The role of data quality in a master data management strategy
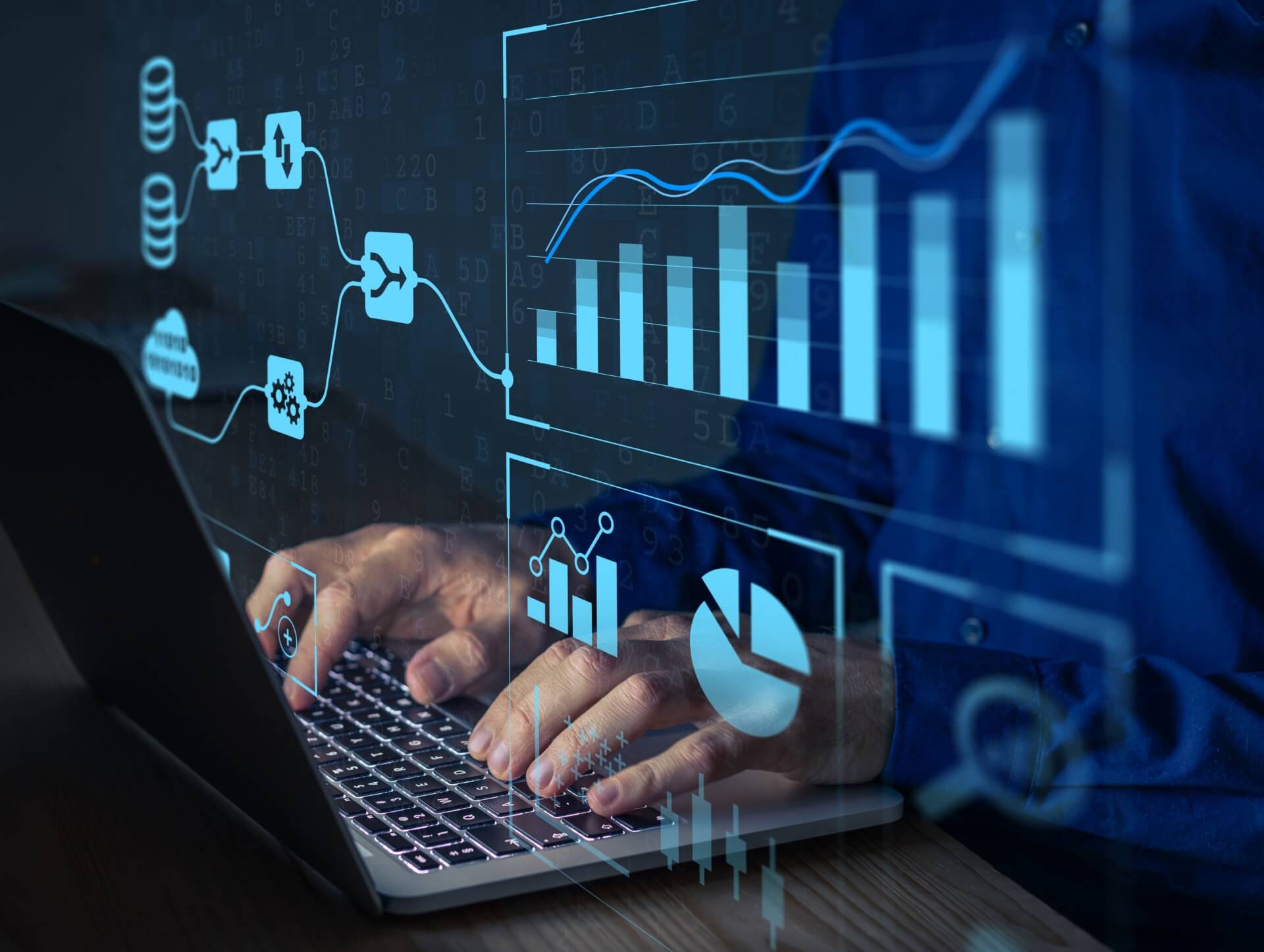
06/09/2023
In today's data-driven world, businesses rely heavily on accurate and reliable data to make informed decisions and drive growth. However, managing and maintaining data quality can be a daunting task, especially when dealing with large volumes of data from various sources.
