The role of master data modeling in financial services
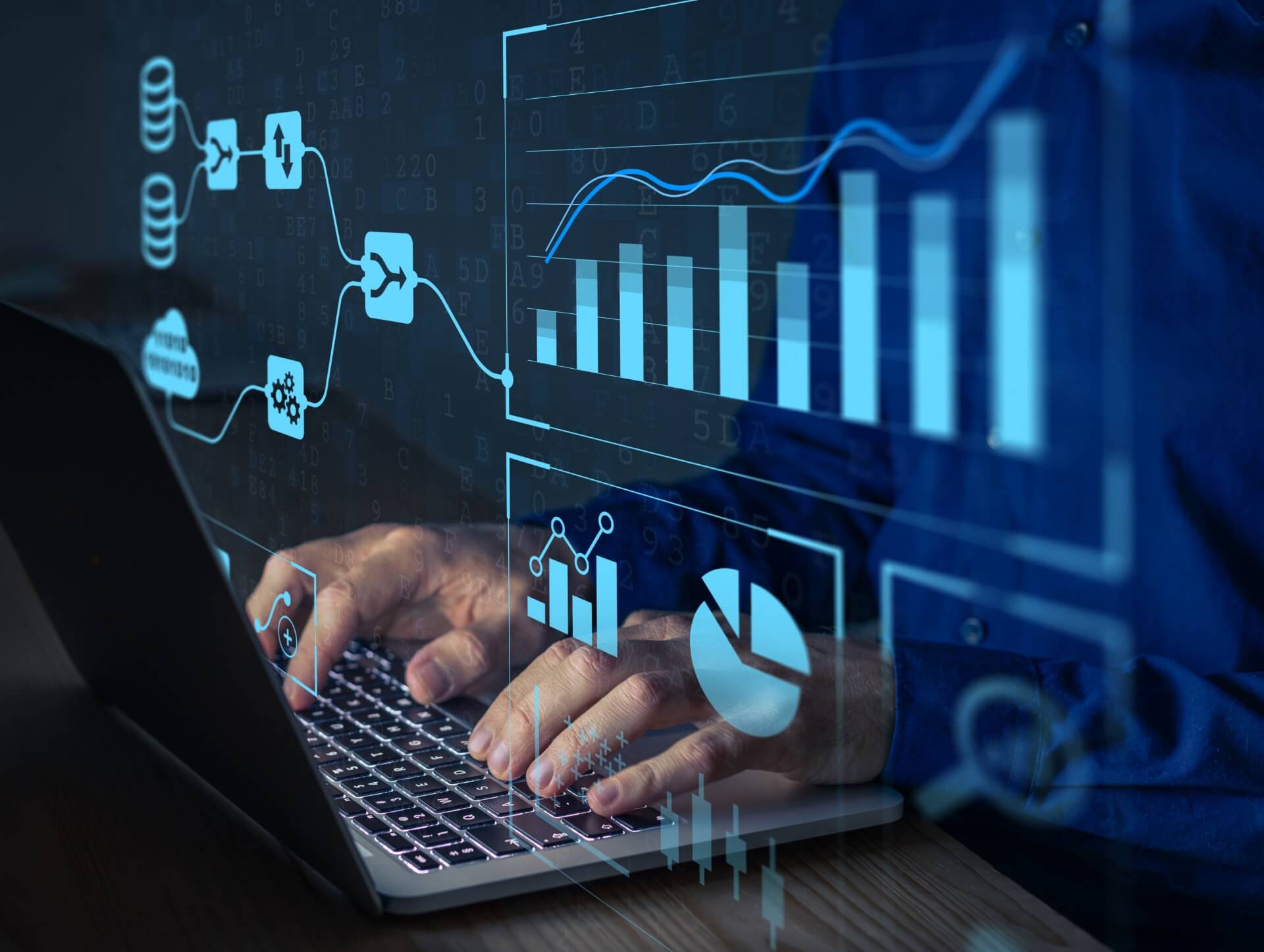
06/09/2023
Master data modeling plays a crucial role in the financial services industry, enabling organizations to effectively manage their data assets and drive business growth. In this article, we will explore the concept of master data modeling, its benefits, and its impact on various aspects of financial services. We will also discuss best practices for implementing master data modeling and overcoming challenges in the process.
What is Master Data Modeling?
Master data modeling refers to the process of designing and creating a logical representation of an organization's key data elements, known as master data. Master data includes critical information about customers, products, employees, suppliers, and other entities that are essential for day-to-day operations and decision-making.
