The role of master data modeling in data analytics for fraud detection
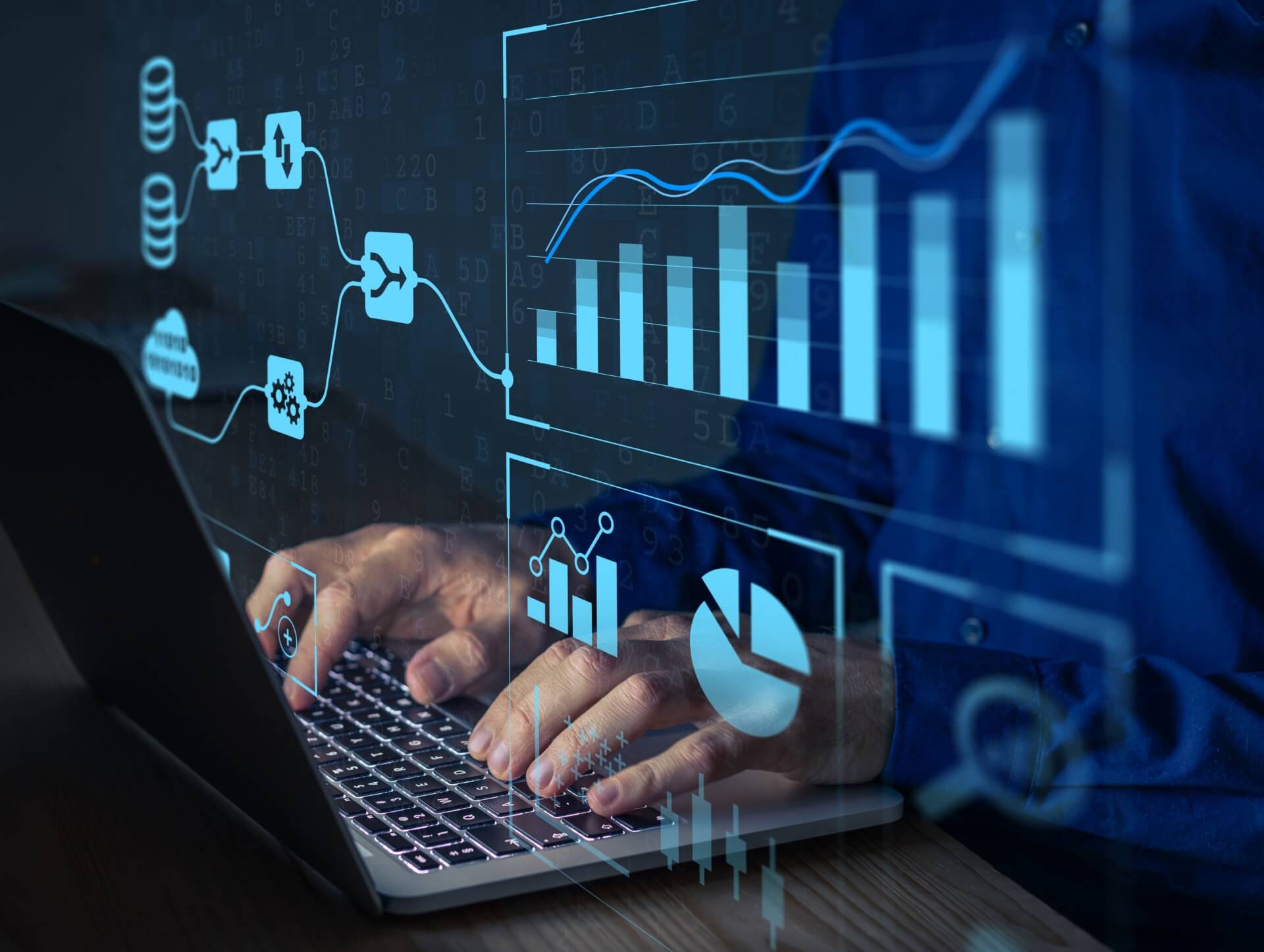
06/09/2023
In today's data-driven world, organizations face numerous challenges in managing and analyzing vast amounts of data. One of the critical challenges is detecting and preventing fraud. Fraudulent activities can result in significant financial losses, damage to reputation, and legal consequences. To effectively address fraud, organizations need to leverage advanced analytics techniques and technologies, such as machine learning and artificial intelligence, to detect and predict fraudulent patterns and behaviors. However, the success of these analytics efforts relies heavily on the quality and accuracy of the underlying data. This is where master data modeling plays a crucial role.
