The role of master data modeling in data analytics for risk management
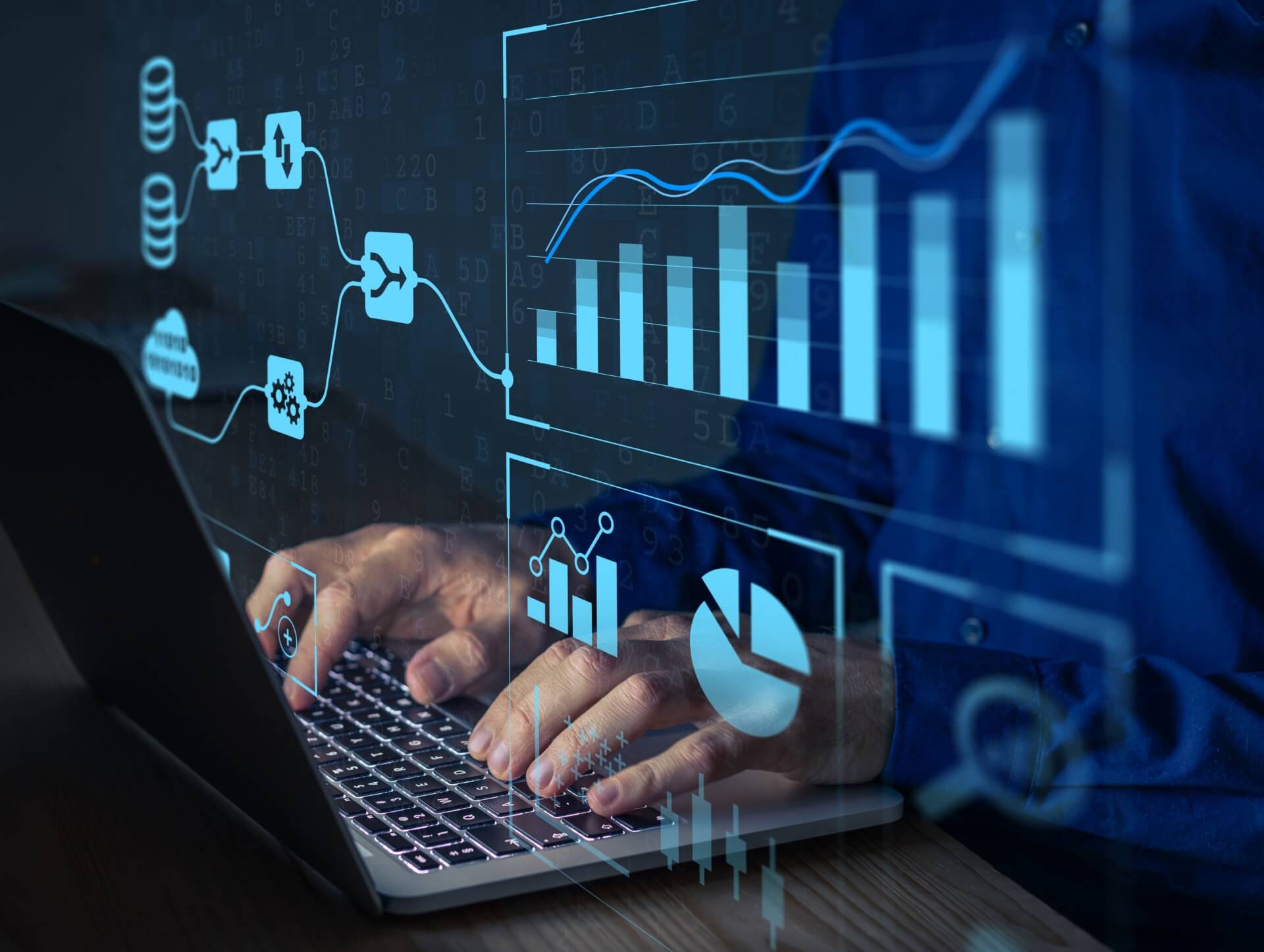
06/09/2023
In today's data-driven world, organizations are constantly collecting and analyzing vast amounts of data to gain valuable insights and make informed decisions. However, with the increasing volume, variety, and velocity of data, it has become crucial for businesses to effectively manage and model their data to ensure accuracy, consistency, and reliability. This is where master data modeling plays a vital role.