This is where a robust master data management (MDM) strategy comes into play. MDM is a comprehensive approach to managing and integrating an organization's critical data, often referred to as master data. It involves defining data governance policies, implementing data quality management processes, and using advanced tools and technologies to ensure data consistency and accuracy.
In this article, we will explore the importance of data quality in a master data management strategy and how it can impact the overall success of an organization.
Data Quality Management in MDM
Data quality management is a critical component of any MDM strategy. It involves defining and enforcing data quality standards, implementing data cleansing and validation processes, and monitoring data quality on an ongoing basis.
High-quality data is essential for effective decision-making, as it ensures that the information used for analysis and reporting is accurate, complete, and consistent. On the other hand, poor data quality can lead to erroneous insights, inefficient operations, and missed opportunities.
By incorporating data quality management into their MDM strategy, organizations can:
- Improve decision-making: Accurate and reliable data enables organizations to make informed decisions based on trustworthy information.
- Enhance operational efficiency: Clean and consistent data reduces errors, eliminates duplication, and streamlines business processes.
- Ensure regulatory compliance: Data quality management helps organizations comply with data protection regulations and maintain data privacy.
- Drive customer satisfaction: Reliable data ensures that organizations can provide personalized and tailored experiences to their customers.
Best Practices for Data Quality Management in MDM
Implementing effective data quality management practices is crucial for a successful MDM strategy. Here are some best practices to consider:
- Define data quality standards: Clearly define the quality standards for your master data, including accuracy, completeness, consistency, and timeliness.
- Establish data governance: Implement a robust data governance framework to ensure accountability, ownership, and responsibility for data quality.
- Implement data cleansing: Regularly cleanse your data to remove duplicates, errors, and inconsistencies. Use automated tools and technologies to streamline the process.
- Perform data validation: Validate your data against predefined rules and criteria to ensure its accuracy and integrity.
- Monitor data quality: Continuously monitor your data quality using metrics and key performance indicators (KPIs). Identify and address any issues or anomalies promptly.
- Train and educate stakeholders: Provide training and education to stakeholders on the importance of data quality and their role in maintaining it.
The Impact of Data Quality on MDM
High-quality data is the foundation of a successful MDM strategy. Without accurate and reliable data, organizations cannot effectively manage and integrate their master data.
Here are some key ways in which data quality impacts MDM:
- Data integration: Data quality issues can hinder the integration of master data from various sources. Inconsistent or incomplete data can lead to errors and inaccuracies in the integrated dataset.
- Data governance: Data quality is a key component of data governance. Poor data quality can undermine the effectiveness of data governance processes and policies.
- Data security: High-quality data is essential for maintaining data security and preventing unauthorized access or breaches. Poor data quality can compromise data security and put sensitive information at risk.
- Data analytics: Accurate and reliable data is crucial for generating meaningful insights and conducting accurate data analytics. Poor data quality can lead to incorrect conclusions and unreliable analytics.
- Customer experience: Data quality directly impacts the customer experience. Inaccurate or incomplete customer data can result in poor customer service and a negative brand image.
- Business processes: Data quality issues can disrupt business processes, leading to inefficiencies, delays, and increased costs.
Data Quality and MDM Success Stories
Several organizations have successfully implemented MDM strategies with a focus on data quality, leading to significant improvements and positive outcomes. Let's explore some real-world examples:
Case Study 1: Company XYZ
Company XYZ, a global manufacturing company, implemented a data quality management program as part of their MDM strategy. They identified data quality issues in their customer master data, including duplicate records, missing information, and outdated contact details.
