The role of master data modeling in data virtualization
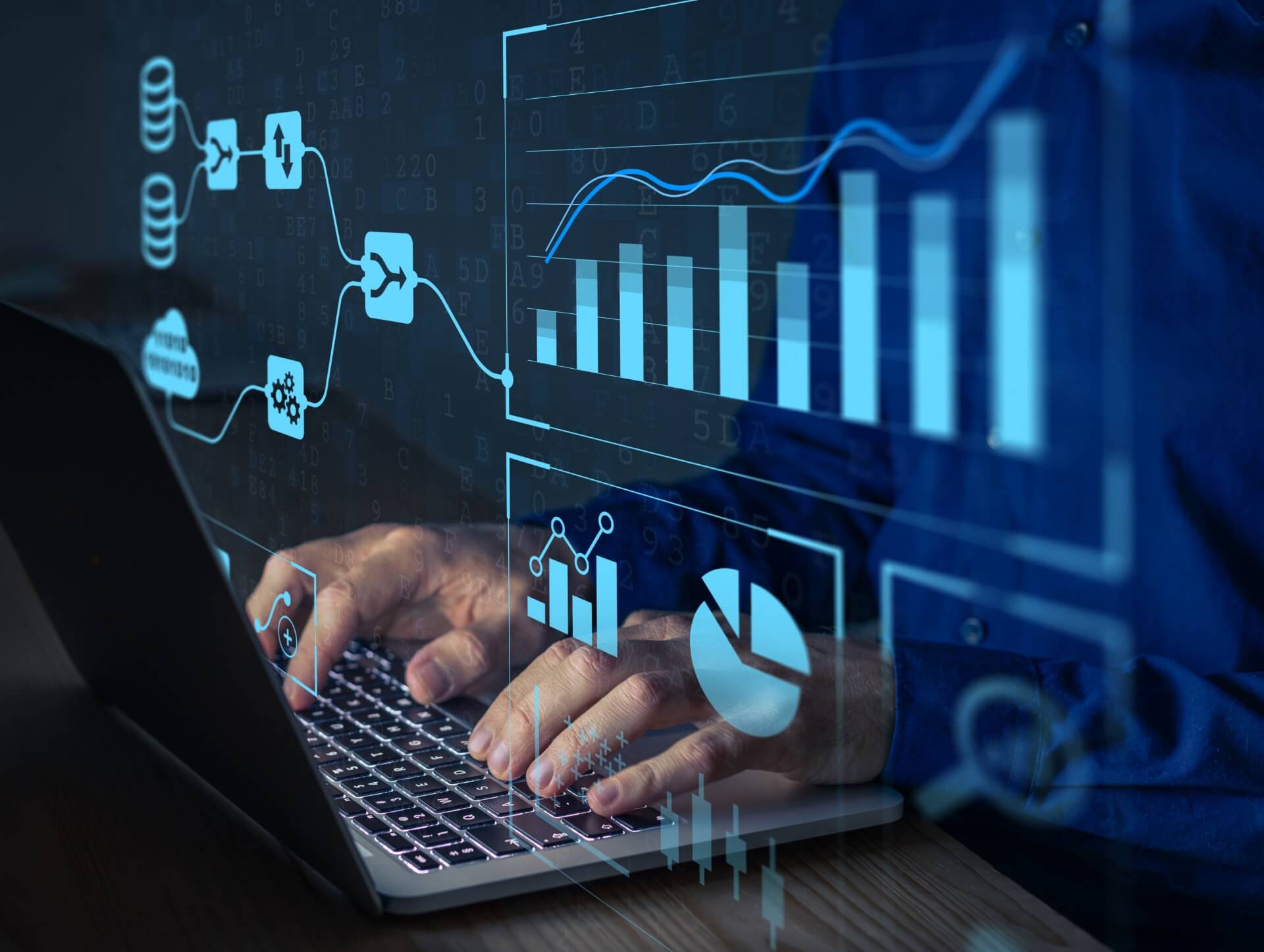
06/09/2023
Master Data Management (MDM) is a crucial process for businesses that aims to establish a single, trusted source of data across various systems and applications. It involves defining and managing critical data entities such as customers, products, and suppliers to ensure data consistency, accuracy, and integrity throughout the organization.
One of the key components of MDM is master data modeling, which plays a vital role in data virtualization. In this article, we will explore the significance of master data modeling in data virtualization, its benefits in master data management, and how it contributes to improving data governance, data security, and data analytics.
