The role of data profiling in a master data management strategy
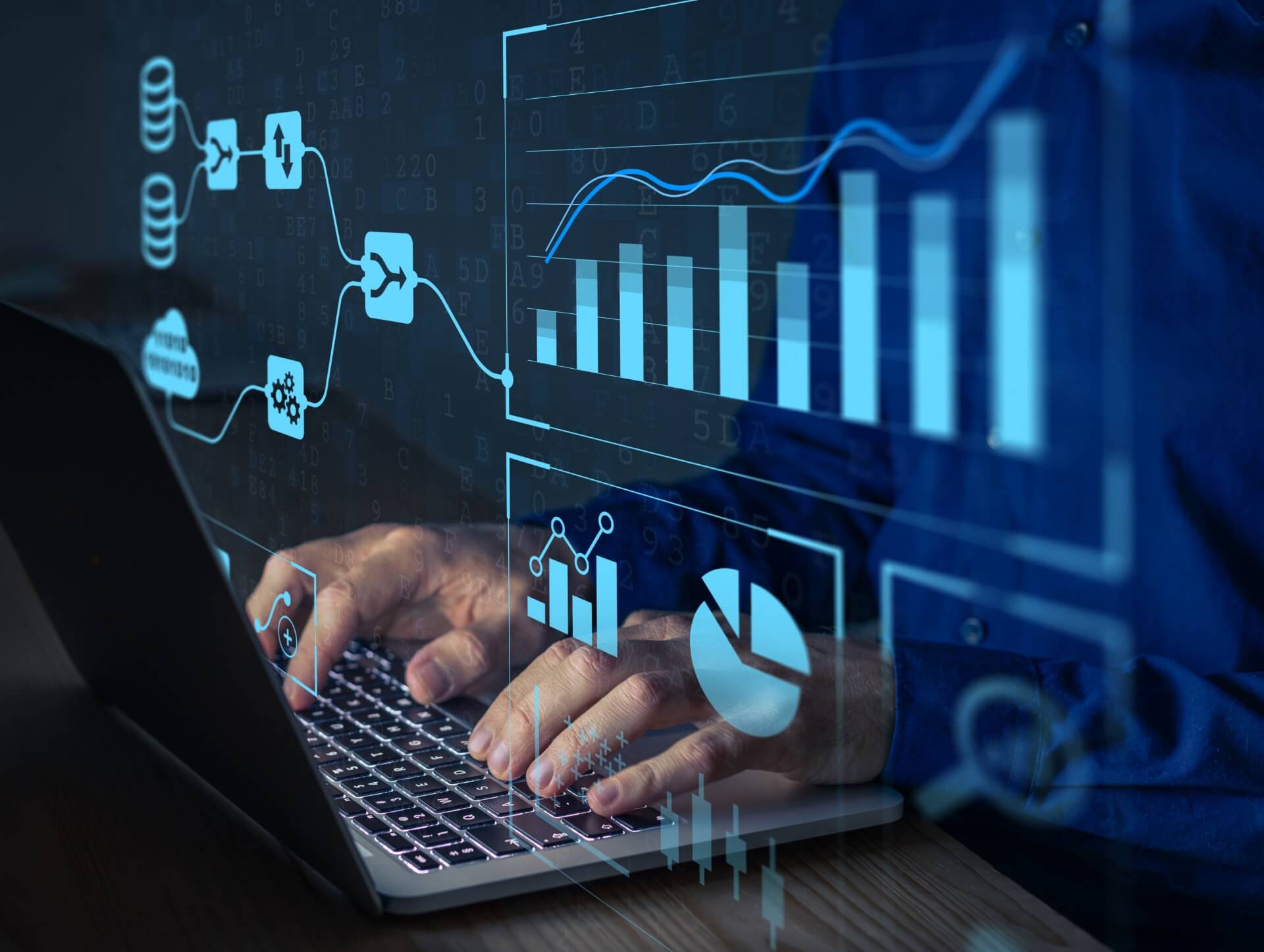
06/09/2023
In today's data-driven world, organizations are increasingly realizing the importance of effectively managing their master data. Master data refers to the critical information that is shared across multiple systems and departments within an organization, such as customer data, product data, and employee data. Master Data Management (MDM) is the process of creating a single, unified view of this master data to ensure consistency, accuracy, and reliability.
One of the key components of a successful MDM strategy is data profiling. Data profiling involves the analysis and assessment of data to understand its quality, completeness, and consistency. By profiling the data, organizations can identify any issues or inconsistencies that may exist and take appropriate actions to improve data quality. In this article, we will explore the role of data profiling in a master data management strategy, its benefits, and best practices.