Master data modeling involves defining the structure, relationships, and attributes of master data entities and creating a standardized format for storing and managing the data. It helps ensure data consistency, accuracy, and integrity across different systems and applications within an organization.
The Benefits of Master Data Modeling
Implementing a master data modeling strategy brings several benefits to financial services organizations:
1. Improved Data Quality and Integrity
Master data modeling enables organizations to establish data governance frameworks and implement data quality management practices. By defining data standards, validation rules, and data cleansing processes, organizations can ensure the accuracy and consistency of their master data. This leads to improved decision-making and better customer experiences.
2. Enhanced Operational Efficiency
By having a well-defined master data model, financial services organizations can streamline data integration processes and eliminate redundant or duplicate data. This results in improved operational efficiency and reduced costs associated with data management.
3. Better Customer Experience
Master data modeling allows organizations to gain a holistic view of their customers by consolidating customer data from various sources and systems. This enables personalized and targeted marketing campaigns, improved customer service, and better customer relationship management.
4. Regulatory Compliance
In the highly regulated financial services industry, compliance with data protection and privacy regulations is of utmost importance. Master data modeling helps organizations ensure compliance by implementing data governance frameworks, data security measures, and data lifecycle management practices.
Implementing Master Data Modeling in Financial Services
Implementing a master data modeling strategy requires careful planning and consideration of various factors. Here are some best practices to follow:
1. Define Clear Objectives and Scope
Before embarking on a master data modeling initiative, organizations should clearly define their objectives and scope. This involves identifying the key master data entities to be modeled, understanding the business processes that rely on this data, and determining the desired outcomes of the project.
2. Establish Data Governance Frameworks
Data governance is crucial for the success of master data modeling. Organizations should establish data governance frameworks that define roles, responsibilities, and processes for managing master data. This includes creating data stewardship roles, implementing data quality management practices, and establishing data governance committees.
3. Choose the Right Tools and Technologies
There are several master data management (MDM) tools and technologies available in the market. Organizations should carefully evaluate their requirements and choose a suitable MDM solution that aligns with their business goals and IT infrastructure. This may include considering factors such as scalability, integration capabilities, data security features, and ease of use.
4. Ensure Data Integration and Interoperability
Master data modeling involves integrating master data from various sources and systems. Organizations should ensure that the chosen MDM solution supports data integration and interoperability with existing systems and applications. This includes data mapping, data cleansing, and data transformation processes.
5. Implement Data Security Measures
Data security is a critical aspect of master data modeling. Financial services organizations deal with sensitive customer information and need to ensure its confidentiality, integrity, and availability. Implementing data security measures such as access controls, encryption, and data masking is essential to protect master data from unauthorized access and breaches.
Challenges in Master Data Modeling
Implementing master data modeling in financial services can be challenging due to various reasons:
1. Data Complexity and Volume
The financial services industry deals with vast amounts of data from multiple sources, making data modeling complex. Organizations need to handle structured and unstructured data, integrate data from various systems, and ensure data quality and consistency.
2. Data Governance and Data Stewardship
Establishing effective data governance frameworks and data stewardship roles can be challenging. Organizations need to define clear data ownership, establish data quality management processes, and ensure compliance with data protection regulations.
3. Data Integration and Interoperability
Integrating master data from different systems and ensuring interoperability can be complex. Organizations need to map data attributes, transform data formats, and establish data synchronization processes to maintain data consistency across systems.
4. Data Security and Privacy
Data security and privacy are critical concerns in the financial services industry. Organizations need to implement robust security measures to protect master data from unauthorized access, breaches, and data loss.
Case Studies: Successful Master Data Modeling Implementations
Several financial services organizations have successfully implemented master data modeling strategies to drive business growth and improve operational efficiency. Here are two case studies:
1. XYZ Bank: Streamlining Customer Data Management
XYZ Bank, a leading global bank, implemented a master data modeling solution to streamline its customer data management processes. By consolidating customer data from various systems and applications, XYZ Bank gained a 360-degree view of its customers. This enabled personalized marketing campaigns, improved customer service, and enhanced cross-selling opportunities.
2. ABC Insurance: Ensuring Data Integrity and Compliance
ABC Insurance, a multinational insurance company, implemented a master data modeling solution to ensure data integrity and compliance with regulatory requirements. By creating a centralized repository for its policyholder data, ABC Insurance improved data accuracy, reduced data duplication, and implemented data governance frameworks to meet data protection regulations.
