The role of master data modeling in data governance
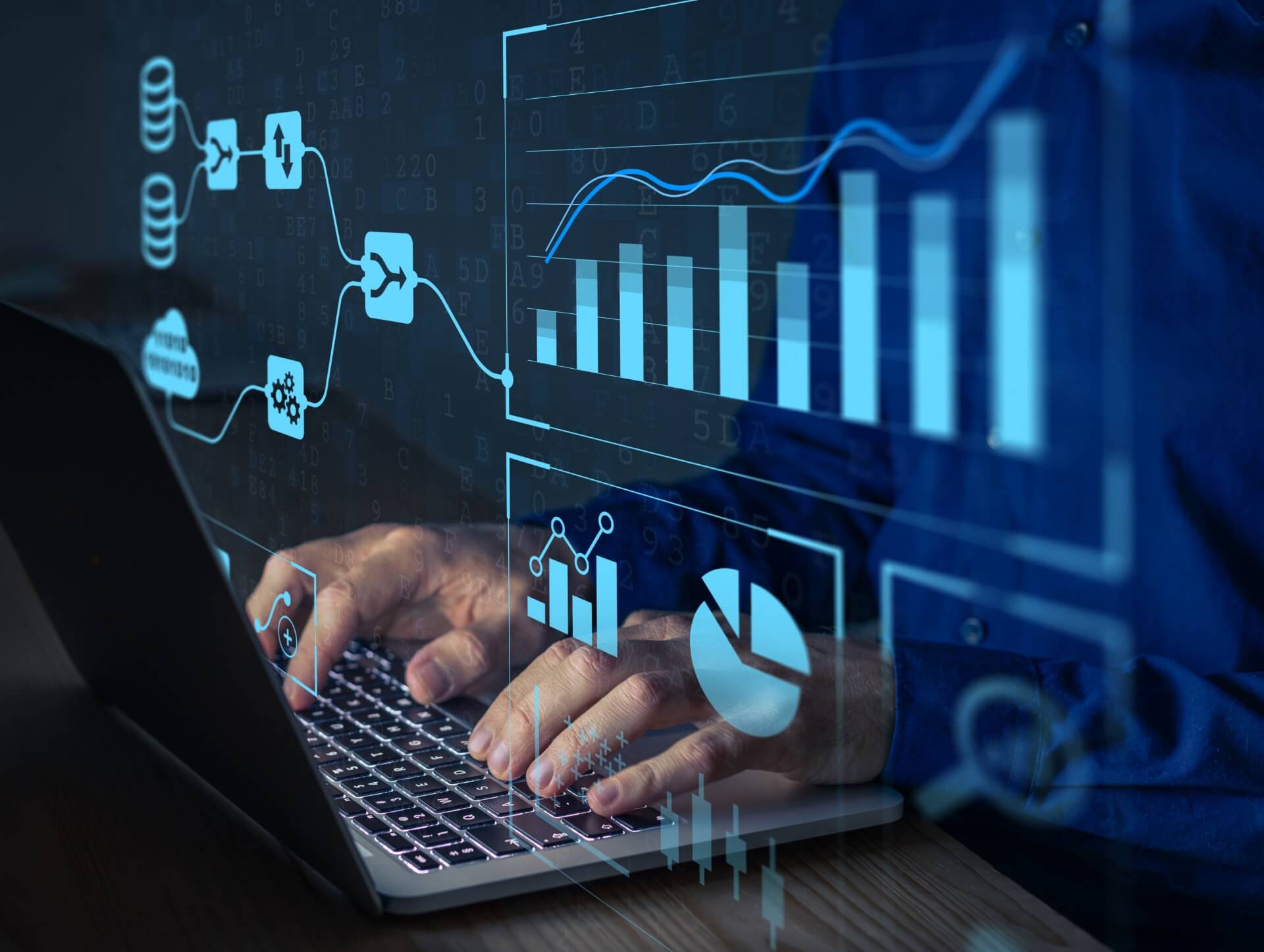
06/09/2023
Master data management (MDM) is an essential practice for organizations to establish a single, accurate, and consistent version of their critical data. It involves creating and maintaining a central repository of master data that serves as a trusted source for various business processes and applications. To effectively implement and govern MDM, master data modeling plays a crucial role. In this article, we will explore the importance of master data modeling in data governance and its impact on businesses.
What is Master Data Modeling?
