The role of master data management in the automotive industry
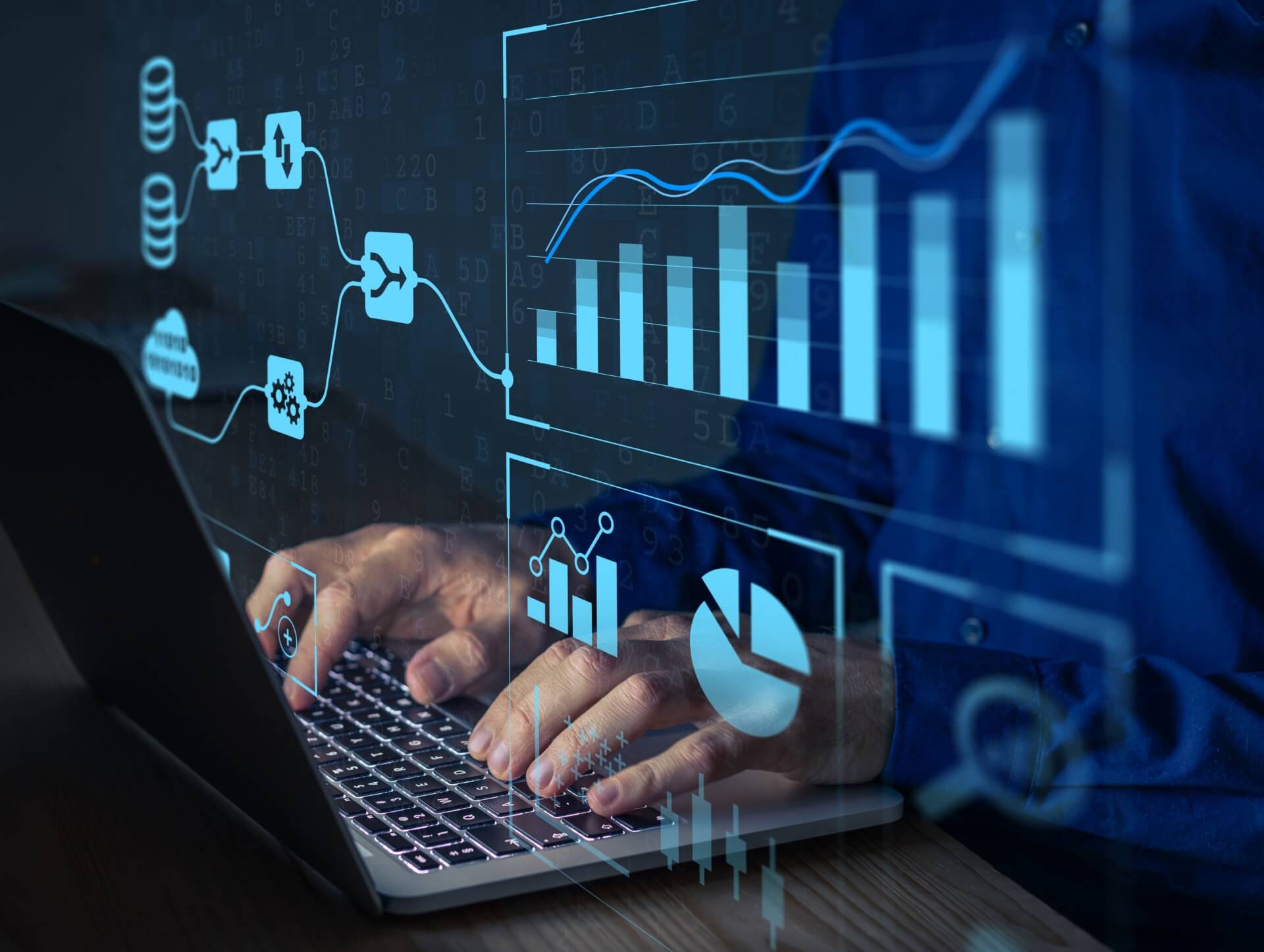
06/09/2023
The automotive industry is one of the most complex and data-driven industries in the world. From manufacturing and supply chain management to sales and customer service, every aspect of the automotive business relies heavily on accurate and reliable data. This is where master data management (MDM) comes into play.
