The role of data quality in MDM best practices
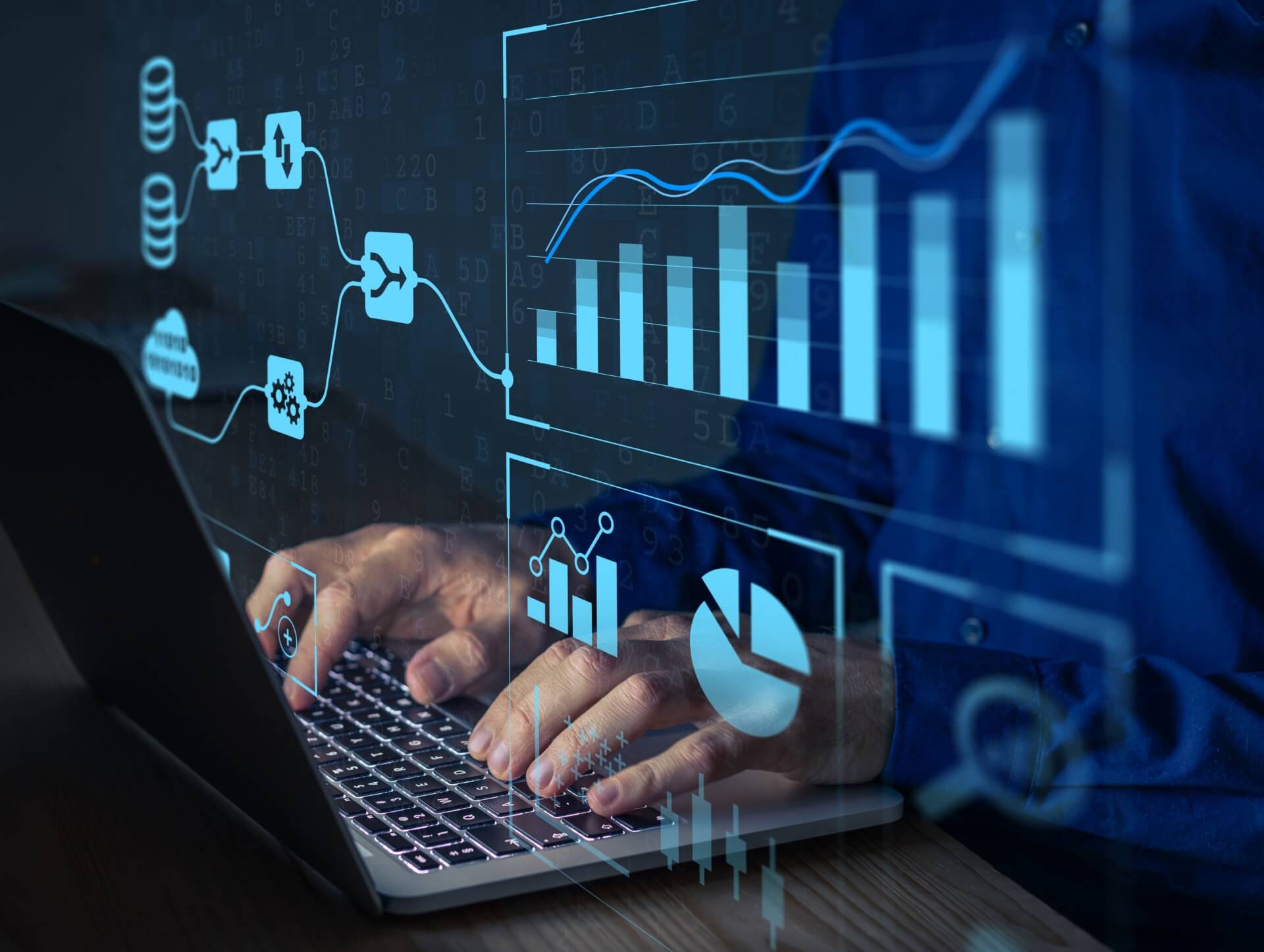
06/09/2023
Master Data Management (MDM) is a strategic approach for organizations to manage and consolidate their critical data assets in a central repository. It involves the implementation of processes, policies, and technologies to ensure the accuracy, consistency, and reliability of data across various systems and applications. One of the key factors that determine the success of an MDM initiative is the quality of data. In this article, we will explore the role of data quality in MDM best practices and its impact on overall business operations.
Data Quality Management in MDM
Data quality management is the process of defining, implementing, and maintaining the standards and procedures for ensuring the accuracy, completeness, and consistency of data. In the context of MDM, data quality management plays a crucial role in maintaining the integrity of master data. It involves various activities such as data profiling, data cleansing, data enrichment, and data validation.
Benefits of Data Quality Management in MDM
Implementing robust data quality management practices in MDM can provide several benefits to organizations. Some of the key benefits include:
- Improved Decision Making: High-quality data ensures that organizations can make informed decisions based on accurate and reliable information. This leads to improved business performance and outcomes.
- Enhanced Customer Experience: Data quality management helps in maintaining accurate and up-to-date customer information, which is essential for delivering personalized and seamless customer experiences.
- Increased Operational Efficiency: By eliminating duplicate and inconsistent data, organizations can streamline their business processes and improve operational efficiency.
- Compliance with Regulations: Data quality management ensures compliance with data privacy regulations and industry standards, reducing the risk of legal and financial penalties.
- Cost Savings: By reducing data errors and inconsistencies, organizations can avoid costly mistakes and rework, resulting in significant cost savings.