What is Master Data Modeling?
Master data modeling is the process of creating a unified and consistent view of an organization's critical data entities, such as customers, products, suppliers, and employees. It involves defining the structure, relationships, and attributes of these data entities to establish a single source of truth. By implementing a well-designed master data model, organizations can ensure data integrity, improve data quality, and enable effective data governance.
The Benefits of Master Data Modeling
Implementing a robust master data modeling strategy offers numerous benefits for organizations:
- Improved Data Quality: A well-defined master data model helps identify and correct data inconsistencies, redundancies, and inaccuracies, leading to improved data quality.
- Enhanced Data Governance: Master data modeling provides a framework for establishing data governance policies, standards, and processes, ensuring data is managed and used in a controlled and compliant manner.
- Increased Operational Efficiency: By establishing a single source of truth, master data modeling reduces data duplication and redundancy, streamlines processes, and improves overall operational efficiency.
- Better Decision Making: Accurate and reliable master data enables organizations to make better-informed decisions, as it provides a holistic view of the business and its operations.
- Improved Customer Experience: Master data modeling allows organizations to gain a comprehensive understanding of their customers, enabling personalized and targeted marketing campaigns, improved customer service, and enhanced customer experience.
Master Data Modeling and Data Analytics
Data analytics plays a crucial role in risk management, as it helps organizations identify, assess, and mitigate potential risks. Master data modeling provides a solid foundation for data analytics by ensuring data accuracy, consistency, and completeness. Here's how master data modeling supports data analytics for risk management:
Data Integration and Data Quality Management
Effective data integration is essential for accurate and meaningful data analytics. Master data modeling enables organizations to integrate multiple data sources, such as internal systems, external databases, and third-party data, into a unified and consistent view. By establishing data relationships and mapping, master data modeling ensures data consistency and improves data quality, leading to reliable and accurate analytics results.
Master Data Modeling for Enterprise Scalability
As organizations grow and expand, the volume and complexity of data also increase. Master data modeling provides a scalable framework for managing and modeling data across the enterprise. It allows organizations to easily add new data entities, modify existing ones, and adapt to changing business requirements. With a well-designed master data model, organizations can efficiently scale their data analytics capabilities and accommodate future growth.
Master Data Modeling and Data Security
Data security is a critical aspect of risk management. Master data modeling helps organizations establish data security measures and controls by defining access rights, data classifications, and data protection policies. By implementing a secure master data management platform, organizations can ensure that sensitive data is protected throughout its lifecycle, reducing the risk of data breaches and unauthorized access.
Challenges in Master Data Modeling
While master data modeling offers numerous benefits, organizations may encounter several challenges during the implementation process. Some of the common challenges include:
- Data Complexity: Organizations often deal with complex data structures and relationships, which can make the master data modeling process challenging.
- Data Governance: Establishing effective data governance practices and ensuring compliance with data regulations can be a complex task.
- Data Integration: Integrating data from multiple sources and systems can be a time-consuming and resource-intensive process.
- Change Management: Implementing a master data modeling strategy requires organizational change and may face resistance from stakeholders.
- Technical Expertise: Organizations need skilled professionals with expertise in data modeling, data management, and data analytics to effectively implement and maintain a master data model.
Best Practices for Master Data Modeling
To overcome the challenges and ensure a successful master data modeling implementation, organizations should follow these best practices:
- Define Clear Objectives: Clearly define the objectives and goals of the master data modeling initiative to ensure alignment with the organization's overall strategy.