By implementing data cleansing and validation processes, Company XYZ was able to improve the accuracy and completeness of their customer data. This resulted in better targeting of marketing campaigns, improved customer segmentation, and increased customer satisfaction.
Case Study 2: Retailer ABC
Retailer ABC, a leading e-commerce company, faced challenges in managing their product master data. They struggled with inconsistent product descriptions, incorrect categorization, and outdated pricing information.
By implementing a robust data quality management process, Retailer ABC was able to clean and standardize their product data. This improved the accuracy and consistency of their online product catalog, resulting in better search results, increased sales, and improved customer experience.
Conclusion
Data quality is a critical component of a successful master data management strategy. By prioritizing data quality, organizations can ensure that their master data is accurate, complete, and consistent, enabling them to make informed decisions, streamline operations, and drive growth.
Implementing data quality management best practices, such as defining data quality standards, establishing data governance, and implementing data cleansing and validation processes, is essential for achieving data quality excellence.
Organizations that prioritize data quality in their MDM strategy will reap the benefits of improved decision-making, enhanced operational efficiency, regulatory compliance, and customer satisfaction.
Contact us
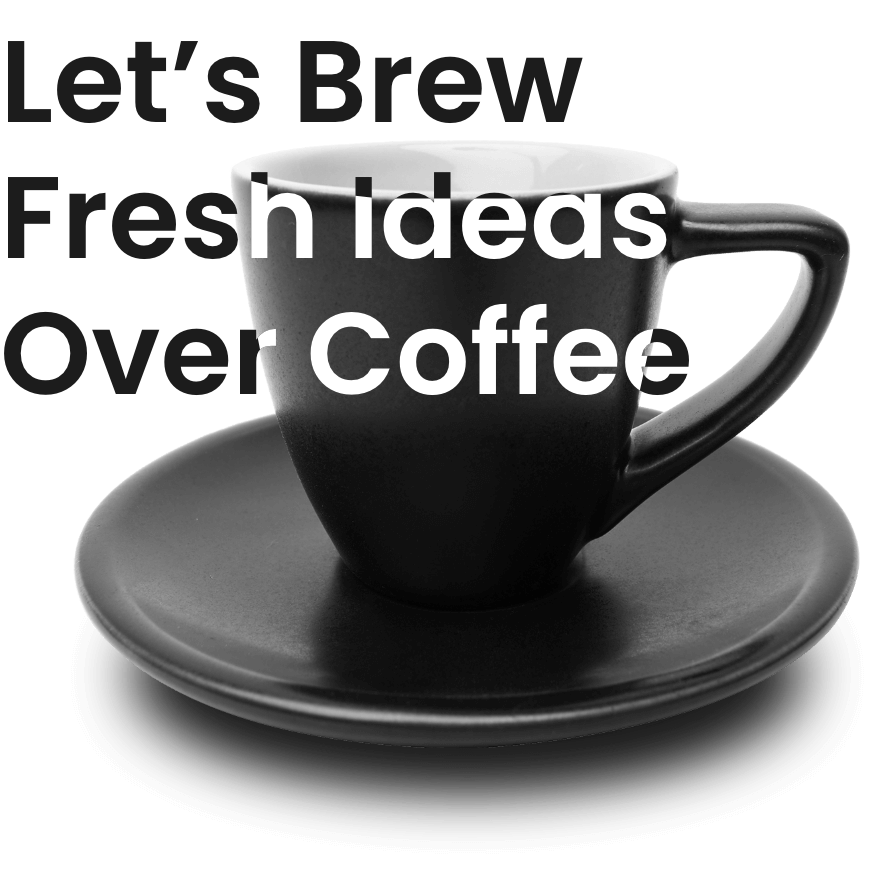
Spanning 8 cities worldwide and with partners in 100 more, we’re your local yet global agency.
Fancy a coffee, virtual or physical? It’s on us – let’s connect!