The Importance of Master Data Management
Master data management (MDM) is a comprehensive approach to managing and integrating an organization's data assets. It involves the creation, maintenance, and governance of a single, trusted source of master data that can be shared across different systems and applications. By implementing a robust MDM strategy, organizations can ensure data consistency, accuracy, and completeness, which are essential for effective data analytics and fraud detection.
Benefits of Master Data Management
Implementing a master data management solution offers several benefits for organizations seeking to enhance their data analytics capabilities and improve fraud detection:
Data Consistency and Accuracy
A key advantage of MDM is the ability to establish a single, authoritative source of master data. This ensures that all data elements are consistent and accurate across different systems and applications. In the context of fraud detection, consistent and accurate data is essential for identifying patterns, anomalies, and suspicious activities.
Improved Data Quality
MDM also enables organizations to improve data quality by implementing data governance processes and data quality management techniques. Data governance in MDM involves defining data standards, policies, and procedures to ensure data integrity and compliance. Data quality management includes activities such as data cleansing, data enrichment, and data validation. By improving data quality, organizations can enhance the effectiveness of their fraud detection algorithms and models.
Enhanced Data Integration
Another benefit of MDM is improved data integration. MDM platforms provide capabilities for integrating data from various sources and systems, including structured and unstructured data. This allows organizations to leverage a wide range of data sources for fraud detection, including transactional data, customer data, social media data, and more. By integrating diverse data sources, organizations can gain deeper insights into fraudulent activities and detect previously unknown patterns.
Streamlined Data Governance
MDM also facilitates streamlined data governance processes. Data governance in MDM involves defining roles, responsibilities, and workflows for managing master data. It ensures that data is properly classified, labeled, and protected throughout its lifecycle. Effective data governance is crucial for maintaining data security and privacy, which are paramount in fraud detection efforts.
Implementing Master Data Management
Implementing a master data management solution requires careful planning and execution. Here are some key steps to consider:
Define MDM Strategy
Before implementing an MDM solution, organizations need to define their MDM strategy. This involves identifying the key objectives, stakeholders, and requirements for the MDM initiative. Organizations should also assess their current data management practices and identify areas for improvement. By establishing a clear strategy, organizations can align their MDM efforts with their overall business goals and objectives.
Select MDM Tools and Technologies
Once the MDM strategy is defined, organizations need to select the right MDM tools and technologies. There are various MDM solutions available in the market, ranging from open source MDM platforms to cloud-based MDM services. Organizations should evaluate different options based on their specific requirements and budget. It is also important to consider factors such as scalability, flexibility, and ease of integration with existing systems.
Design MDM Architecture
Designing the MDM architecture is a critical step in the implementation process. The MDM architecture should define how master data will be stored, managed, and accessed. It should also address data integration and data governance requirements. The architecture should be designed to support the organization's current and future data analytics needs, including fraud detection. It is essential to involve stakeholders from different departments, such as IT, data governance, and analytics, to ensure a comprehensive and effective design.
Implement MDM Solution
Once the MDM architecture is defined, organizations can proceed with the implementation of the MDM solution. This involves setting up the necessary infrastructure, configuring the MDM software, and migrating existing data into the MDM platform. It is important to follow best practices and guidelines provided by the MDM solution provider to ensure a smooth and successful implementation.
Best Practices for Master Data Modeling
Master data modeling is a critical component of MDM and plays a significant role in data analytics for fraud detection. Here are some best practices to consider when modeling master data:
Understand the Business Requirements
Before starting the master data modeling process, it is important to have a clear understanding of the organization's business requirements. This includes understanding the data entities, attributes, and relationships that are relevant to fraud detection. By aligning the master data model with the business requirements, organizations can ensure that the model accurately represents the real-world entities and their relationships.
