The role of master data modeling in data analytics for the banking industry
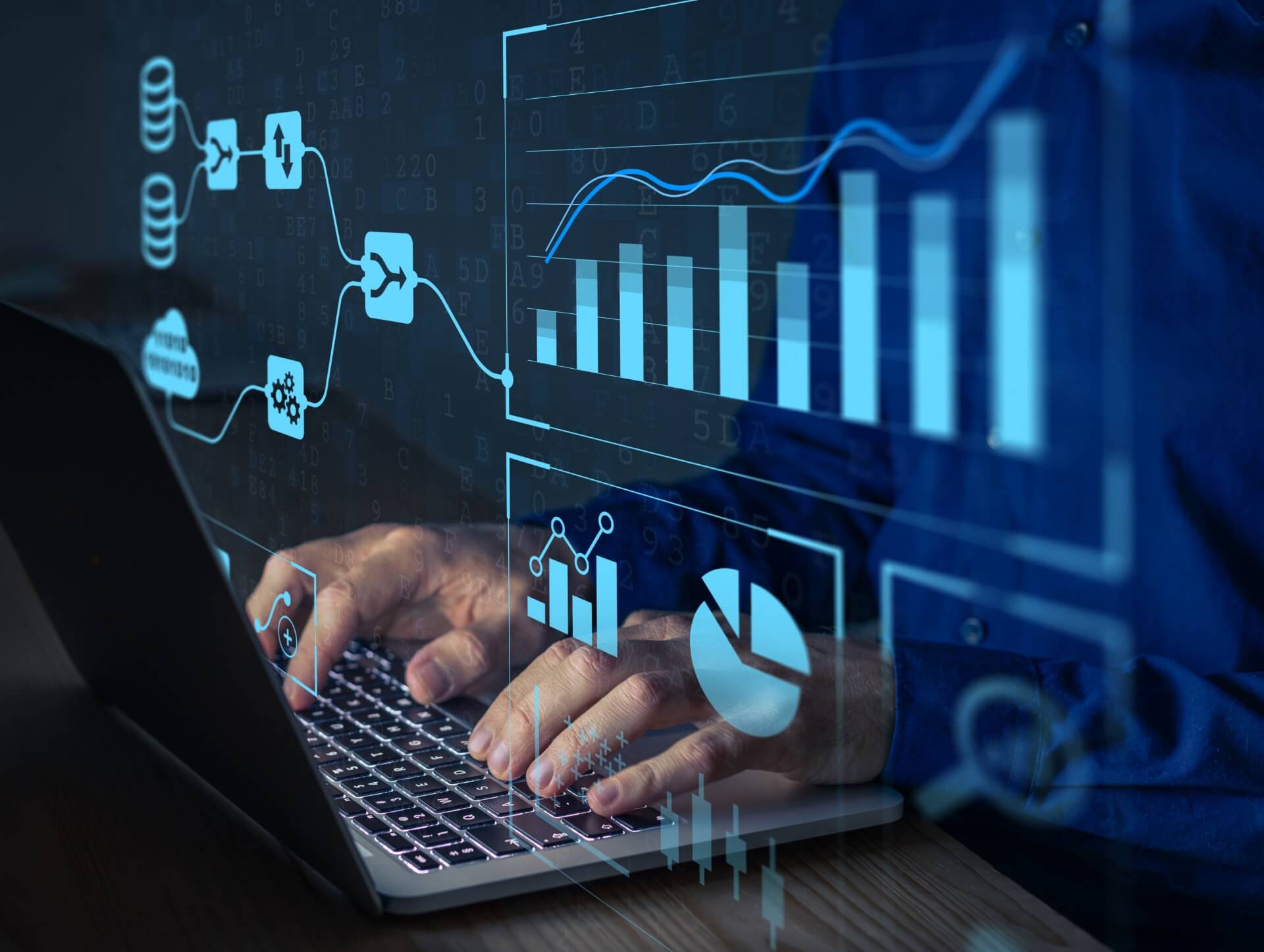
06/09/2023
The banking industry is increasingly relying on data analytics to gain insights, make informed decisions, and enhance customer experience. However, the success of data analytics initiatives depends on the quality and accuracy of the underlying data. This is where master data modeling plays a crucial role. In this article, we will explore the role of master data modeling in data analytics for the banking industry, including its benefits, challenges, and best practices.
