The role of data modeling techniques in MDM implementation
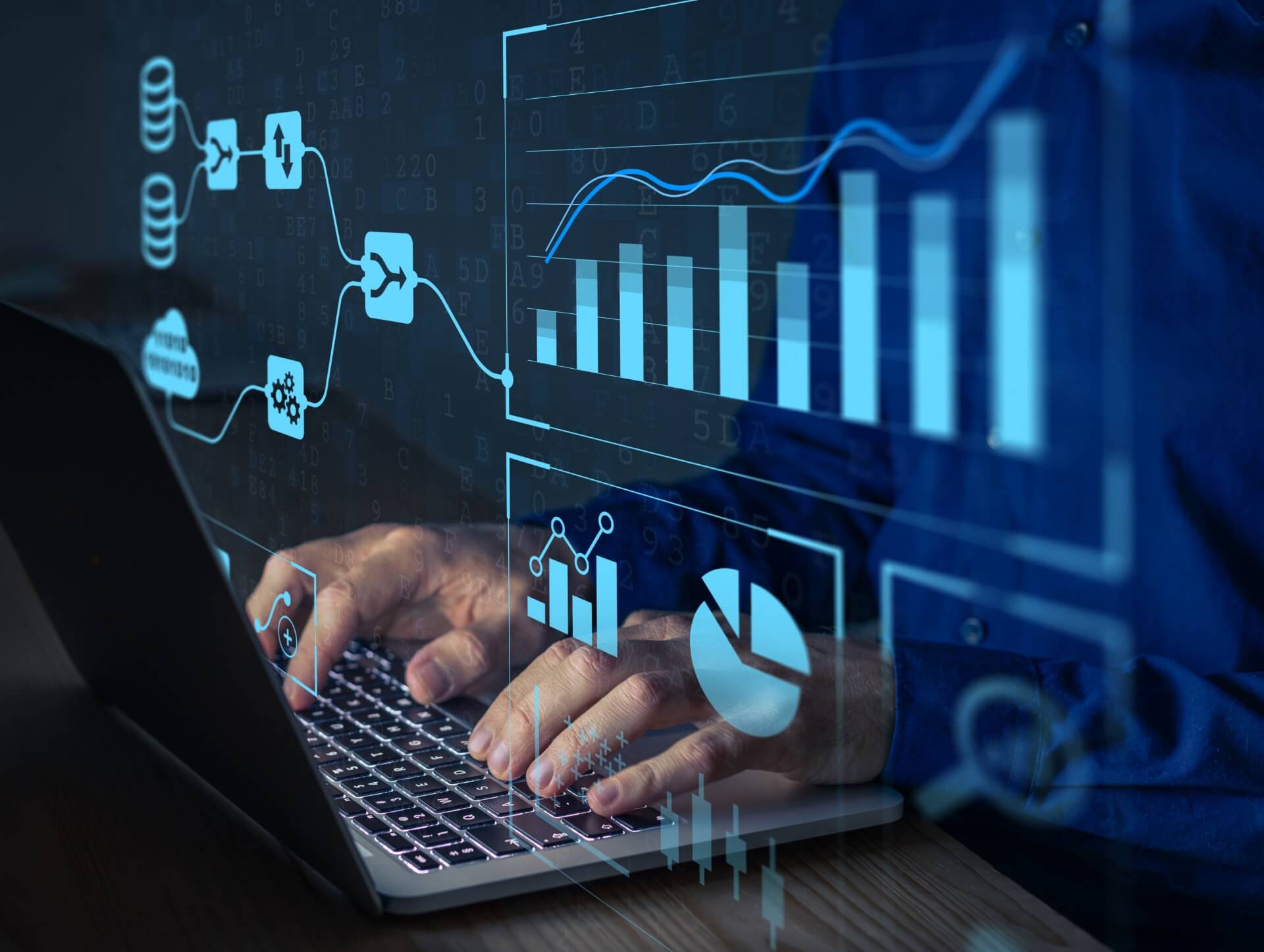
06/09/2023
Master Data Management (MDM) is a comprehensive approach to managing and maintaining an organization's critical data assets, commonly referred to as master data. The successful implementation of MDM plays a crucial role in ensuring data accuracy, consistency, and integrity, which in turn drives better business decisions and enhances operational efficiency. One of the key components of MDM implementation is data modeling, which involves the creation of a logical representation of the data and its relationships within the organization. In this article, we will explore the significance of data modeling techniques in MDM implementation and its impact on the overall success of the MDM strategy.