The Role of Master Data Modeling in Data Virtualization
Data virtualization is the process of abstracting, integrating, and delivering data from various sources in real-time, without physically moving or replicating the data. It allows organizations to access and analyze data from multiple systems and applications as if it were stored in a single location.
Master data modeling plays a crucial role in data virtualization by providing a unified and standardized representation of the master data entities. It involves designing a logical data model that defines the structure, relationships, and attributes of the master data entities, such as customers, products, and suppliers.
By modeling the master data entities, organizations can ensure consistency and accuracy in data integration and virtualization processes. The master data model acts as a blueprint for integrating and mapping data from various sources, enabling organizations to create a virtualized view of the data that can be accessed and analyzed in real-time.
Furthermore, master data modeling enables organizations to define data validation rules, data quality standards, and data transformation logic, which are essential for ensuring the accuracy, completeness, and integrity of the master data in the virtualized environment.
Benefits of Master Data Modeling in Master Data Management
Master data modeling offers several benefits in the context of master data management:
- Improved Data Governance: Master data modeling provides a foundation for implementing effective data governance practices. It helps organizations establish data ownership, define data stewardship roles and responsibilities, and enforce data quality standards and policies.
- Enhanced Data Security: By modeling the master data entities and defining data security rules, organizations can ensure that sensitive data is protected throughout the data virtualization process. Access controls, encryption, and other security measures can be implemented based on the master data model.
- Better Data Analytics: Master data modeling enables organizations to create a unified view of the master data, which can be leveraged for advanced analytics and reporting. By integrating and virtualizing data from various sources, organizations can gain valuable insights and make data-driven decisions.
- Streamlined Data Integration: Master data modeling simplifies the process of integrating and mapping data from disparate sources. It provides a common framework for data integration and ensures consistency and accuracy in data transformation and mapping.
Master Data Modeling and Data Governance
Data governance is an essential component of MDM, as it ensures that data is managed effectively throughout its lifecycle. Master data modeling plays a crucial role in establishing and implementing data governance practices:
1. Data Ownership and Stewardship: The master data model defines the ownership of each data entity and assigns data stewardship responsibilities. Data stewards are responsible for ensuring data quality, resolving data issues, and enforcing data governance policies.
2. Data Quality Management: The master data model includes data quality rules and standards, which are used to assess and measure data quality. By defining data quality metrics and thresholds, organizations can monitor and improve the quality of the master data.
3. Data Security and Privacy: Master data modeling enables organizations to define data security and privacy rules based on the sensitivity and classification of the data. Access controls, encryption, and other security measures can be implemented to protect the master data from unauthorized access and breaches.
4. Data Compliance: The master data model helps organizations comply with data regulations and industry standards. By incorporating compliance requirements into the master data model, organizations can ensure that data is managed in accordance with legal and regulatory guidelines.
Master Data Modeling and Data Security
Data security is a critical aspect of MDM, as it involves managing and protecting sensitive data from unauthorized access, breaches, and theft. Master data modeling contributes to data security in the following ways:
1. Data Classification and Sensitivity: The master data model enables organizations to classify and categorize data based on its sensitivity. By defining data classification levels, organizations can apply appropriate security controls and measures to protect sensitive data.
2. Access Controls: Master data modeling allows organizations to define access controls and permissions based on the roles and responsibilities of users. Role-based access controls ensure that only authorized users have access to the master data, reducing the risk of unauthorized data access.
3. Data Encryption: The master data model can include encryption requirements for sensitive data. Encryption ensures that data is protected even if it is intercepted or accessed by unauthorized individuals or systems.
4. Data Masking and Anonymization: Master data modeling enables organizations to define data masking and anonymization techniques for sensitive data. By masking or anonymizing data, organizations can protect the privacy of individuals and comply with data privacy regulations.
Master Data Modeling and Data Analytics
Data analytics is a key driver of business success, as it enables organizations to gain insights, make informed decisions, and drive innovation. Master data modeling contributes to data analytics in the following ways:
1. Unified View of Master Data: By creating a unified and standardized representation of the master data, organizations can analyze and gain insights from a comprehensive view of the data. The master data model acts as a foundation for data integration and virtualization, enabling organizations to create a single source of truth for analytics.
2. Data Integration and Transformation: Master data modeling simplifies the process of integrating and transforming data from various sources. By mapping data to the master data model, organizations can ensure consistency and accuracy in data integration, enabling more reliable and accurate analytics.
3. Data Aggregation and Roll-up: The master data model allows organizations to aggregate and roll-up data to higher levels of granularity. This enables organizations to analyze data at different levels of detail and make meaningful comparisons and summaries.