What is Master Data Modeling?
Master data modeling is the process of creating a logical representation of the key data entities and their relationships within an organization. It involves identifying the critical data elements, defining their attributes, and establishing the relationships between them. Master data modeling provides a foundation for data governance, data integration, and data analytics.
The Benefits of Master Data Modeling in Data Analytics
Master data modeling brings several benefits to data analytics in the banking industry:
1. Improved Data Quality
By creating a standardized and consistent representation of master data, master data modeling helps in improving data quality. It ensures that the data used for analytics is accurate, complete, and reliable. This, in turn, leads to more accurate and reliable insights.
2. Enhanced Data Integration
Master data modeling facilitates data integration by providing a common understanding of data elements and their relationships. It helps in aligning data from different sources and systems, enabling a more comprehensive view of customer information, transactions, and other relevant data. This integrated data is essential for effective data analytics.
3. Streamlined Data Governance
Master data modeling establishes a foundation for data governance by defining the structure, rules, and policies for managing master data. It enables organizations to establish data stewardship roles, enforce data quality standards, and ensure compliance with regulatory requirements. Data governance is crucial for maintaining data accuracy, consistency, and security.
4. Improved Decision-Making
Master data modeling provides a holistic view of customer data, product data, and other critical data elements. This comprehensive view enables better decision-making by providing insights into customer behavior, product performance, and market trends. It helps banks identify new opportunities, optimize processes, and mitigate risks.
Challenges in Master Data Modeling for Data Analytics
While master data modeling brings significant benefits, it also comes with its own set of challenges:
1. Data Complexity
The banking industry deals with vast amounts of complex data, including customer data, transaction data, and market data. Master data modeling requires understanding and capturing the relationships between these different data elements, which can be challenging due to their complexity.
2. Data Governance
Effective master data modeling requires strong data governance practices. Establishing data governance processes, defining data stewardship roles, and enforcing data quality standards can be complex and time-consuming. It requires a collaborative effort from different stakeholders across the organization.
3. Data Integration
Data integration is a critical aspect of master data modeling. Banks often have multiple systems and databases that store different aspects of customer information. Integrating these disparate data sources and ensuring data consistency can be challenging.
4. Data Security
Master data modeling involves working with sensitive and confidential data, including customer information and financial transactions. Ensuring data security and protecting against unauthorized access is of utmost importance. Banks must implement robust security measures to safeguard data throughout the data analytics process.
Best Practices for Master Data Modeling in Data Analytics
To overcome the challenges and maximize the benefits of master data modeling in data analytics, banks should follow these best practices:
1. Clearly Define Data Entities and Attributes
Clearly define the key data entities and their attributes based on the specific requirements of the banking industry. This includes customer data, product data, transaction data, and other relevant data elements. Ensure that the definitions are consistent and aligned with industry standards.
2. Establish Data Governance Processes
Establish robust data governance processes to ensure data accuracy, consistency, and security. Define data stewardship roles and responsibilities, and implement data quality standards and policies. Regularly monitor and measure data quality to identify and address any issues.
3. Implement Data Integration Strategies
Implement data integration strategies to bring together data from different sources and systems. Use data integration tools and technologies to streamline the process and ensure data consistency. Establish data integration workflows and processes to automate the integration process.
4. Prioritize Data Security
Implement strong data security measures to protect sensitive and confidential data. This includes encrypting data, implementing access controls, and regularly monitoring for any security breaches. Conduct regular security audits and assessments to identify and address any vulnerabilities.
Case Studies: The Role of Master Data Modeling in Data Analytics
Several banks have successfully implemented master data modeling in their data analytics initiatives:
1. Bank XYZ
Bank XYZ implemented a master data management solution to create a unified view of customer data across its various systems and channels. This enabled them to gain insights into customer behavior, preferences, and profitability. They were able to personalize their marketing campaigns, optimize cross-selling opportunities, and improve customer satisfaction.