The Benefits of Master Data Management
Before diving into the role of data profiling in MDM, let's first understand the benefits of implementing a master data management strategy. A well-implemented MDM strategy can bring several advantages to an organization, including:
- Improved data quality: MDM ensures that data is accurate, consistent, and up-to-date, enabling organizations to make better-informed business decisions.
- Enhanced data governance: MDM provides a framework for defining and enforcing data governance policies and procedures, ensuring compliance with regulations and industry standards.
- Increased operational efficiency: By centralizing and standardizing master data, MDM reduces data redundancy and improves data integration, resulting in streamlined business processes and increased efficiency.
- Better customer experience: MDM enables organizations to have a single, unified view of their customers, allowing them to deliver personalized and targeted experiences, leading to improved customer satisfaction and loyalty.
- Support for digital transformation: MDM provides a solid foundation for digital transformation initiatives by ensuring reliable and consistent data across systems, enabling organizations to leverage emerging technologies such as artificial intelligence and machine learning.
The Role of Data Profiling in Master Data Management
Data profiling plays a crucial role in a master data management strategy. It helps organizations understand the quality and characteristics of their data, identify data issues, and take corrective actions. Let's explore some key aspects of data profiling in MDM:
Data Quality Management
Data quality is a critical factor in the success of any MDM initiative. Poor data quality can lead to inaccurate reporting, inefficient processes, and missed business opportunities. Data profiling helps organizations assess the quality of their data by analyzing various data attributes such as completeness, accuracy, consistency, and timeliness. By identifying data quality issues, organizations can implement data cleansing and enrichment processes to improve the overall quality of their master data.
Data Governance in MDM
Data governance is the framework of policies, processes, and controls that ensure the effective and responsible management of data. Data profiling provides valuable insights into the quality and integrity of data, enabling organizations to establish and enforce data governance rules. By understanding the data lineage, data owners, and data usage patterns, organizations can implement effective data governance practices, ensuring compliance with regulations and industry standards.
MDM Best Practices
Data profiling is closely linked to MDM best practices. It helps organizations identify data quality issues, define data standards, and establish data management processes. By following best practices such as data cleansing, data standardization, and data validation, organizations can achieve accurate and reliable master data. Data profiling also helps in ongoing monitoring and measurement of data quality, ensuring that the MDM strategy is effective and sustainable.
Data Integration in MDM
One of the key challenges in MDM is integrating data from various sources and systems. Data profiling helps organizations understand the structure, format, and quality of data from different sources, facilitating the integration process. By profiling the data, organizations can identify any data inconsistencies or incompatibilities, enabling them to resolve these issues before the data is integrated into the master data management platform.
Implementing Data Profiling in a Master Data Management Strategy
Implementing data profiling in a master data management strategy involves several steps and considerations. Let's take a look at the key aspects of the implementation process:
1. Define Objectives and Scope
The first step in implementing data profiling is to define clear objectives and scope. Organizations need to identify what they want to achieve through data profiling and which data sets they want to profile. It is important to prioritize the most critical data sets and focus on specific attributes that are relevant to the business requirements.
2. Select Data Profiling Tools and Technologies
There are several data profiling tools and technologies available in the market. Organizations need to evaluate and select the most suitable tools based on their requirements, budget, and technical capabilities. Some popular data profiling tools include Informatica Data Quality, IBM InfoSphere Information Analyzer, and Talend Data Profiling.
3. Develop Data Profiling Rules and Metrics
Data profiling rules and metrics define the criteria against which the data will be analyzed. Organizations need to develop rules and metrics that align with their data quality goals and business requirements. These rules can be based on industry standards, regulatory requirements, or specific data quality dimensions such as completeness, accuracy, consistency, and validity.
4. Execute Data Profiling Processes
Once the rules and metrics are defined, organizations can execute the data profiling processes. This involves running the selected data profiling tools and technologies on the identified data sets. The tools will analyze the data, generate profiling reports, and highlight any data quality issues or anomalies.
5. Analyze and Interpret Profiling Results
After the data profiling processes are completed, organizations need to analyze and interpret the profiling results. This involves reviewing the generated reports, identifying data quality issues, and understanding the root causes of these issues. Organizations can use data visualization techniques to gain insights from the profiling results and make informed decisions.
6. Take Corrective Actions
Based on the analysis of the profiling results, organizations need to take appropriate corrective actions to improve data quality. This may involve data cleansing, data enrichment, data standardization, or data validation processes. It is important to establish data governance practices to ensure ongoing data quality management and maintenance.
7. Monitor and Measure Data Quality
Data profiling is not a one-time activity. It is an ongoing process that requires monitoring and measurement of data quality. Organizations need to establish metrics and KPIs to track the effectiveness of their data profiling efforts. Regular monitoring and measurement help in identifying any new data quality issues and taking proactive actions to address them.