4. Advanced Analytics and Machine Learning: Master data modeling provides a foundation for advanced analytics and machine learning. By integrating and virtualizing data from various sources, organizations can leverage advanced analytics techniques to uncover hidden patterns, predict outcomes, and drive data-driven decision-making.
Challenges in Master Data Modeling
Despite its benefits, master data modeling also presents challenges that organizations need to overcome:
- Data Complexity: Master data modeling can be complex due to the variety of data entities, relationships, and attributes involved. Organizations need to invest time and effort in understanding the data landscape and designing a comprehensive master data model.
- Data Integration: Integrating data from various sources can be challenging, as it requires aligning data structures, resolving data conflicts, and ensuring data consistency. Master data modeling helps streamline the data integration process, but organizations still need to overcome technical and operational challenges.
- Data Quality: Maintaining data quality is a continuous challenge in master data management. Organizations need to define data quality rules, monitor data quality metrics, and implement data cleansing and enrichment processes to ensure the accuracy and integrity of the master data.
- Data Governance: Implementing effective data governance practices requires organizational commitment, stakeholder buy-in, and clear communication. Master data modeling provides a foundation for data governance, but organizations need to establish governance processes and enforce data governance policies.
Conclusion
Master data modeling plays a crucial role in data virtualization and master data management. By creating a unified and standardized representation of the master data entities, organizations can improve data governance, data security, and data analytics. Master data modeling provides a foundation for effective data integration, transformation, and virtualization, enabling organizations to access and analyze data from multiple sources in real-time. Despite the challenges involved, organizations can leverage master data modeling to establish a single source of truth for their critical data entities and drive data-driven decision-making.
Contact us
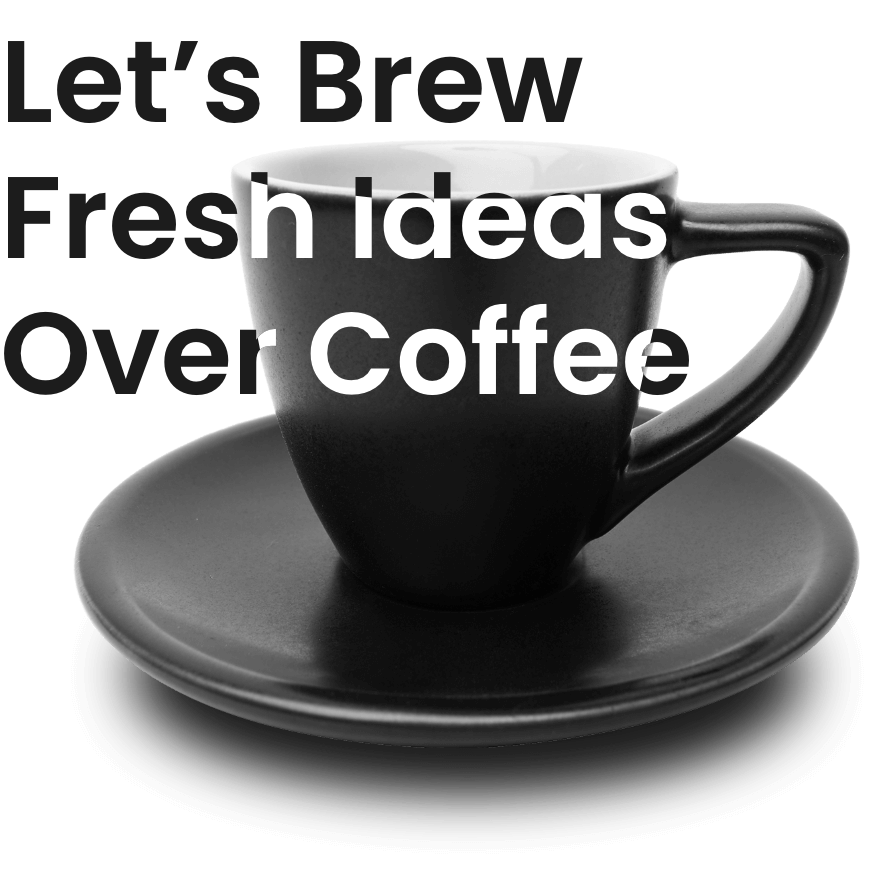
Spanning 8 cities worldwide and with partners in 100 more, we’re your local yet global agency.
Fancy a coffee, virtual or physical? It’s on us – let’s connect!