2. Bank ABC
Bank ABC implemented a master data management platform to streamline their data integration processes. They were able to integrate customer data from multiple sources, including online banking, mobile banking, and branch interactions. This allowed them to provide a seamless and personalized customer experience across different channels.
The Future of Master Data Modeling in Data Analytics for the Banking Industry
The role of master data modeling in data analytics for the banking industry will continue to grow in importance. As banks embrace digital transformation and leverage advanced analytics techniques, the need for accurate and reliable data becomes even more critical. Master data modeling will play a significant role in ensuring data quality, integration, and governance.
Furthermore, the increasing adoption of cloud-based master data management solutions and multi-cloud environments will present new challenges and opportunities for master data modeling. Banks will need to adapt their master data modeling strategies to effectively manage and analyze data across different cloud platforms.
Conclusion
Master data modeling is a crucial component of data analytics for the banking industry. It enables banks to improve data quality, enhance data integration, streamline data governance, and make better-informed decisions. Despite the challenges, following best practices and leveraging master data modeling can help banks unlock the full potential of their data analytics initiatives and gain a competitive advantage in the market.
Contact us
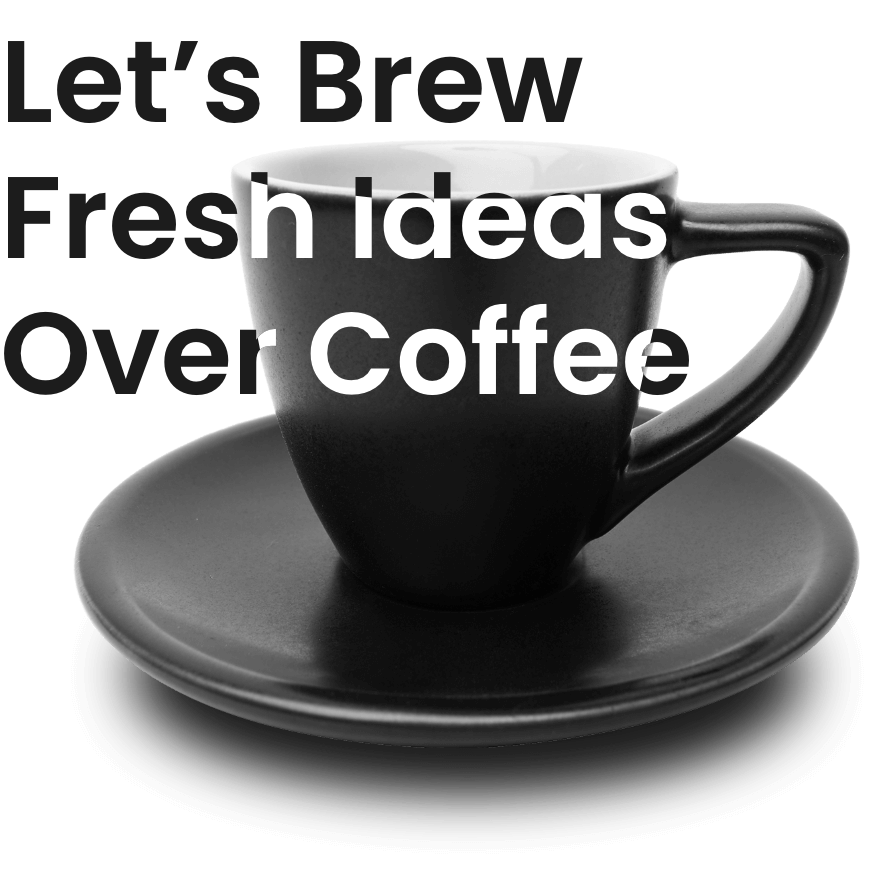
Spanning 8 cities worldwide and with partners in 100 more, we’re your local yet global agency.
Fancy a coffee, virtual or physical? It’s on us – let’s connect!