Challenges in Data Profiling in MDM
While data profiling is an essential component of a master data management strategy, it comes with its own set of challenges. Some common challenges in data profiling in MDM include:
1. Data Volume and Complexity
Organizations deal with large volumes of data from multiple sources, making data profiling a complex task. Data can be structured or unstructured, and it may have different formats, making it challenging to analyze and profile effectively.
2. Data Privacy and Security
Data privacy and security are major concerns in data profiling. Organizations need to ensure that sensitive or confidential information is not exposed during the profiling process. It is important to implement appropriate security measures and comply with data protection regulations.
3. Data Integration and Data Mapping
Data profiling requires integration and mapping of data from various sources and systems. This can be a complex process, especially when dealing with heterogeneous data sources and different data models. Organizations need to invest in data integration tools and technologies to simplify this process.
4. Data Governance and Stakeholder Engagement
Effective data profiling requires strong data governance practices and stakeholder engagement. Organizations need to establish clear roles and responsibilities, define data ownership, and engage stakeholders from different departments to ensure collaboration and alignment.
Conclusion
Data profiling plays a crucial role in a master data management strategy. It helps organizations assess the quality, completeness, and consistency of their data, enabling them to make informed decisions and drive business value. By implementing data profiling best practices and leveraging the right tools and technologies, organizations can improve data quality, enhance data governance, and achieve successful MDM implementations. Data profiling is not a one-time activity but an ongoing process that requires continuous monitoring and measurement to ensure the effectiveness and sustainability of the MDM strategy.
References
- Smith, J. (2021). Master Data Management: Strategies and Best Practices. New York, NY: John Wiley & Sons.
- Johnson, A. (2020). Data Profiling in Master Data Management. Retrieved from https://www.dataversity.net/data-profiling-in-master-data-management/
- White, S. (2019). Master Data Management: A Practical Guide. San Francisco, CA: Morgan Kaufmann.
Contact us
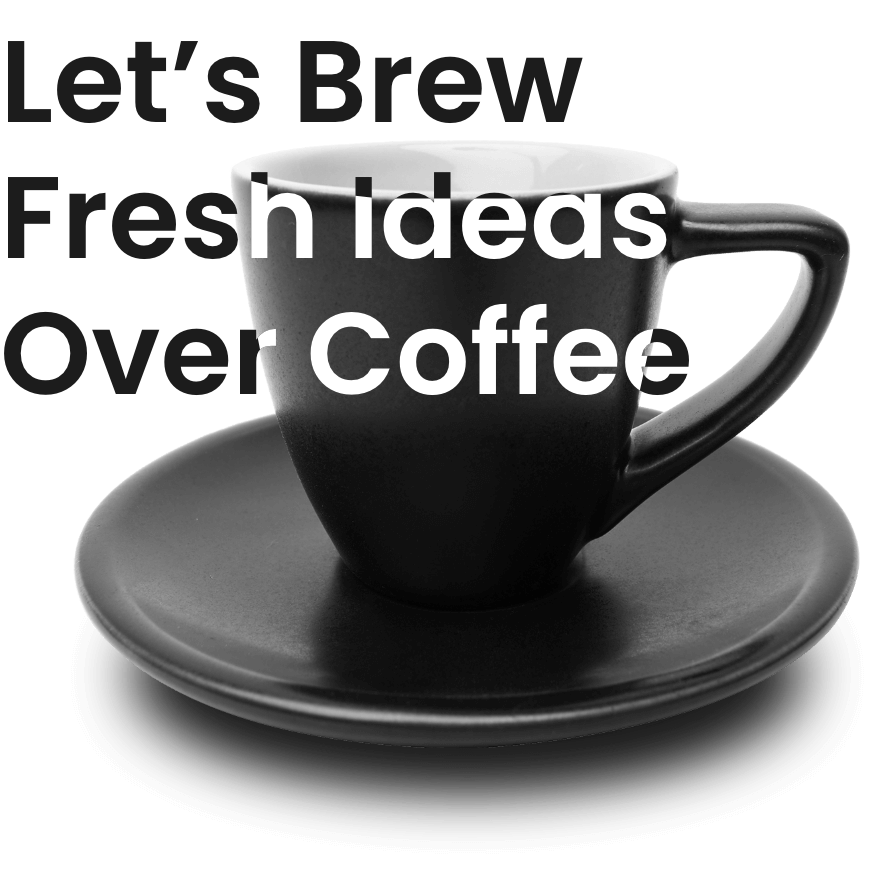
Spanning 8 cities worldwide and with partners in 100 more, we’re your local yet global agency.
Fancy a coffee, virtual or physical? It’s on us – let’s connect!