Master data modeling is the process of designing the structure and relationships of master data entities within an organization. It involves defining the attributes, hierarchies, and relationships of entities such as customers, products, locations, and suppliers. The goal of master data modeling is to create a logical representation of master data that aligns with the business requirements and ensures data consistency, accuracy, and integrity.
The Role of Master Data Modeling in Data Governance
Data governance in MDM refers to the set of processes, policies, and standards that govern the management and usage of master data. Master data modeling plays a pivotal role in establishing a solid foundation for data governance. Here are some key aspects of how master data modeling contributes to data governance:
1. Data Quality Management
Master data modeling helps organizations define data quality rules and standards. By modeling the attributes and relationships of master data entities, organizations can enforce data validation rules, ensure data completeness, and improve data accuracy. This ensures that the master data meets the required quality standards and can be relied upon for critical business decisions.
2. MDM Strategy for Businesses
A well-designed master data model forms the basis of an effective MDM strategy. It enables organizations to identify and prioritize the key master data entities that need to be managed. By understanding the relationships and dependencies between different entities, organizations can define the scope and objectives of their MDM initiatives and align them with their business goals.
3. MDM Implementation Process
Master data modeling plays a crucial role in the implementation process of MDM solutions. It provides a blueprint for how the master data should be structured, stored, and integrated with various systems and applications. This helps organizations streamline the implementation process and ensures that the MDM solution meets the specific requirements of the organization.
4. Data Integration in MDM
Master data modeling enables organizations to define the integration points and data flows between different systems and applications. It helps identify the data sources, data mappings, and transformations required to integrate the master data with other systems. This ensures data consistency and enables organizations to have a unified view of their master data across the enterprise.
Benefits of Master Data Modeling in Data Governance
Implementing master data modeling as part of data governance practices brings several benefits to organizations. Here are some key advantages:
1. Improved Data Consistency and Accuracy
Master data modeling ensures that the master data is consistently structured and accurately represented. This helps organizations eliminate duplicates, inconsistencies, and errors in their master data. With a well-defined master data model, organizations can establish data standards and enforce them across the enterprise, resulting in improved data quality.
2. Enhanced Data Accessibility and Usability
By modeling the relationships and dependencies between master data entities, organizations can improve data accessibility and usability. A well-designed master data model provides a clear understanding of how different master data entities are connected, making it easier for users to navigate and retrieve the required data. This enhances data usability and enables users to make informed decisions based on reliable and up-to-date information.
3. Streamlined Business Processes
Master data modeling helps organizations streamline their business processes by providing a standardized and consistent view of master data. With a well-defined master data model, organizations can automate data validation, data enrichment, and data integration processes, reducing manual effort and improving operational efficiency. This enables organizations to accelerate their business processes and respond quickly to market changes.
4. Improved Data Governance and Compliance
Master data modeling is a key enabler for effective data governance and compliance. By defining the structure and relationships of master data entities, organizations can establish data governance policies, data ownership, and data stewardship roles. This ensures that the master data is governed and managed according to the defined standards and regulatory requirements, reducing the risk of data breaches and non-compliance.
Challenges in Master Data Modeling
While master data modeling brings significant benefits to organizations, it also comes with its own set of challenges. Here are some common challenges faced in master data modeling:
1. Complexity of Data Relationships
In complex organizations, the relationships between master data entities can be intricate and challenging to model. Organizations often struggle to capture and represent the complex relationships accurately, leading to inconsistencies and inaccuracies in the master data model. It requires a deep understanding of the business processes and data dependencies to overcome this challenge.
2. Data Integration and Interoperability
Integrating master data with various systems and applications can be a complex task. Organizations need to ensure seamless data integration and interoperability while maintaining data consistency and integrity. This requires careful planning, mapping, and transformation of data to ensure that it aligns with the requirements of different systems and applications.
3. Data Security and Privacy
Master data modeling involves handling sensitive and confidential data, such as customer information and financial data. Organizations need to ensure data security and privacy throughout the master data lifecycle. This includes implementing access controls, data encryption, and data masking techniques to protect the master data from unauthorized access and data breaches.
4. Change Management and Adoption
Implementing a new master data model requires change management and user adoption. Organizations need to communicate the benefits and impact of the new master data model to the stakeholders and ensure their buy-in. They also need to provide training and support to users to help them understand and adapt to the new model effectively.