The Benefits of Data Modeling in MDM
Data modeling serves as a foundational element in the MDM implementation process, providing several key benefits to organizations:
1. Improved Data Quality
By employing data modeling techniques, organizations can define and enforce data quality standards and rules. Data models help identify and correct data inconsistencies, redundancies, and errors, ensuring that the master data is accurate and reliable. This, in turn, improves decision-making, enhances customer satisfaction, and reduces operational risks.
2. Enhanced Data Governance
Data modeling enables organizations to establish a robust data governance framework by defining data ownership, data stewardship roles, and data access controls. A well-designed data model ensures that data is managed consistently across the organization, aligning with regulatory compliance requirements and industry standards. This promotes data transparency, accountability, and trustworthiness.
3. Efficient Data Integration
Data modeling facilitates seamless data integration by providing a clear understanding of data relationships and dependencies. This enables organizations to integrate data from disparate sources and systems, ensuring that the master data is complete and up-to-date. With a comprehensive view of the data landscape, organizations can achieve a single source of truth and avoid data silos.
4. Scalability and Flexibility
Effective data modeling techniques enable organizations to design a scalable and flexible MDM architecture. By modeling the data entities, attributes, and relationships, organizations can accommodate future business requirements and changes without disrupting the existing data infrastructure. This agility allows organizations to adapt to evolving market dynamics and maintain a competitive edge.
Best Practices for Data Modeling in MDM
Implementing data modeling techniques in MDM requires careful planning and execution. Here are some best practices to consider:
1. Understand the Business Requirements
Prior to data modeling, it is essential to have a clear understanding of the organization's business requirements and objectives. This involves collaborating with business stakeholders to identify the critical data entities, attributes, and relationships that need to be managed within the MDM solution. By aligning data modeling with business goals, organizations can ensure that the MDM solution meets the specific needs of the organization.
2. Follow Industry Standards and Best Practices
Adhering to industry standards and best practices is crucial for effective data modeling in MDM. Organizations should leverage established data modeling methodologies, such as the Entity-Relationship (ER) model or the Unified Modeling Language (UML), to ensure consistency and compatibility with existing systems and tools. Additionally, organizations should define naming conventions, data types, and data validation rules to maintain data integrity and interoperability.
3. Collaborate with Data Stewards and Subject Matter Experts
Data modeling should be a collaborative effort involving data stewards, subject matter experts, and IT professionals. Data stewards and subject matter experts possess valuable domain knowledge and can provide insights into the data relationships and business rules. Their involvement ensures that the data model accurately represents the organization's data landscape and meets the specific requirements of different business units.
4. Continuously Monitor and Refine the Data Model
Data modeling is an iterative process that requires continuous monitoring and refinement. As the organization's data landscape evolves, the data model should be updated to reflect the changes. Regularly reviewing the data model and incorporating feedback from stakeholders helps identify potential issues and ensures that the data model remains aligned with the organization's evolving business needs.
Challenges in Data Modeling for MDM
While data modeling plays a critical role in MDM implementation, organizations may encounter certain challenges during the process:
1. Complex Data Relationships
Organizations often have complex data relationships, involving multiple data entities and hierarchies. Modeling these relationships accurately requires a deep understanding of the business processes and data dependencies. Failure to capture these relationships correctly can result in data inconsistencies and inaccuracies, undermining the effectiveness of the MDM solution.
2. Data Integration and Interoperability
Data modeling for MDM involves integrating data from various sources and systems. Ensuring data interoperability and compatibility can be challenging, especially when dealing with diverse data formats, data types, and data structures. Organizations need to establish robust data integration processes and leverage data integration technologies to overcome these challenges.