What is Master Data Management?
Master data management refers to the processes, technologies, and strategies used to manage an organization's critical data assets. It involves creating and maintaining a single, trusted source of master data that can be used by different departments and systems across the organization. Master data includes essential information about customers, products, suppliers, locations, and more.
MDM solutions provide a centralized platform for data governance, data quality management, and data integration. By implementing MDM, organizations can ensure data consistency, accuracy, and completeness, leading to improved operational efficiency, better decision-making, and enhanced customer experience.
The Benefits of Master Data Management
Implementing a robust master data management strategy in the automotive industry can bring several benefits:
Data Consistency and Accuracy
With MDM, automotive companies can maintain consistent and accurate data across all systems and departments. This ensures that everyone is working with the same information, reducing the risk of errors and improving data quality.
Improved Operational Efficiency
By centralizing and standardizing master data, MDM eliminates data silos and redundant processes. This streamlines operations and reduces the time and effort required to access and update data. For example, in the automotive manufacturing process, MDM can help optimize supply chain management by providing real-time visibility into inventory levels, demand, and production capacity.
Enhanced Customer Experience
MDM enables automotive companies to have a 360-degree view of their customers by consolidating and integrating customer data from different touchpoints. This allows companies to personalize their marketing campaigns, improve customer service, and provide a seamless experience across all channels.
Master Data Management Solutions
There are various master data management solutions available in the market, ranging from on-premises to cloud-based platforms. Some popular MDM solutions include:
Open Source Master Data Management
Open source MDM solutions provide flexibility and cost-effectiveness. They allow organizations to customize the software according to their specific needs and integrate it with other systems. Examples of open source MDM solutions include Talend, MuleSoft, and Apache MDM.
Cloud Master Data Management
Cloud-based MDM solutions offer scalability, agility, and accessibility. They eliminate the need for on-premises infrastructure and provide real-time access to master data from anywhere. Some popular cloud MDM providers include Informatica, Reltio, and Stibo Systems.
Master Data Management Best Practices
Implementing master data management successfully requires following best practices:
Data Governance in MDM
Establish a data governance framework to define data ownership, roles, and responsibilities. This ensures that data is managed and maintained consistently across the organization. Data stewards should be appointed to enforce data governance policies and resolve data-related issues.
Data Quality Management
Data quality is crucial for effective MDM. Implement data cleansing and validation processes to eliminate duplicates, standardize data formats, and ensure data accuracy. Regularly monitor and measure data quality metrics to identify and address data quality issues.
MDM Strategy for Businesses
Develop a clear MDM strategy aligned with your organization's goals and objectives. Identify the key data domains and entities to be managed, define data governance processes, and establish a roadmap for MDM implementation.
MDM Implementation Process
Follow a structured approach for MDM implementation, starting with data profiling and analysis, data modeling and mapping, data integration and consolidation, and data governance and stewardship. Test the MDM solution thoroughly before deploying it and provide training to users for effective adoption.
Master Data Governance
Master data governance is a crucial component of MDM. It involves defining policies, procedures, and standards for managing and maintaining master data throughout its lifecycle. Data governance ensures data integrity, compliance with regulations, and alignment with business rules.
MDM Tools and Technologies
There are several MDM tools and technologies available to support MDM initiatives:
Data Integration in MDM
Data integration tools like Informatica PowerCenter, Talend Data Integration, and IBM InfoSphere enable seamless integration of master data from various sources and systems.
Master Data Modeling
Master data modeling tools like SAP PowerDesigner and erwin Data Modeler help in designing and visualizing the relationships and attributes of master data entities.
MDM and Data Security
MDM solutions should include robust security features to protect sensitive master data. Encryption, access controls, and audit trails should be implemented to ensure data security and compliance with data protection regulations.
MDM and Data Analytics
Integrating MDM with data analytics tools like Tableau, QlikView, and Power BI enables organizations to gain valuable insights from their master data. Analytics can help identify trends, patterns, and anomalies in data, leading to better decision-making.
Challenges in MDM
Implementing MDM in the automotive industry can be challenging due to various factors:
Data Complexity and Volume
The automotive industry deals with large volumes of complex data from multiple sources, including product data, vehicle configurations, customer data, and more. Managing and integrating this data can be challenging.