Best Practices for Data Quality Management in MDM
To achieve optimal data quality in MDM, organizations should follow these best practices:
- Define Data Quality Metrics: Organizations should define clear and measurable data quality metrics to assess the quality of master data. These metrics can include accuracy, completeness, consistency, timeliness, and uniqueness.
- Implement Data Profiling: Data profiling involves analyzing the structure, content, and relationships within the data to identify data quality issues. It helps organizations understand the quality of their data and prioritize data cleansing activities.
- Establish Data Cleansing Processes: Data cleansing involves identifying and correcting or removing inaccurate, incomplete, or inconsistent data. Organizations should establish robust data cleansing processes to ensure the integrity of master data.
- Leverage Data Enrichment: Data enrichment involves enhancing the quality and value of data by adding additional information from external sources. Organizations can leverage data enrichment techniques to improve the accuracy and completeness of their master data.
- Implement Data Validation: Data validation involves ensuring that data meets specific integrity constraints and business rules. Organizations should implement data validation processes to prevent the entry of invalid or inconsistent data into the MDM system.
The Impact of Data Quality on MDM Strategy
Data quality has a significant impact on the success of an MDM strategy. A well-defined and implemented data quality management approach ensures that organizations have reliable and consistent data to support their MDM initiatives. It enables organizations to:
- Build a strong foundation for their MDM platform by ensuring the accuracy and completeness of master data.
- Enable effective data integration by providing high-quality data to downstream systems and applications.
- Support data governance efforts by establishing data quality standards and processes.
- Improve data analytics and reporting capabilities by providing clean and reliable data for analysis.
- Enhance customer experience by maintaining accurate and up-to-date customer information.
- Ensure compliance with data privacy regulations and industry standards.
Challenges in Data Quality Management for MDM
While data quality management is crucial for MDM success, organizations often face several challenges in implementing and maintaining high data quality. Some of the common challenges include:
- Data Complexity: Organizations deal with large volumes of complex data from various sources, making it challenging to ensure data quality.
- Data Integration: Integrating data from different systems and applications can introduce data quality issues, such as duplicate and inconsistent data.
- Data Governance: Lack of clear data governance policies and processes can hinder effective data quality management in MDM.
- Change Management: Implementing data quality management practices requires organizational change and may face resistance from employees.
- Technical Limitations: Organizations may face technical limitations in terms of tools and technologies to support data quality management in MDM.
Conclusion
Data quality plays a crucial role in the success of MDM initiatives. Implementing robust data quality management practices can provide several benefits to organizations, including improved decision making, enhanced customer experience, increased operational efficiency, compliance with regulations, and cost savings. To ensure high data quality in MDM, organizations should follow best practices such as defining data quality metrics, implementing data profiling, establishing data cleansing processes, leveraging data enrichment, and implementing data validation. Despite the challenges faced, organizations can overcome them by adopting a strategic approach to data quality management and investing in the right tools and technologies.
Contact us
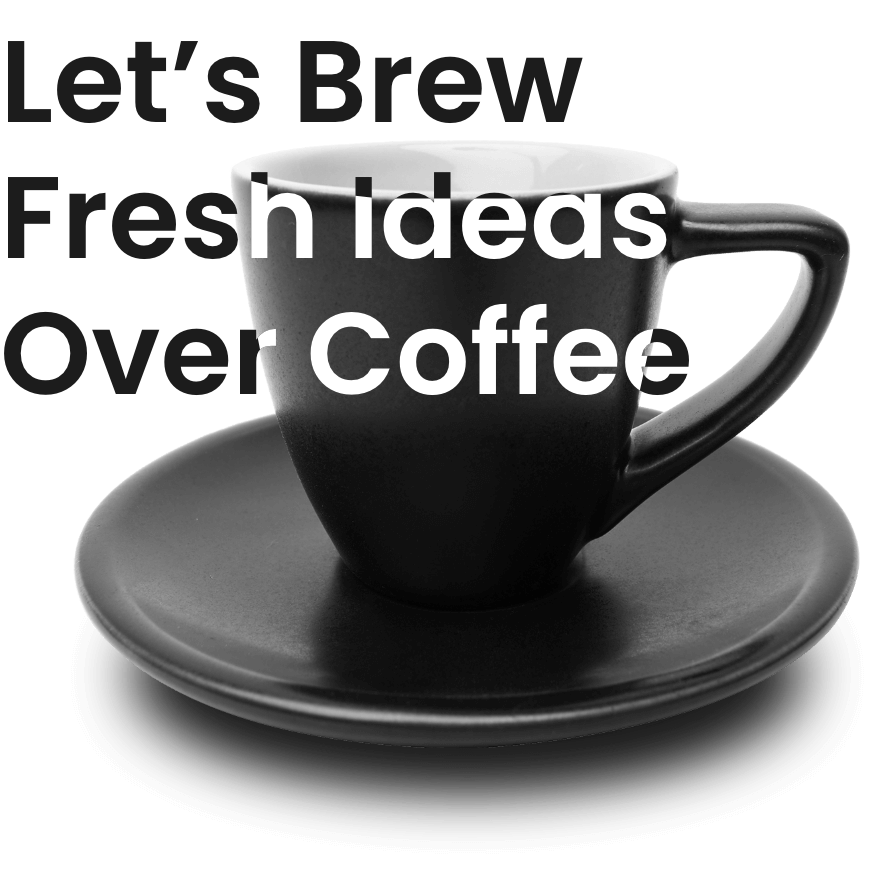
Spanning 8 cities worldwide and with partners in 100 more, we’re your local yet global agency.
Fancy a coffee, virtual or physical? It’s on us – let’s connect!