Follow Standard Data Modeling Techniques
When modeling master data, it is essential to follow standard data modeling techniques, such as entity-relationship modeling or dimensional modeling. These techniques provide a structured approach to representing data entities, attributes, and relationships. They help ensure consistency, clarity, and accuracy in the master data model.
Consider Data Security and Privacy
Data security and privacy are critical considerations in master data modeling, especially in the context of fraud detection. Organizations should define appropriate access controls, encryption mechanisms, and data masking techniques to protect sensitive information. It is also important to comply with relevant data protection regulations, such as GDPR or CCPA.
Ensure Data Integration and Interoperability
Master data modeling should facilitate data integration and interoperability across different systems and applications. This includes defining standard data formats, data mappings, and data transformation rules. By ensuring data integration and interoperability, organizations can leverage the full potential of their data assets for fraud detection.
Conclusion
Master data modeling plays a crucial role in data analytics for fraud detection. By implementing a robust master data management strategy and leveraging advanced analytics techniques, organizations can enhance their fraud detection capabilities, improve data quality, and mitigate financial and reputational risks. However, it is important to follow best practices in master data modeling and ensure data security and privacy throughout the data lifecycle. With the right approach and technology, organizations can effectively detect, prevent, and mitigate fraud, safeguarding their assets and maintaining customer trust.
Contact us
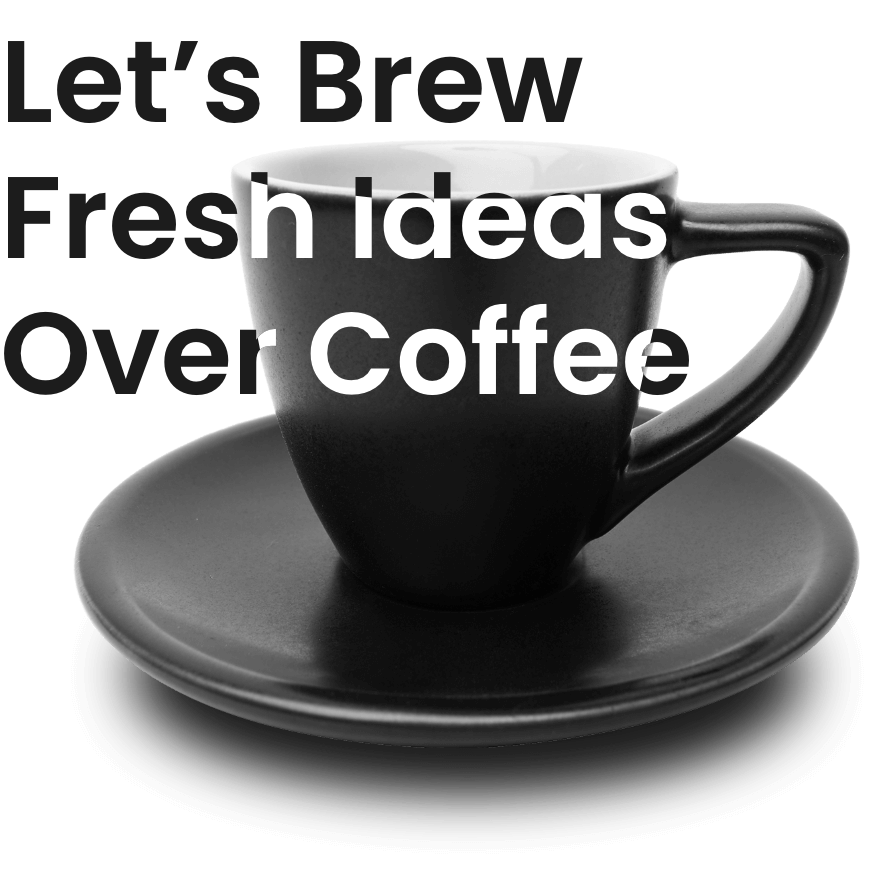
Spanning 8 cities worldwide and with partners in 100 more, we’re your local yet global agency.
Fancy a coffee, virtual or physical? It’s on us – let’s connect!