Data Governance and Stakeholder Alignment
Establishing data governance processes and ensuring stakeholder alignment can be difficult, especially in organizations with multiple departments and systems. It requires strong leadership, communication, and collaboration.
Data Integration and Legacy Systems
Integrating master data from legacy systems and disparate sources can be a complex task. Data mapping, data cleansing, and data transformation may be required to achieve data consistency and accuracy.
MDM Case Studies
Several automotive companies have successfully implemented MDM to address their data management challenges:
Case Study 1: BMW
BMW implemented an MDM solution to consolidate and manage its product data across different regions and systems. This enabled BMW to streamline its product launch processes, reduce time-to-market, and improve data accuracy.
Case Study 2: Ford
Ford implemented an MDM solution to integrate and manage customer data from various touchpoints. This allowed Ford to personalize its marketing campaigns, improve customer segmentation, and enhance the overall customer experience.
MDM in Multi-Cloud Environments
The automotive industry is increasingly adopting multi-cloud environments for various applications and services. MDM in multi-cloud environments involves integrating and managing master data across different cloud platforms. This requires careful planning, data governance, and data integration strategies.
MDM ROI and Value
The return on investment (ROI) and value of effective MDM in the automotive industry can be significant:
Data Governance in Lifecycle Management
Data governance is crucial throughout the data lifecycle, from data creation to data archiving. MDM ensures that data is managed consistently and compliantly throughout its lifecycle, reducing the risk of data breaches and non-compliance.
Data Security throughout the Lifecycle
MDM solutions include robust security features to protect data throughout its lifecycle. Encryption, access controls, and data masking techniques help safeguard sensitive master data from unauthorized access.
Data Compliance in Management
MDM enables organizations to comply with data protection regulations, such as GDPR and CCPA, by ensuring data accuracy, consent management, and data subject rights. This reduces the risk of regulatory penalties and reputational damage.
Master Data in Lifecycle Management
Managing master data effectively throughout its lifecycle improves data quality, reduces data duplication, and enhances data consistency. This leads to better decision-making, improved operational efficiency, and increased customer satisfaction.
Metadata Management in Data Lifecycle
Metadata management is essential for effective data lifecycle management. MDM solutions provide capabilities to capture, store, and manage metadata associated with master data, enabling better data discovery, data lineage, and data governance.
Automation in Data Lifecycle
Automation plays a crucial role in data lifecycle management. MDM solutions can automate data integration, data cleansing, and data validation processes, reducing manual efforts and improving efficiency.
Cloud-Based Data Lifecycle
Cloud-based MDM solutions offer scalability, agility, and cost-effectiveness in managing the data lifecycle. They provide real-time access to master data, enable seamless collaboration, and support data governance across distributed teams.
Analytics in Data Lifecycle
Integrating MDM with data analytics tools enables organizations to gain valuable insights from their master data throughout its lifecycle. Analytics can help identify data patterns, trends, and anomalies, leading to proactive decision-making and continuous improvement.
Challenges in Data Lifecycle Management
Data lifecycle management in the automotive industry can face several challenges:
Data Proliferation and Variety
The automotive industry generates vast amounts of data from various sources, including IoT devices, sensors, social media, and more. Managing and analyzing this diverse data can be challenging.
Data Privacy and Security
Data privacy and security are critical in the automotive industry, considering the sensitivity of customer data and intellectual property. Ensuring data privacy and security throughout the data lifecycle requires robust security measures and compliance with regulations.
Data Quality and Integrity
Data quality and integrity are essential throughout the data lifecycle. Ensuring data accuracy, completeness, and consistency requires data cleansing, data validation, and data standardization processes.
Best Practices for Lifecycle Management
Implementing effective data lifecycle management in the automotive industry requires following best practices:
Data Governance and Stewardship
Establish a data governance framework with clear roles, responsibilities, and processes. Appoint data stewards to ensure data quality, compliance, and alignment with business rules throughout the data lifecycle.
Data Integration and Consolidation
Implement data integration and consolidation processes to bring together data from various sources and systems. This ensures a unified view of data and enables effective data analysis and decision-making.
Data Quality Management
Implement data quality management processes to ensure data accuracy, completeness, and consistency. This involves data profiling, data cleansing, data validation, and ongoing monitoring of data quality metrics.