Best Practices for Master Data Modeling
To overcome the challenges and ensure the success of master data modeling, organizations should follow these best practices:
1. Understand the Business Requirements
Before designing the master data model, organizations should have a clear understanding of their business requirements and objectives. This includes identifying the key master data entities, their attributes, and the relationships between them. By aligning the master data model with the business requirements, organizations can ensure that it meets the specific needs of the organization.
2. Involve Stakeholders and Subject Matter Experts
Master data modeling is a collaborative process that requires input from various stakeholders and subject matter experts. Organizations should involve business users, data stewards, IT teams, and other relevant stakeholders in the design and validation of the master data model. This ensures that the model reflects the diverse perspectives and requirements of the organization.
3. Follow Standard Data Modeling Techniques
Organizations should follow standard data modeling techniques and best practices while designing the master data model. This includes using entity-relationship diagrams, defining data types and constraints, and ensuring data normalization. By adhering to standard data modeling techniques, organizations can create a robust and scalable master data model.
4. Ensure Data Governance and Data Stewardship
Effective data governance and data stewardship are essential for maintaining the integrity and quality of the master data model. Organizations should establish data governance policies, data ownership, and data stewardship roles to ensure that the master data is governed and managed effectively. This includes defining data validation rules, data cleansing processes, and data maintenance procedures.
Conclusion
Master data modeling plays a critical role in data governance and is essential for organizations to establish a reliable and accurate source of master data. It enables organizations to improve data quality, streamline business processes, and ensure data governance and compliance. By following best practices and overcoming the challenges, organizations can leverage master data modeling to derive valuable insights, make informed decisions, and drive business growth.
Contact us
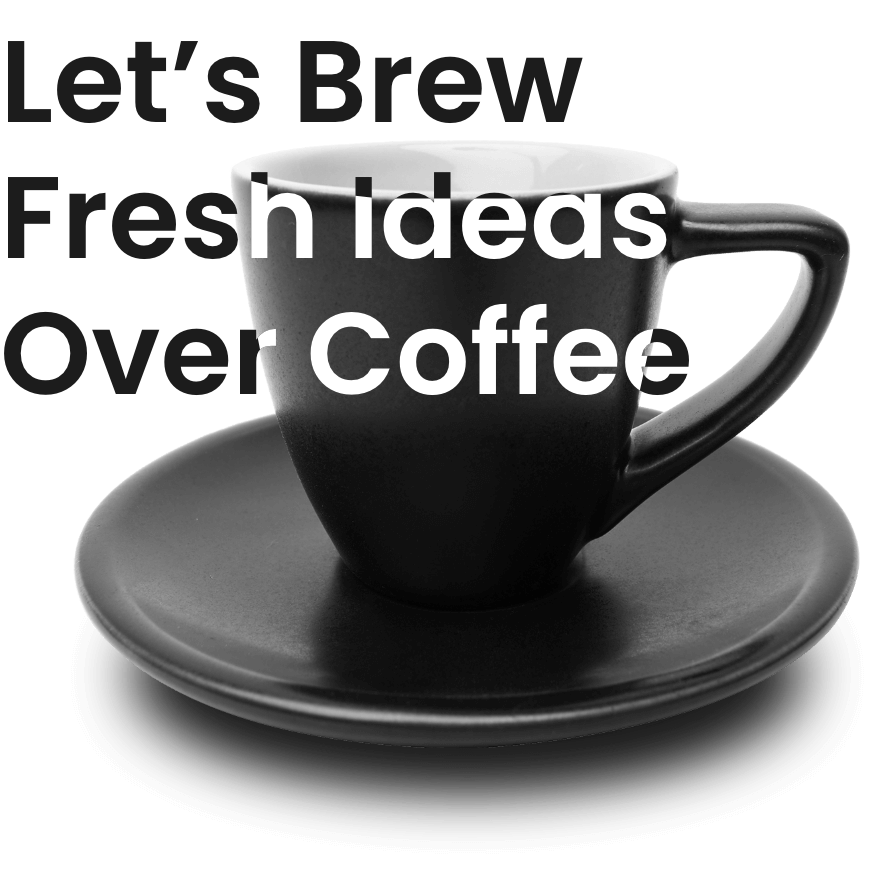
Spanning 8 cities worldwide and with partners in 100 more, we’re your local yet global agency.
Fancy a coffee, virtual or physical? It’s on us – let’s connect!