3. Data Security and Privacy
Data modeling should consider data security and privacy requirements to protect sensitive information. Organizations need to implement appropriate access controls, encryption mechanisms, and data masking techniques to safeguard the master data. Failure to address data security concerns can lead to data breaches, regulatory non-compliance, and reputational damage.
4. Maintenance and Governance
Over time, the data model may require updates and modifications to accommodate changing business needs. Ensuring efficient maintenance and governance of the data model can be challenging, especially as the organization scales and the data landscape expands. Organizations need to establish clear processes and roles for data model maintenance, version control, and change management.
Conclusion
Data modeling techniques play a critical role in the successful implementation of Master Data Management (MDM) solutions. By leveraging data modeling, organizations can achieve improved data quality, enhanced data governance, efficient data integration, and scalability. However, organizations must also consider the challenges associated with data modeling, such as complex data relationships, data integration, data security, and maintenance. By following best practices and collaborating with stakeholders, organizations can overcome these challenges and realize the full potential of MDM. In an increasingly data-driven business landscape, effective data modeling is essential for organizations to gain a competitive edge, drive innovation, and maximize the value of their data assets.
Contact us
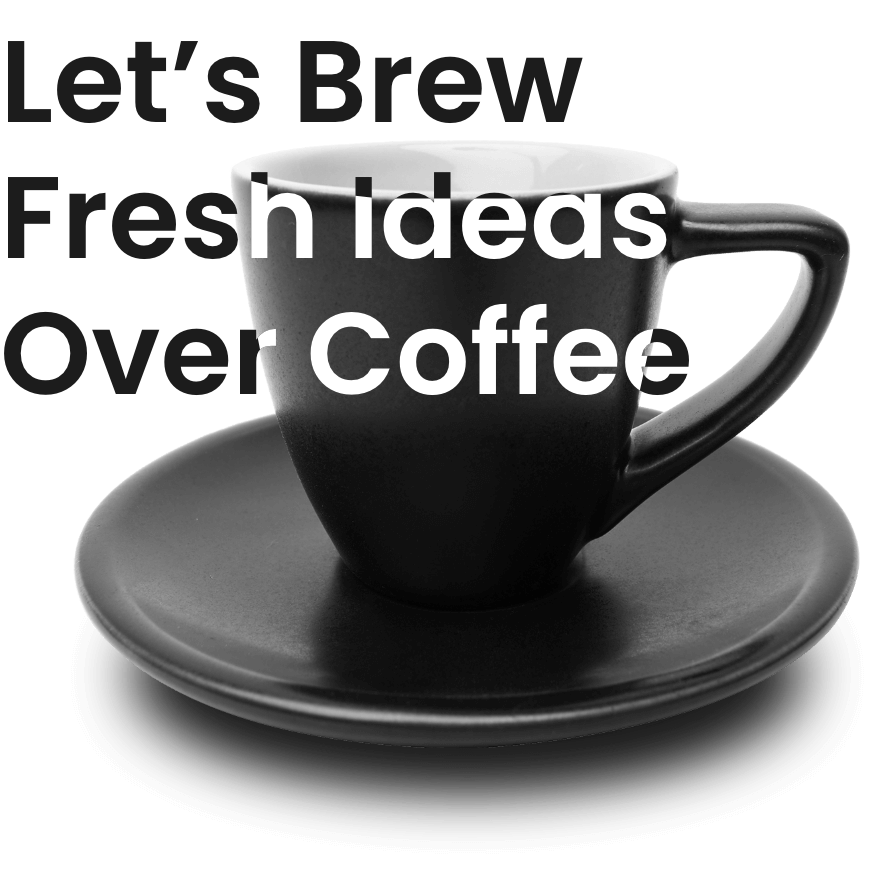
Spanning 8 cities worldwide and with partners in 100 more, we’re your local yet global agency.
Fancy a coffee, virtual or physical? It’s on us – let’s connect!