Data Privacy and Security
Implement robust data privacy and security measures throughout the data lifecycle. This includes encryption, access controls, data masking, and compliance with data protection regulations.
Continuous Improvement and Innovation
Data lifecycle management is an ongoing process. Continuously monitor and improve data management processes, embrace new technologies and best practices, and stay updated with the latest industry trends.
ROI of Effective Data Lifecycle
The ROI of effective data lifecycle management in the automotive industry can be significant:
Improved Data Quality and Accuracy
Effective data lifecycle management ensures data quality and accuracy throughout the data lifecycle. This leads to better decision-making, reduced operational costs, and improved customer satisfaction.
Enhanced Regulatory Compliance
Data lifecycle management helps organizations comply with data protection regulations, such as GDPR and CCPA. This reduces the risk of regulatory penalties and reputational damage.
Optimized Data Storage and Management
Effective data lifecycle management enables organizations to optimize data storage and management costs. By identifying and archiving non-essential data, organizations can reduce storage requirements and improve overall data management efficiency.
Improved Data Analytics and Insights
Effective data lifecycle management provides accurate and timely data for analytics. This enables organizations to gain valuable insights, identify trends, and make data-driven decisions.
Increased Operational Efficiency
Effective data lifecycle management streamlines data-related processes, reduces manual efforts, and improves overall operational efficiency. This leads to cost savings and faster time-to-market.
Contact us
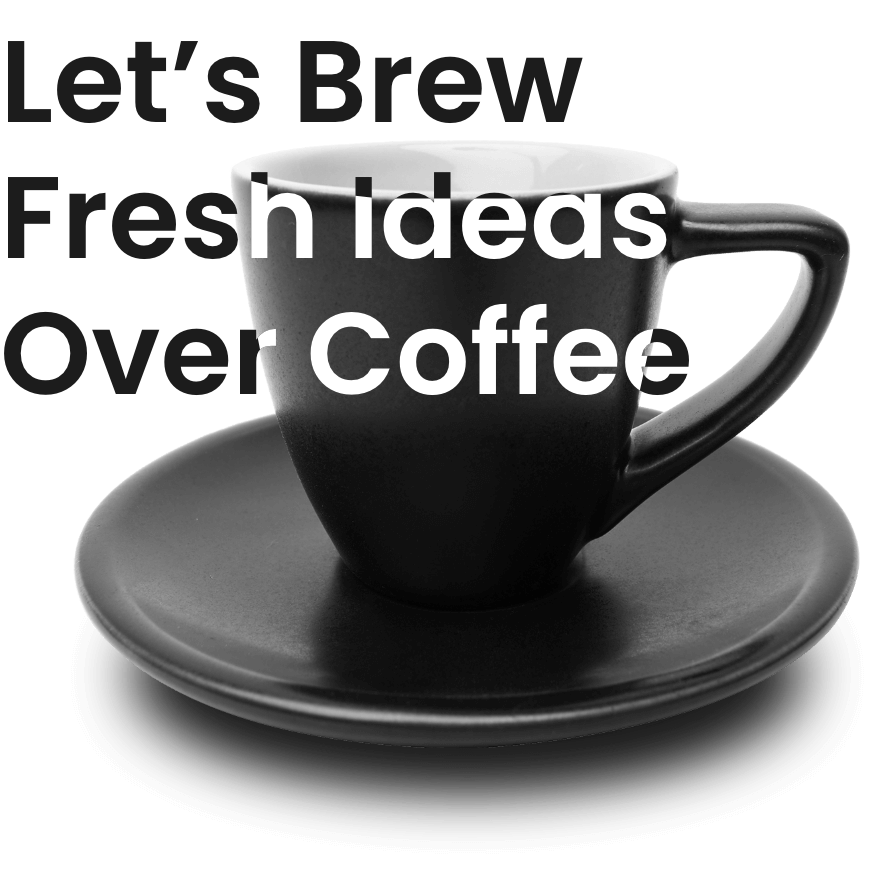
Spanning 8 cities worldwide and with partners in 100 more, we’re your local yet global agency.
Fancy a coffee, virtual or physical? It’s on us – let’s connect!