The Future of Master Data Modeling in Financial Services
The financial services industry is constantly evolving, and so is the role of master data modeling. In the future, we can expect to see the following trends:
1. Integration with Advanced Analytics
Master data modeling will be integrated with advanced analytics technologies, enabling organizations to derive insights and make data-driven decisions. By combining master data with transactional data and external data sources, financial services organizations can gain a competitive edge and identify new business opportunities.
2. Focus on Data Security and Privacy
With the increasing frequency and sophistication of cyber threats, data security and privacy will remain a top priority for financial services organizations. Master data modeling will incorporate enhanced data security measures, including advanced encryption techniques, multi-factor authentication, and secure data sharing protocols.
3. Adoption of Cloud-Based Master Data Management
Financial services organizations will increasingly adopt cloud-based master data management solutions to leverage the scalability, flexibility, and cost-efficiency of cloud computing. Cloud-based MDM platforms offer seamless integration with other cloud-based applications and provide real-time access to master data from anywhere, anytime.
4. Automation in Data Lifecycle Management
Automation will play a significant role in data lifecycle management, including master data modeling. Organizations will leverage technologies such as artificial intelligence and machine learning to automate data cleansing, data transformation, and data governance processes. This will result in improved data quality, reduced manual effort, and faster time-to-insights.
Conclusion
Master data modeling is a critical component of effective data management in the financial services industry. By implementing a well-defined master data modeling strategy, organizations can improve data quality, enhance operational efficiency, and deliver better customer experiences. However, implementing master data modeling comes with its own set of challenges, including data complexity, data governance, data integration, and data security. By following best practices and leveraging the right tools and technologies, financial services organizations can overcome these challenges and unlock the full potential of their data assets.
Contact us
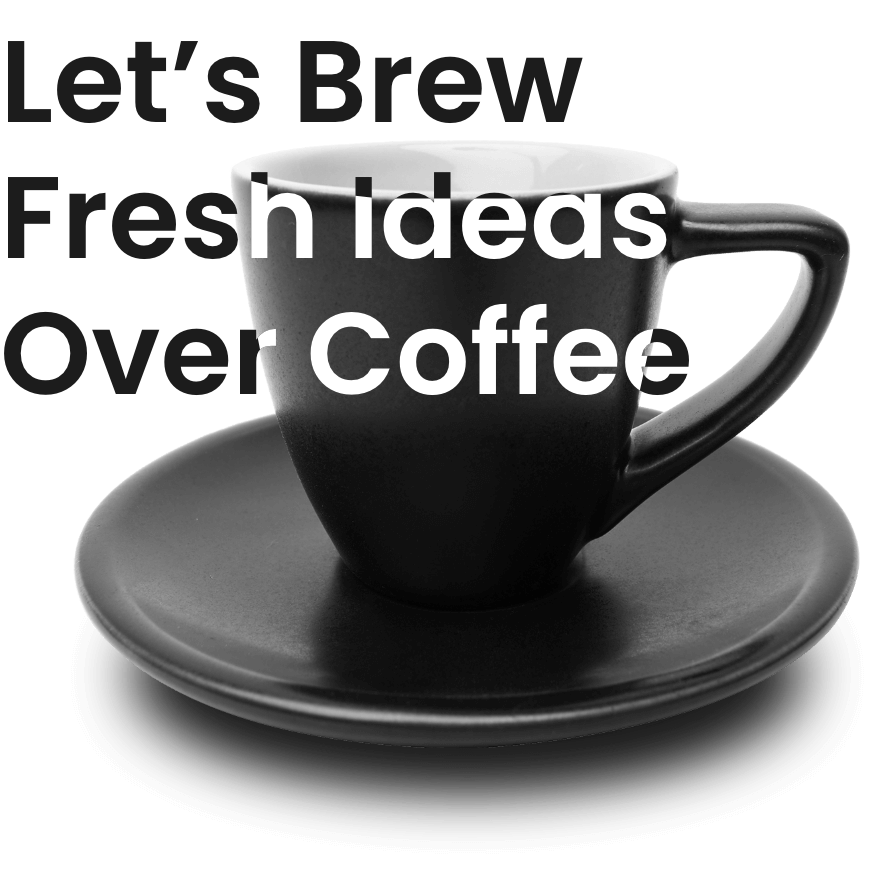
Spanning 8 cities worldwide and with partners in 100 more, we’re your local yet global agency.
Fancy a coffee, virtual or physical? It’s on us – let’s connect!