- Engage Stakeholders: Involve key stakeholders from different departments and functions to gain their support and ensure their requirements are met.
- Establish Data Governance Framework: Develop a comprehensive data governance framework to ensure data integrity, security, and compliance throughout the master data modeling process.
- Invest in Training and Education: Provide training and education to employees involved in the master data modeling process to enhance their skills and knowledge.
- Implement Data Quality Management: Incorporate data quality management practices, such as data cleansing, data validation, and data enrichment, to improve the accuracy and reliability of the master data.
- Adopt Agile Methodologies: Implement agile methodologies and iterative approaches to ensure flexibility and adaptability during the master data modeling process.
- Monitor and Measure: Continuously monitor and measure the performance of the master data model to identify areas for improvement and make necessary adjustments.
Conclusion
Master data modeling plays a critical role in data analytics for risk management. By implementing a well-designed master data model, organizations can ensure data accuracy, consistency, and reliability, leading to improved data analytics results and better-informed decision making. However, organizations need to overcome challenges and follow best practices to successfully implement and maintain a master data model. With the right approach, master data modeling can be a powerful tool for organizations to manage and leverage their data effectively.
Contact us
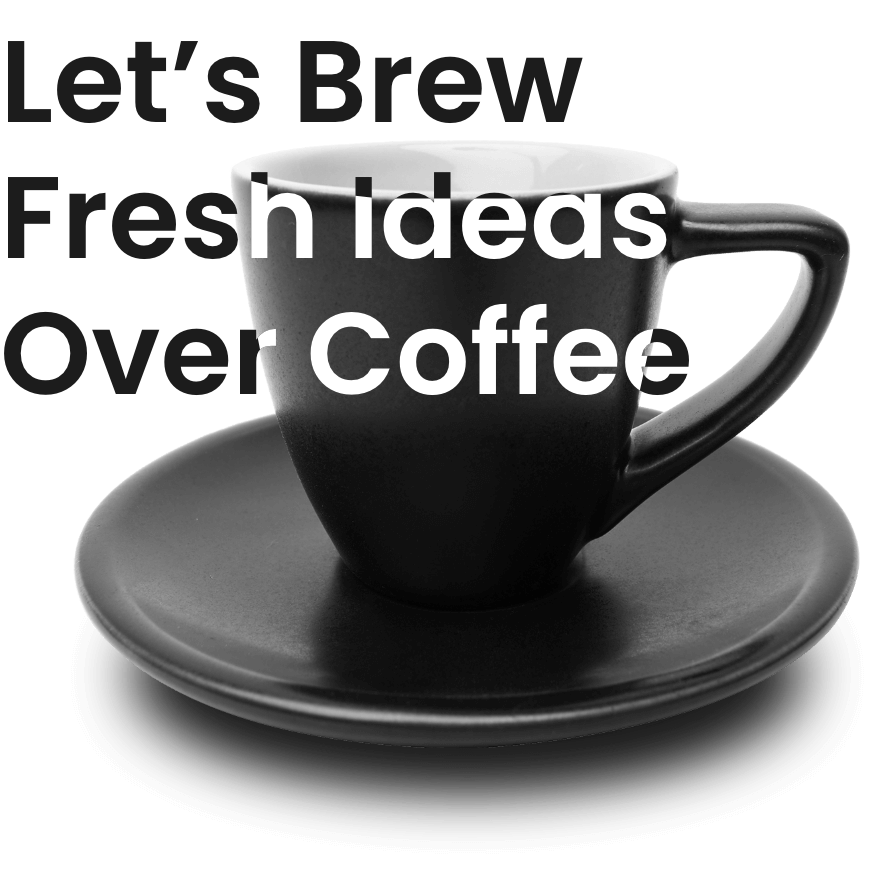
Spanning 8 cities worldwide and with partners in 100 more, we’re your local yet global agency.
Fancy a coffee, virtual or physical? It’s on us – let’s connect!