its relationships can pose challenges in data modeling for MDM. Organizations often deal with large volumes of data, multiple data sources, and complex data structures. It is important to carefully analyze and model the data to ensure its accuracy and consistency.
2. Data Governance: Incorporating data governance principles into the data model can be challenging. It requires aligning the data model with the data governance policies, rules, and standards. Organizations need to establish clear roles and responsibilities for data governance and ensure that the data model supports the governance requirements.
3. Data Integration: Integrating data from different sources can be complex, especially when dealing with heterogeneous systems and diverse data formats. Data modeling plays a crucial role in mapping and transforming the data to ensure its compatibility and consistency.
4. Data Quality: Ensuring data quality is a significant challenge in MDM. Data modeling needs to incorporate data quality rules and metrics to measure and improve the quality of master data. It requires continuous monitoring and refinement of the data model to maintain data quality.
Conclusion
Data modeling plays a crucial role in the successful implementation of MDM solutions. It helps in defining the data model, integrating data, ensuring data governance, and managing data quality. By following best practices and addressing the challenges, organizations can leverage data modeling to achieve accurate, consistent, and reliable master data, which is essential for making informed business decisions and driving organizational growth.
Contact us
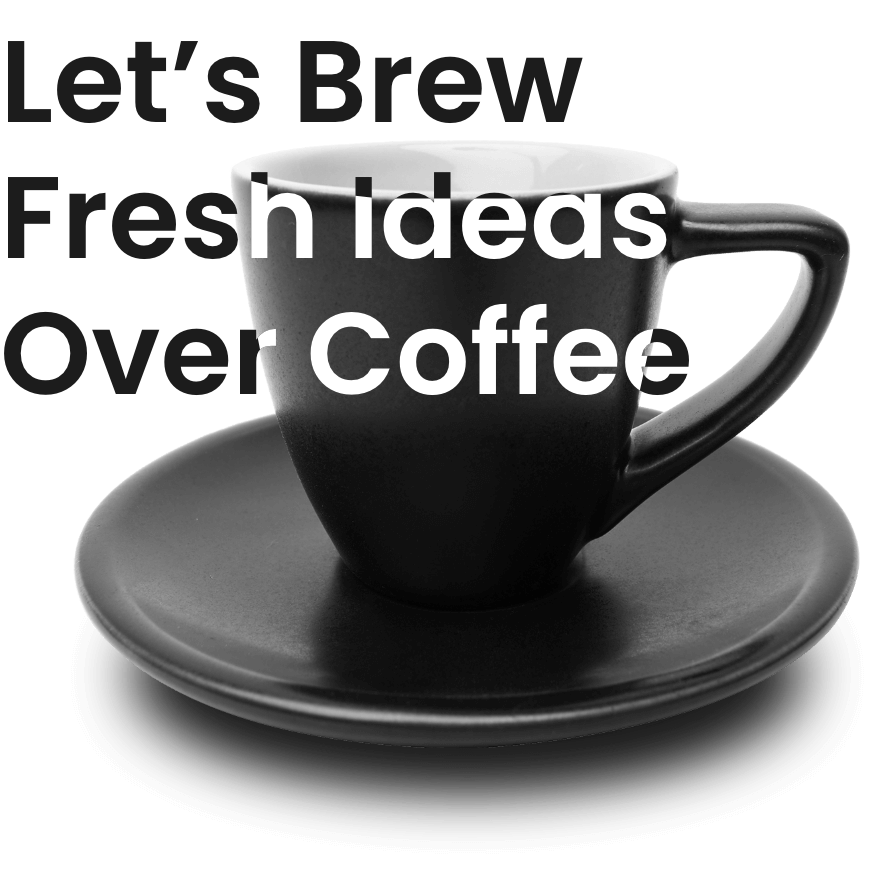
Spanning 8 cities worldwide and with partners in 100 more, we’re your local yet global agency.
Fancy a coffee, virtual or physical? It’s on us – let’s connect!